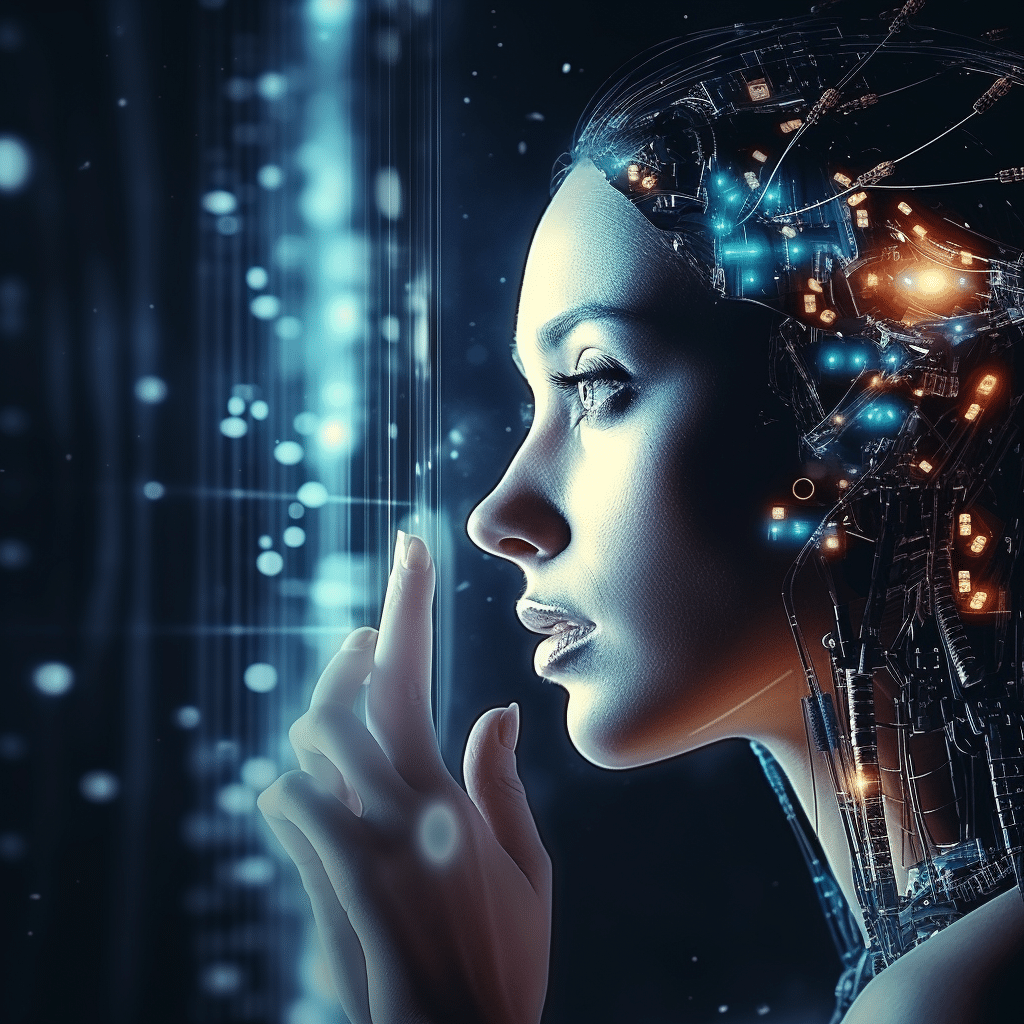
Introduction
Artificial Intelligence (AI) is rapidly becoming an integral part of our lives, impacting industries and making autonomous decisions. However, with the increasing complexity of AI algorithms, it becomes crucial to ensure transparency in AI decision-making processes to maintain fairness, accountability, and user trust.
The Importance of Transparency
Transparency is essential to understand and question the decision-making processes of AI systems. It helps identify biases, prevent unintended consequences, and allows for thorough evaluation and improvement, ensuring fairness and ethical outcomes.
Challenges in AI Decision-Making Transparency
Ensuring transparency in AI decision-making presents several challenges:
- Black Box Algorithms: Many sophisticated AI algorithms work as black boxes, making it difficult to understand the internal processes or reason behind specific decisions.
- Data Bias: Biased training datasets can lead to biased AI decision-making, which can result in unfair and discriminatory outcomes.
- Legal and Ethical Implications: AI decisions must comply with legal and ethical standards. Understanding the underlying decision-making processes helps avoid potential legal and ethical issues.
Strategies to Ensure Transparency
1. Explain ability Techniques
Integrating explainability techniques enables AI systems to provide detailed explanations for their decisions. Techniques such as LIME (Local Interpretable Model-agnostic Explanations) and SHAP (SHapley Additive exPlanations) shed light on the decision’s contributing factors and facilitate understanding.
2. Accessible Documentation
Comprehensive documentation on AI models and their decision-making processes should be provided, ensuring accessibility for both technical experts and non-experts. This empowers users to evaluate the system’s fairness and raises awareness of potential biases and limitations.
3. Auditability and Accountability
Auditability allows for examining the AI decision-making process, tracking inputs, outputs, and model behavior. With clear accountability mechanisms, stakeholders can identify and address potential biases or errors when they occur.
The Future of Transparent AI
As AI technology evolves, the adoption of transparency practices will play a vital role in fostering responsible AI deployment. Industry standards, regulations, and collaborations between AI developers, researchers, and policymakers will contribute to building a transparent and trustworthy AI ecosystem.
“Transparency is the cornerstone of AI ethics, ensuring fairness, accountability, and mitigation of risks.” – AI Ethics Expert
Conclusion
Embracing transparency in AI decision-making processes is crucial to address concerns surrounding biases, accountability, and ethical implications. By implementing explainability techniques, providing accessible documentation, and emphasizing auditability, we can build trust in AI systems and promote their responsible adoption across diverse domains.
What are the risks and challenges of AI algorithm transparency and decision-making?
Maintaining transparency in AI algorithms and decision-making can be challenging due to various risks and obstacles. Some potential risks and challenges include:
1. Intellectual property protection:
Companies may be hesitant to disclose the inner workings of their AI algorithms to protect their intellectual property, competitive advantage, and trade secrets.
2. Bias and fairness:
AI algorithms can learn biases from the data they are trained on, which can lead to biased decision-making. Ensuring fairness and minimizing bias in AI models requires transparency and access to data, which can be challenging in cases where sensitive or proprietary data is involved.
3. Complexity and lack of interpretability:
AI algorithms, particularly deep learning models, can be highly complex and difficult to understand. Lack of interpretability makes it challenging to explain the logic behind AI decisions and identify potential errors or biases.
4. Data privacy and security:
Transparency in AI algorithms may require access to large amounts of data. Ensuring privacy and security while handling sensitive user data can be a significant challenge, especially with increasing regulations like the General Data Protection Regulation (GDPR).
5. Adversarial attacks:
Transparent AI algorithms are susceptible to adversarial attacks, where malicious actors exploit vulnerabilities in the system to manipulate or deceive the AI’s decision-making process.
6. Trade-offs between transparency and performance:
Increasing transparency may sometimes come at the cost of reduced performance or efficiency. Striking a balance between transparency and model performance is necessary to maximize the benefits of AI systems.
7. Regulating black-box systems:
Some AI algorithms, such as deep neural networks, are considered black-box systems due to their lack of interpretability. Regulating and ensuring transparency in such systems can be challenging for policymakers and regulatory bodies.
Addressing these risks and challenges requires a multi-faceted approach that involves collaborations between policymakers, industry experts, and researchers. It involves developing techniques for explainability and interpretability in AI, refining data collection and handling practices, and finding suitable regulatory frameworks to promote transparency while protecting intellectual property and data privacy.
How can enterprises apply AI decision-making transparency rules and practices?
Implementing policies and practices to ensure transparency in AI decision-making processes is important to build trust and accountability. Here are some steps organizations can take:
1. Data transparency:
Ensure there is clarity on what data is being used for training AI models and how it was collected. Make sure data sources are reliable, diverse, and free from biases.
2. Algorithm transparency:
Document and share the algorithms used in AI decision-making processes. This includes the logic, reasoning, and rules followed by the AI system. Avoid using “black-box” models that are difficult to interpret.
3. Explain-ability:
Develop methods to explain AI decisions in a clear and understandable manner. This helps users, customers, and stakeholders understand the reasoning behind AI-based decisions.
4. Regular audits:
Conduct regular audits of AI systems to monitor their performance, identify biases, and ensure compliance with ethical guidelines and regulations.
5. User feedback and redress:
Establish mechanisms for users to provide feedback, question decisions, and seek redress in case of unfair or incorrect AI-based outcomes.
6. Ethical guidelines:
Develop and enforce clear ethical guidelines for AI development and deployment, addressing potential biases, discrimination, and privacy concerns.
7. Cross-functional teams:
Form multidisciplinary teams including AI experts, legal professionals, ethicists, and domain experts to ensure a holistic approach to AI decision-making.
8. Internal and external transparency:
Communicate internally and externally about the organization’s AI practices, including transparency measures, to build trust with stakeholders and the public.
9. Education and training:
Educate employees and end-users about AI systems, their limitations, and the organization’s approach to transparency. Encourage discussions on AI ethics and responsible AI use.
10. Collaboration and industry standards:
Work with other organizations, industry groups, and regulatory bodies to develop common standards and best practices for transparency in AI decision-making.
By following these steps, organizations can promote transparency, foster accountability, and address concerns related to the fairness and ethical use of AI systems.
How can explain ability be used to ensure transparency in AI decision-making?
Explainability plays a crucial role in ensuring transparency in AI decision-making by providing insights into how and why an AI system makes a particular decision. It helps in understanding the underlying factors, logic, and considerations involved in the decision-making process of AI models. Implementing explainability techniques ensures that decisions made by AI systems are not perceived as black boxes but can be comprehended and interpreted by humans.
Effective implementation of explainability in AI decision-making involves several key steps:
1. Model selection:
Choose AI models that inherently provide explainability. For example, rule-based systems or decision trees are inherently explainable, as their decision-making process can be easily traced and understood.
2. Interpretability techniques:
Use interpretability techniques to explain complex AI models, such as deep neural networks. Techniques like feature importance, saliency maps, or attention mechanisms can shed light on which input features or parts of the model contribute most significantly to the final decision.
3. Design for explainability:
Incorporate model-agnostic explainability techniques in the AI system design. Techniques like LIME (Local Interpretable Model-agnostic Explanations) or SHAP (Shapley Additive Explanations) can provide insights into the contributions of different features to the decision-making process.
4. User-friendly explanations:
Present explanations in a user-friendly and understandable format to stakeholders. This involves avoiding technical jargon, using visualization techniques, and providing clear explanations of the decision-making process. User-friendly explanations enhance trust in the AI system and facilitate human oversight.
5. Constant evaluation and improvement:
Regularly evaluate and improve the explainability of AI models. This might involve conducting audits, performing sensitivity analyses, or gathering user feedback. Continuously incorporating feedback and improving the explainability of the system ensures transparency is maintained over time.
6. Compliance with regulations:
Ensure compliance with regulatory requirements regarding transparency and explainability, such as the General Data Protection Regulation (GDPR) in the European Union.
Overall, effective implementation of explainability in AI decision-making fosters transparency, accountability, and trust in AI systems. It enables stakeholders to understand and validate the decisions made by AI models, ultimately promoting responsible and ethical AI adoption.
Your point of view caught my eye and was very interesting. Thanks. I have a question for you.
Very interesting info!Perfect just what I was looking for!Blog monry