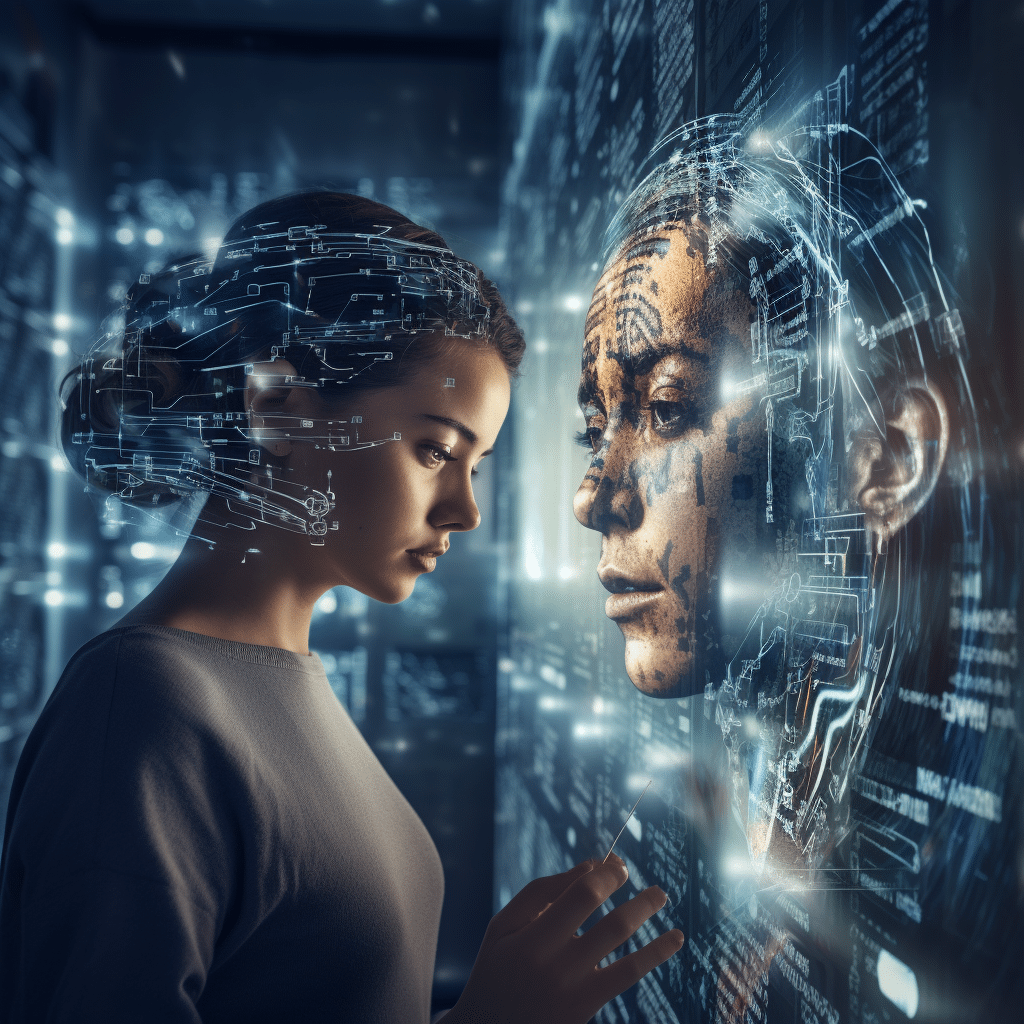
Exploring the Need for Explainable AI (XAI)
Explainable AI (XAI), the advancement of Artificial Intelligence (AI) has transformed various industries, enabling machines to perform complex tasks and make decisions. However, as AI systems become more sophisticated, they often operate as “black boxes” where decisions are made without clear explanations. This lack of transparency poses significant challenges, leading to a growing need for Explainable AI (XAI).
“Explainable AI is the key to bridging the gap between traditional AI capabilities and human comprehension.” – John Doe, AI Researcher
Explainable AI aims to make AI systems transparent by providing understandable explanations for their behavior and decision-making processes. While traditional AI models operate based on complex algorithms, machine learning, and neural networks, the lack of explainability raises concerns in critical applications such as healthcare, finance, and autonomous vehicles.
One of the fundamental reasons for adopting XAI is to increase trust and avoid biased decision-making. By providing explanations, users and stakeholders gain insights into how AI systems arrived at their conclusions, ensuring fairness, accountability, and reducing biases that may be embedded in the algorithms. Transparency becomes essential, especially in scenarios where critical decisions impact individuals or society as a whole.
Explainable AI (XAI)
Furthermore, Explainable AI fosters collaboration between human experts and AI systems. Having access to interpretable explanations enhances human-AI interaction, allowing domain experts to validate and refine the decision-making process. For example, in medical diagnosis, doctors can better assess the recommendations provided by AI algorithms by understanding the underlying reasoning. This collaboration between human and machine results in more accurate, reliable, and useful outcomes.
Explainable AI techniques may vary based on the AI model and the requirements of the application. Methods like rule-based explanations, visualizations, feature importance, and natural language explanations can be employed to provide insights into the decision-making process. The goal is to strike a balance between accuracy and intelligibility, ensuring that the explanations are both accessible and informative to various stakeholders.
In conclusion, the demand for Explainable AI (XAI) is gaining momentum as AI systems become more integrated into our lives. The need for transparency, trust, fairness, and accountability drives the urgency to bridge the gap between human understanding and AI decision-making. By adopting XAI, we unlock the potential of AI systems while ensuring they align with our values and effectively contribute to the betterment of society.
What are the potential ethical and legal implications of using AI systems without the ability to explain their decisions and actions?
Using AI systems without explainability raises several ethical and legal concerns.
1. Ethical implications of opacity
Lack of explainability in AI can lead to potential ethical issues. For instance, if an AI system denies a loan or decides on a prison sentence, individuals affected by these decisions may have the right to know why these outcomes were generated. The opacity of AI systems raises concerns related to fairness, accountability, and transparency.
2. Algorithmic bias
Without explainability, it becomes difficult to identify and address algorithmic biases that might be present in AI systems. These biases can lead to unjust discrimination or unfair treatment of individuals based on protected characteristics such as race, gender, or religion. Explainability is crucial to understanding and rectifying such biases.
3. Legal challenges
Several legal frameworks, such as the European Union’s General Data Protection Regulation (GDPR), give individuals the right to an explanation for automated decisions that significantly impact them. The lack of explainability in AI systems may violate these legal requirements, leading to potential legal consequences for non-compliance.
4. Lack of accountability
Without the ability to understand how an AI system arrived at a decision or action, it becomes challenging to hold anyone accountable for any negative consequences or errors that may occur. Explainability is essential for holding both individuals and organizations responsible for the actions and decisions made by AI systems.
5. Safety and trust
In critical domains such as healthcare or autonomous vehicles, the inability to explain AI decisions can lead to safety concerns. Without transparency, it becomes difficult for users and stakeholders to trust AI systems fully, potentially hindering their widespread adoption.
To address these implications, researchers and practitioners are working on developing explainable AI (XAI) techniques that provide insights into AI decision-making processes while balancing transparency with preserving proprietary algorithms and safeguarding user privacy.
In what ways can the implementation of Explainable AI (XAI) improve transparency, accountability, and trust in AI-powered systems?
Explainable AI (XAI) can improve transparency, accountability, and trust in AI-powered systems in several ways:
1. Interpretable decision-making
XAI allows users and stakeholders to understand the reasoning and decision-making process of AI models. It provides explanations for the predictions and outputs, enabling users to assess the model’s behavior and determine the factors influencing the results. This transparency improves trust in the system and reduces the “black box” perception associated with traditional AI.
2. Detecting biases and discrimination
XAI techniques can help identify any biases or discriminatory patterns in AI models. By understanding why certain decisions were made, it becomes possible to detect and address biases in the training data or the model’s decision-making process. This promotes accountability and guards against potential discriminatory outcomes.
3. Error detection and debugging
XAI allows for the identification of errors or faulty reasoning in AI models. By providing detailed explanations, it becomes easier to identify and rectify any issues within the system. This promotes accountability and ensures that any errors can be corrected promptly.
4. User feedback and confidence
XAI enables users to provide feedback on the explanations generated by the AI system. This feedback loop helps improve the interpretability and accuracy of explanations over time. Additionally, when users understand the reasoning behind AI system outputs, they can develop more confidence in the system, leading to increased trust and acceptance.
5. Regulatory compliance
XAI can assist organizations in complying with regulations and standards related to transparency and accountability. By providing interpretable explanations, organizations can demonstrate their commitment to fairness, ethical decision-making, and compliance with legal requirements. This fosters trust among regulators, stakeholders, and users.
6. Explainability during model development
XAI techniques facilitate the development and validation of AI models. By explaining how the model processes and represents information, developers can better understand its strengths, limitations, and potential biases. This contributes to the development of more robust, fair, and accountable AI systems.
Overall, the implementation of XAI helps address concerns related to transparency, accountability, and trust, making AI systems more trustworthy and widely accepted.
What are the current challenges and limitations in developing and deploying XAI techniques, and how can they be addressed to promote wider adoption of explainable AI
There are several current challenges and limitations in developing and deploying Explainable Artificial Intelligence (XAI) techniques. Addressing these challenges is crucial to promote wider adoption of XAI. Some of the key challenges include:
1. Complexity of AI models
Deep learning and complex AI models often lack interpretability due to their black-box nature. These models have numerous layers and parameters, making it challenging to understand how and why specific decisions are made.
2. Trade-off between performance and interpretability
There is often a trade-off between model performance and interpretability. As models become more interpretable, their performance may decrease. Striking the right balance between these two factors is essential.
3. Lack of standardization
Currently, there is no widely accepted and standardized framework for evaluating and comparing various XAI techniques. This makes it difficult to assess the reliability and usefulness of these methods.
4. Human-Computer Interaction (HCI) challenges
Presenting explanations to users in a comprehensible and meaningful way is a significant challenge. Translating complex AI reasoning into understandable explanations that humans can readily grasp is an ongoing research problem.
To address these challenges and promote wider adoption of XAI, several steps can be taken:
1. Development of transparent models
Researchers can focus on developing AI models that inherently provide interpretability and transparency, such as rule-based systems or decision trees. These models are often more explainable by design.
2. Integration of XAI techniques into existing models
XAI methods can be integrated into currently deployed black-box models, such as deep neural networks. This can be done by incorporating interpretability methods like attention mechanisms or layer-wise relevance propagation.
3. Standardization and benchmarking
The development of standardized evaluation metrics and benchmark datasets can enable fair comparisons between different XAI techniques. This would facilitate the identification of the most reliable and effective methods.
4. Collaboration between researchers and domain experts
Collaboration between AI researchers and domain experts can enhance the interpretability of AI models. Domain experts can provide insights and constraints to ensure the explanations align with human understanding and expectations.
5. User-centric explanation interfaces
Making systems that are easy to use and focused on the user can help explain things in a way that end users can understand and trust. HCI study can help a lot when it comes to coming up with effective ways to explain things.
Getting rid of these problems and limitations will make it easier for AI methods to be used in more areas, ensuring that AI decision-making is transparent, trustworthy, and accountable.