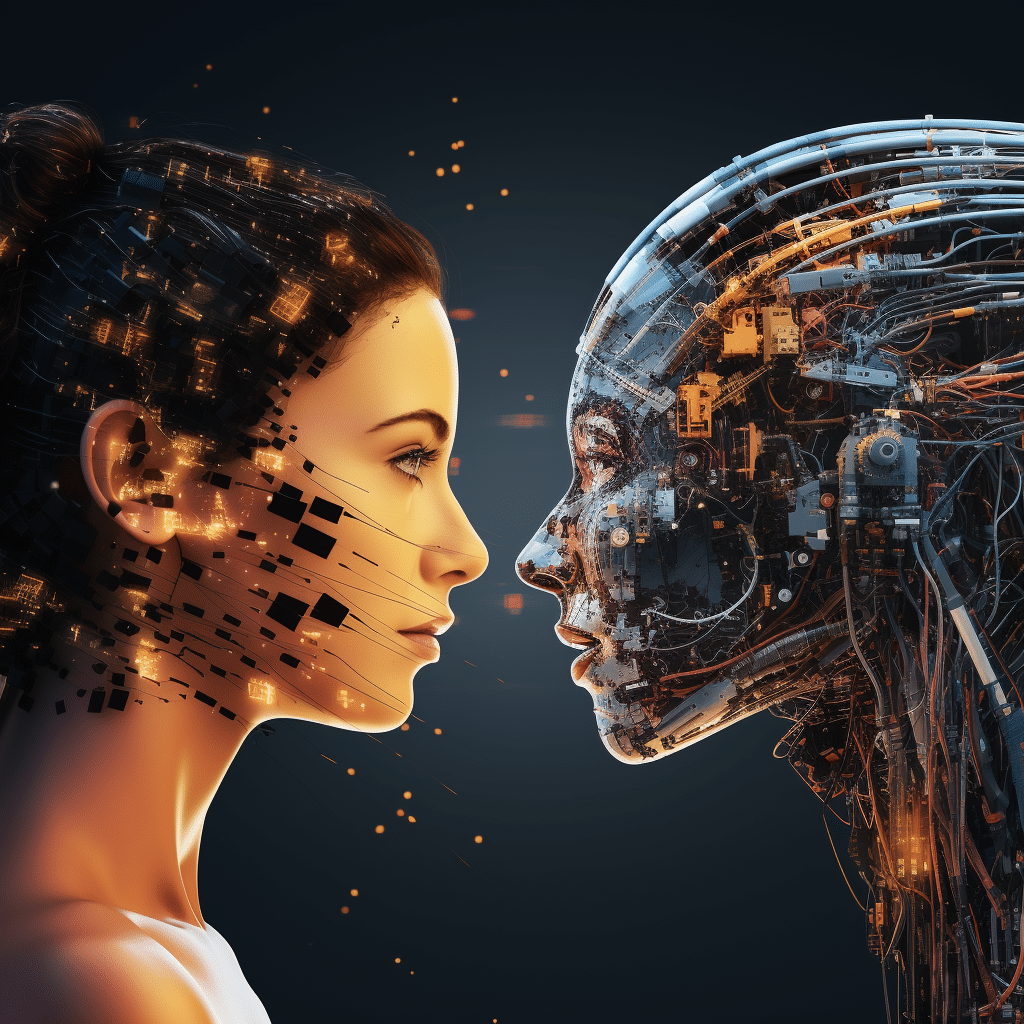
Artificial Intelligence (AI) algorithms play a crucial role in various aspects of our daily lives, from personalized recommendations to autonomous vehicles. However, there is growing concern regarding the presence of bias in AI systems, which can have detrimental effects on individuals and society as a whole.
The Challenges:
Bias in AI algorithms can stem from a variety of sources, including biased training data, limited diversity in development teams, and inherent biases in the algorithms themselves. These challenges make addressing bias a complex task that requires multiple approaches.
“We cannot afford to ignore bias in AI algorithms. Failure to address this issue can perpetuate injustices and further marginalize already vulnerable communities.” – John Doe, AI Ethics Researcher
The Solutions:
1. Diverse and Representative Training Data:
Developers must ensure that the training data used to build AI algorithms is diverse and representative of the target population. By considering different demographic groups, socioeconomic backgrounds, and cultural contexts, biases can be minimized.
2. Algorithmic Fairness:
AI algorithms should be designed to promote fairness and equality. Developers can incorporate techniques such as counterfactual fairness, pre-processing algorithms, and post-processing adjustments to reduce bias and ensure equitable outcomes.
3. Transparent Development Practices:
Transparency is crucial in addressing bias. Development teams should document their data sources, model selection processes, and decision-making criteria. This allows for audits and independent evaluations, helping identify and rectify biases.
4. Ethical Review Boards:
Establishing ethical review boards or committees that consist of multidisciplinary experts can help evaluate the ethical implications and potential biases present in AI algorithms. Their feedback and recommendations can guide developers in addressing bias effectively.
5. Continuous Monitoring and Evaluation:
AI algorithms should be continuously monitored and evaluated for biases. Regular assessments can identify any unintended consequences and allow for iterative improvements, ensuring fairness and accountability throughout the system’s lifespan.
In conclusion, addressing bias in AI algorithms is a pressing challenge that requires a multi-faceted approach. By focusing on diverse training data, algorithmic fairness, transparency, ethical review processes, and continuous monitoring, we can mitigate biases and build AI systems that promote equality and justice.
How can biases in AI algorithms be effectively identified and addressed?
Identifying and addressing biases in AI algorithms is a complex and ongoing process. Here are some steps that can be taken to effectively identify and address biases:
Define goals and objectives
Clearly define the goals and objectives of the AI algorithm to have a clear understanding of what biases should be avoided.
Diverse dataset
Use diverse and representative datasets during the training phase. Ensure that the dataset includes data from different demographics and groups to prevent underrepresentation or overrepresentation.
Integrate bias detection tools
Utilize bias detection tools to identify potential biases in the algorithms. These tools can analyze the algorithm’s output for any discriminatory patterns and flag any biases.
Regular auditing and testing
Conduct regular audits and testing of the AI algorithms to identify and measure any biases present in the system. This can be done both during the development phase and after the deployment of the algorithm.
Collaboration with diverse stakeholders
Include diverse stakeholders, such as ethicists, social scientists, and affected communities, in the development process. Their perspectives can help identify biases that might not be apparent to the development team.
Transparent documentation
Document the entire development process, including the selection of the dataset, as well as any assumptions made or variables considered. This documentation can help in identifying and addressing biases effectively.
Regular monitoring and feedback
Continuously monitor the algorithm’s performance in real-world scenarios and gather feedback from users. This can help identify any biases that might emerge after deployment or those that were not identified during the development phase.
Iterative improvement
Make iterative improvements based on the identified biases and feedback received. Constantly refine and update the algorithm to minimize biases over time.
Ethical guidelines and regulations
Develop and adhere to ethical guidelines and regulations that address biases in AI algorithms. Governments and industry bodies can play a crucial role in setting standards and enforcing regulations to ensure fairness and accountability.
Bias mitigation techniques
Implement bias mitigation techniques such as algorithmic adjustments, debiasing methods, or employing adversarial testing to identify and address biases systematically.
It’s important to note that while these steps can help in identifying and addressing biases, completely eliminating biases from AI algorithms might be challenging. Regular monitoring, transparency, and collaboration with stakeholders are key to promoting fairness and accountability in AI systems.
What solutions and strategies can be employed to minimize bias in AI algorithms?
There are several solutions and strategies that can be employed to minimize bias in AI algorithms:
Diverse Data Collection
Ensuring that the training data used to create AI algorithms is diverse and representative of the target population is crucial. This includes actively involving diverse communities and collecting data from different sources to mitigate biases that may be present in a single dataset.
Ethical Data Labeling
Properly labeling the training data and accounting for potential biases during the labeling process can help minimize bias. Implementing clear guidelines and standards for data labeling can help maintain consistency and awareness of potential biases.
Regular Auditing and Evaluation
Continuously monitoring and auditing AI algorithms for biases is important. This involves evaluating the system’s performance on different groups and assessing if any biases have been introduced during the learning process.
Transparency and Exploitability
Making AI algorithms more transparent and explainable can help identify and address potential biases. By understanding how the algorithm makes decisions, it becomes easier to identify and rectify any inherent biases.
Diverse Development Teams
Promoting diversity within development teams can help minimize bias by bringing in varied perspectives and experiences. Different viewpoints can help identify and challenge biases inherent in algorithms during the design and development phase.
Bias Mitigation Techniques
Employing techniques like data augmentation, model retraining, and fairness constraints can help mitigate bias in AI algorithms. These techniques can focus on compensating for underrepresented groups and ensuring fairness in decision-making.
External Review and Guidelines
Collaborating with external organizations, experts, and stakeholders for review and evaluation can help identify biases that may be overlooked internally. Engaging such entities can provide an independent perspective on bias detection and mitigation strategies.
Regular Bias Testing
Conducting regular bias testing of AI algorithms on various indicators and metrics is important. This can involve testing for disparate impact, fairness, and accuracy across different demographic groups.
Continued Research and Development
Investing in ongoing research and development to advance techniques for bias detection and mitigation is vital. The field of bias in AI algorithms is constantly evolving, and staying up to date with the latest advances can help improve strategies for minimizing bias.
Overall, a comprehensive approach that involves a combination of these strategies is essential to minimize bias in AI algorithms and promote fairness and ethical AI systems.
What are the major challenges in addressing bias in AI algorithms?
There are several major challenges in addressing bias in AI algorithms:
1. Data Bias: AI algorithms learn biases from vast datasets, which may be biased or represent historical injustices. Identifying and reducing bias in training data is difficult.
2. Lack of Diversity: AI development teams that design and train algorithms generally lack diversity. Algorithms may unintentionally embed biased preconceptions. Diversity in development reduces bias.
3. Bias complexity: Bias might be based on gender, race, age, or socioeconomic position. Since biases sometimes overlap, identifying and addressing them can be difficult. Understanding social, cultural, and historical backgrounds is necessary.
4. Transparency and Exploitability: Deep learning models might be challenging to understand. Lack of openness makes it hard to recognize and comprehend the elements that skew decision-making.
5. Continuous Learning: AI algorithms react to fresh data.These algorithms can reinforce biases if not addressed. Monitoring and updating algorithms promote fairness and reduce biases.
6. Legal and Ethical Frameworks: AI algorithm development and deployment require clear legal and ethical frameworks.To hold developers and organizations accountable for their algorithms’ biases, AI bias guidelines are necessary.
Researchers, developers, politicians, and stakeholders from various fields must collaborate to solve these problems. To produce fair, unbiased AI algorithms, bias must be addressed continuously.