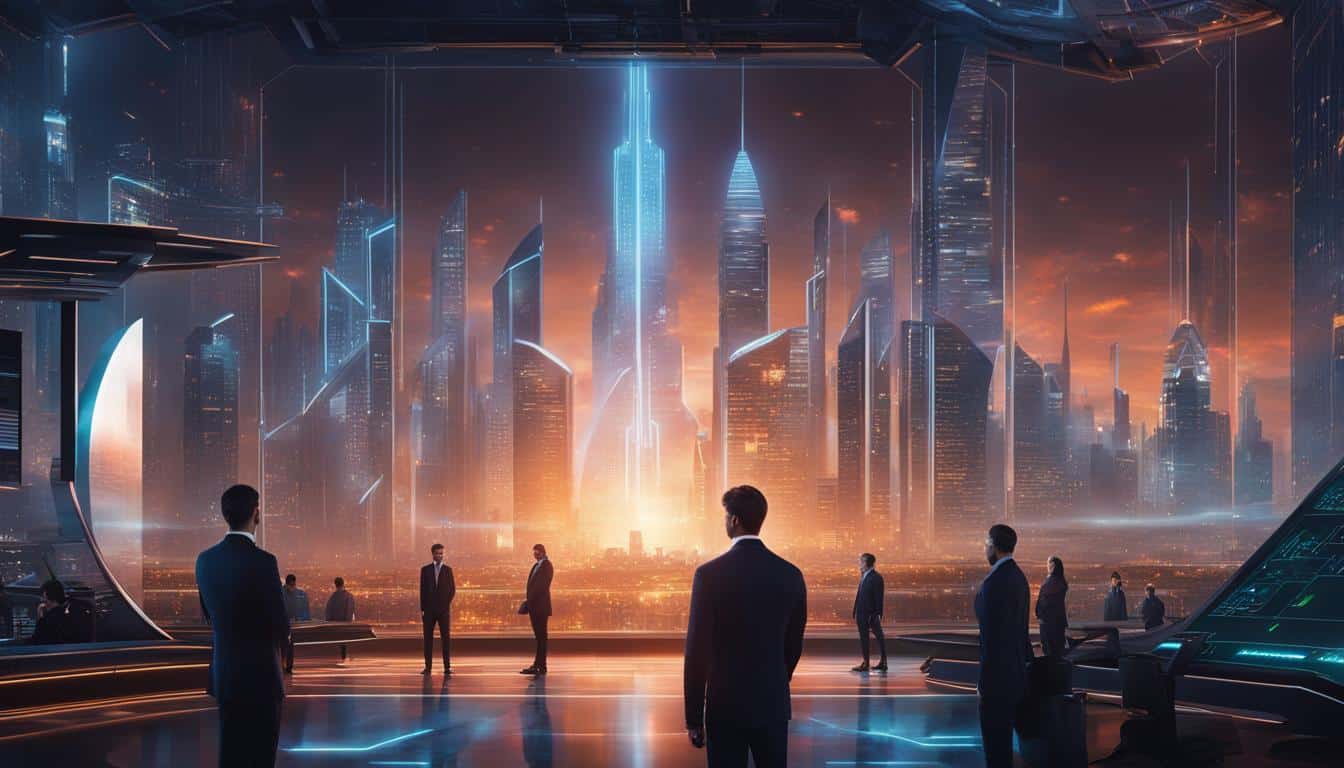
Credit rating agencies play a vital role in the financial ecosystem by evaluating the creditworthiness of individuals and businesses, providing valuable information to lenders and investors. Traditionally, credit rating agencies relied on manual evaluation processes that were time-consuming and subject to human biases. However, with the introduction of AI, these agencies can now leverage advanced algorithms and machine learning models to analyze vast amounts of data, including financial records, payment histories, and economic indicators, to make more informed credit decisions.
AI-powered credit rating systems have demonstrated their ability to improve the accuracy of credit assessments, leading to fairer and more reliable credit ratings. By using AI, credit rating agencies can detect subtle patterns and identify correlations that humans may overlook. This technology enables them to assess the creditworthiness of borrowers more objectively and make more precise predictions about default risks.
Key Takeaways:
- AI is transforming credit rating agencies by enhancing their efficiency and accuracy.
- AI technology enables credit rating agencies to analyze vast amounts of data for better credit assessments.
- AI-powered credit rating systems can detect subtle patterns and make more precise predictions about default risks.
- The adoption of AI in credit rating agencies leads to fairer and more reliable credit ratings.
- AI is reshaping the way creditworthiness is assessed, providing valuable insights to lenders and investors.
The Impact of AI and ML in the Financial Sector
The financial sector has undergone a significant transformation with the adoption of artificial intelligence (AI) and machine learning (ML) systems. These technologies have revolutionized the industry, enabling financial institutions to enhance client experiences and improve operational efficiency.
One of the key benefits of AI and ML in finance is the automation of processes. This has led to cost savings and increased productivity, allowing financial institutions to allocate resources more effectively. AI and ML systems also enable predictive analytics, empowering institutions to make data-driven decisions and identify potential risks or opportunities.
Moreover, AI and ML have improved risk and fraud management in the financial sector. These technologies have the ability to analyze vast amounts of data in real-time, detecting patterns and anomalies that might go unnoticed with traditional methods. This has enhanced the accuracy of risk assessment models and strengthened fraud prevention measures.
AI and ML systems have revolutionized the financial sector, reshaping client experiences and improving operational efficiency.
The potential value of AI in the banking sector alone is estimated to reach $1 trillion. However, the adoption of AI and ML systems also poses unique challenges and risks that need to be addressed. One of the main concerns is the lack of explainability in AI models, which can make it difficult to understand the reasoning behind certain decisions. This raises questions about transparency and accountability, especially in critical areas such as credit rating and lending.
In addition, the financial sector must also be mindful of cyber risks and privacy concerns associated with AI adoption. As AI systems become more sophisticated, the potential for data breaches and malicious attacks increases. Financial institutions need to develop robust cybersecurity measures and ensure the protection of sensitive customer information.
The Impact of AI and ML in the Financial Sector
Despite these challenges, the impact of AI and ML in the financial sector is undeniable. These technologies have the potential to drive financial deepening and improve overall efficiency in the industry. Financial institutions that successfully integrate AI and ML systems into their operations will gain a competitive edge in the fast-paced and evolving financial landscape.
Table: Benefits and Challenges of AI and ML in the Financial Sector
Benefits | Challenges |
---|---|
Automation of processes | Lack of explainability in AI models |
Predictive analytics for data-driven decisions | Cyber risks and privacy concerns |
Improved risk and fraud management |
As AI technology continues to advance, the financial sector should embrace these innovations while ensuring proper regulatory oversight and safeguards. Collaboration between regulators, industry stakeholders, and academia is crucial to develop ethical guidelines and governance frameworks that promote responsible and transparent use of AI in finance.
AI and ML in Credit Risk Assessment
Artificial intelligence (AI) and machine learning (ML) technologies have revolutionized the field of credit risk assessment. These advanced technologies have transformed the way credit rating agencies evaluate risk for individual customers and commercial entities, as well as analyze credit risk within the wider credit value chain.
Through the use of AI and ML models, credit rating agencies can now analyze complex datasets to provide real-time insights and dynamically adjust risk profiles. This enables more accurate and efficient underwriting processes, reduces losses, and improves portfolio monitoring and collection models.
Incorporating AI and ML technologies into credit risk assessment allows for a deeper understanding of creditworthiness, enabling more informed lending decisions. With the ability to process vast amounts of data and detect patterns that may not be apparent to human analysts, AI and ML models enhance the accuracy and effectiveness of credit risk analysis. This leads to better risk management strategies and improved overall financial performance.
Benefits of AI and ML in Credit Risk Assessment | Challenges in AI and ML Adoption |
---|---|
|
|
As credit rating agencies continue to embrace AI and ML technologies, they will further optimize credit risk assessment processes and enhance the accuracy of credit scoring models. The integration of big data analytics and advancements in credit scoring algorithms will shape the future of credit rating agencies, transforming the way creditworthiness is evaluated and lending decisions are made.
ML Techniques for Credit Risk Analysis
When it comes to credit risk analysis, machine learning (ML) techniques are playing a crucial role in revolutionizing the process. These techniques, such as artificial neural networks, random forest, and boosting, are enabling financial institutions to better understand and process credit data, leading to more accurate risk assessments.
Artificial neural networks are powerful ML models that can handle non-linear and interactive patterns between variables. They are particularly useful for analyzing complex credit datasets and uncovering hidden insights. Random forest, on the other hand, creates decision trees to aggregate information and make predictions. By considering multiple decision trees, random forest models can provide more robust and accurate credit risk assessments. Lastly, boosting, a variant of random forest, takes the relationship between decision trees into account, producing even more optimized results.
By leveraging these ML techniques, financial institutions can enhance the accuracy and efficiency of their credit risk analysis models. These techniques enable better risk assessment, allowing institutions to make more informed lending decisions. Moreover, ML techniques can optimize underwriting processes, reduce losses, and improve portfolio monitoring and collection models. Through the application of artificial neural networks, random forest, and boosting, credit risk analysis is undergoing a transformation that leads to improved credit assessment and risk management.
Opportunities and Challenges of AI in Regulatory Compliance
However, the adoption of AI in regulatory compliance also poses unique challenges. Financial regulators need to navigate issues related to the explainability and transparency of AI models. It is essential to ensure that AI systems are fair, unbiased, and comply with legal and regulatory requirements. The lack of interpretability in AI models can make it difficult for regulators to understand the basis of decisions made by these systems. Additionally, there are concerns about the potential for AI models to reinforce biases or discriminate against certain individuals or groups. Striking a balance between the adoption of AI technology and regulatory oversight is crucial to ensure the responsible and ethical use of AI in regulatory compliance.
Opportunities of AI in Regulatory Compliance | Challenges of AI in Regulatory Compliance |
---|---|
|
|
The Future of AI in Credit Rating Agencies
Artificial intelligence (AI) is changing credit rating agencies, making credit analysis more precise and efficient. AI-driven credit analysis will enhance credit scoring models and allow for better risk assessment. As technology grows, credit rating agencies can use machine learning algorithms to create more sophisticated risk assessment models. These advances in credit scoring algorithms will modernize credit rating agencies and provide deeper insights into creditworthiness. AI can help credit rating agencies evaluate creditworthiness more accurately, improving credit risk measurement and analysis.
The Benefits of AI-driven Credit Analysis
AI-driven credit analysis offers numerous advantages for credit rating agencies. First and foremost, it enhances the accuracy and efficiency of credit scoring models, enabling more precise risk assessment. Additionally, AI algorithms are capable of analyzing vast amounts of data in real-time, enabling credit rating agencies to adapt risk profiles dynamically. This ability to assess risk in real-time ensures that credit rating agencies have access to the most up-to-date information when making credit decisions.
Furthermore, AI-driven credit analysis optimizes underwriting processes, reducing losses and improving portfolio monitoring and collection models. By automating and streamlining these processes, credit rating agencies can achieve greater operational efficiency and deliver faster and more reliable credit assessments. Ultimately, the integration of AI into credit rating agencies paves the way for a more advanced and accurate credit assessment landscape.
Benefits of AI-driven Credit Analysis |
---|
Enhanced accuracy and efficiency of credit scoring models |
Real-time analysis of vast data sets for dynamic risk assessment |
Optimized underwriting processes for reduced losses |
Improved portfolio monitoring and collection models |
The future of credit rating agencies lies in the continued integration of AI technology. AI-driven credit analysis will enhance the accuracy and efficiency of credit scoring models, ensuring more precise risk assessment. With advances in credit scoring algorithms and the utilization of big data, credit rating agencies are poised to modernize and shape the future of credit assessment.
The Digital Divide and AI Adoption in Developing Economies
Access to artificial intelligence (AI) technologies and the benefits they offer is not evenly distributed across the globe. Developing economies often face challenges in adopting AI due to limited resources, lack of investment, and a growing digital divide. While advanced economies have embraced AI and reaped its rewards, there is a pressing need to bridge this gap and ensure that developing economies also have access to the transformative power of AI.
The digital divide refers to the gap between those who have access to digital technologies and those who do not. This divide extends to AI technologies, which require robust digital infrastructure, skilled workforce, and supportive business environments. Developing economies face barriers such as inadequate internet connectivity, limited research and development capabilities, and insufficient investment in AI initiatives. Bridging this digital divide requires targeted efforts to address these challenges and create an inclusive environment for AI adoption.
“Bridging the digital divide requires a digital-friendly policy framework focused on infrastructure investment, supportive business environments, skills development, and risk management frameworks.”
Moreover, it is essential to recognize the unique needs and challenges of developing economies when it comes to AI adoption. This includes addressing concerns about data privacy, regulatory frameworks, and ethical considerations. By tailoring AI strategies to the specific context of these economies, policymakers can maximize the benefits of AI while minimizing potential risks.
Ensuring Financial Stability in AI Adoption
The adoption of artificial intelligence (AI) in the financial sector has brought numerous benefits, but it also raises concerns about financial stability. One of the key challenges is the explainability of AI models. AI/ML-based decisions may lack transparency, making it difficult for stakeholders to understand the reasoning behind the outcomes. This lack of explainability can create uncertainty and undermine trust in AI systems. Regulators and industry stakeholders must prioritize the development of explainable AI models to ensure transparency and accountability in decision-making processes.
Another important consideration is the cyber risks associated with AI adoption. As AI systems become more sophisticated and interconnected, they also become more vulnerable to cyber threats. Hackers could exploit vulnerabilities in AI algorithms or manipulate AI models to achieve malicious objectives. Financial institutions and regulators need to implement robust cybersecurity measures to protect AI systems from cyber attacks and ensure the integrity and confidentiality of data used in AI algorithms.
Financial stability issues can arise due to the robustness of AI algorithms and the reliance on a few AI/ML service providers.
Moreover, the financial sector’s reliance on a few AI/ML service providers can pose risks to financial stability. If these providers experience disruptions or fail to meet their obligations, it could have significant implications for the functioning of the financial system. To mitigate this risk, regulators should promote competition, diversity, and resiliency in the AI/ML service provider landscape. This can be achieved through regulatory measures that promote market competition, encourage the development of open-source AI tools, and establish contingency plans for AI service disruptions.
Global Collaboration for AI Regulation
The ethical risks associated with the adoption of AI in the financial sector have highlighted the need for global collaboration for effective regulation. As AI technologies continue to advance, it becomes crucial to ensure responsible and ethical use, while also addressing potential risks. Global collaboration can play a significant role in shaping the regulatory framework and minimizing the negative impacts of AI adoption.
One key aspect of global collaboration is addressing the ethical risks of AI. The development and use of AI systems raise concerns about bias, fairness, and transparency. By collaborating on ethical standards and guidelines, countries can collectively work towards ensuring that AI systems are developed and deployed in an ethical manner. This includes promoting transparency in AI algorithms, avoiding bias in decision-making processes, and protecting privacy and data security.
“Global collaboration is essential in developing a comprehensive regulatory framework for AI. By sharing knowledge, pooling resources, and collaboratively addressing ethical risks, countries can collectively shape the future of AI adoption in the financial sector.”
Another area where global collaboration is crucial is in digital infrastructure investment. Developing economies often face challenges in adopting AI due to limited access to digital infrastructure and resources. By collaborating on digital infrastructure investment, countries can help bridge the digital divide and ensure that all nations have the necessary resources to adopt and benefit from AI technologies. This includes investments in broadband connectivity, data centers, and AI research and development centers.
Key Points:
- Global collaboration is crucial for regulating AI and mitigating ethical risks.
- Ethical standards and guidelines need to be developed collaboratively to ensure responsible use of AI.
- Digital infrastructure investment is essential to bridge the digital divide and enable AI adoption in developing economies.
The Potential of AI in Finance Post-COVID-19
The pandemic has made financial institutions turn to AI for solutions. AI is being used in customer relationship management to improve customer experience. AI-powered chatbots and virtual assistants analyze customer data and provide tailored recommendations.
AI-powered chatbots and virtual assistants are being employed to enhance customer interactions and provide personalized support.
AI in finance
As the financial sector continues to embrace AI post-COVID-19, there are several key benefits that are expected to emerge. AI-driven automation will contribute to greater operational efficiency, allowing financial institutions to streamline processes, reduce costs, and allocate resources more effectively. Furthermore, AI has the potential to improve fraud detection and prevention, as machine learning algorithms can quickly identify anomalous behavior and patterns indicative of fraudulent activity. By leveraging AI’s capabilities, financial institutions can minimize risks, protect their customers, and safeguard the integrity of financial systems.
The adoption of AI in finance post-COVID-19 is not without challenges. One of the primary concerns is the ethical use of AI, particularly in areas like credit scoring and lending decisions. It is crucial for financial institutions to ensure that AI algorithms do not perpetuate bias or discriminate against certain individuals or communities. Transparency and accountability in AI decision-making are essential to build trust and maintain fairness in the financial sector. Additionally, robust cybersecurity measures must be put in place to protect AI systems from potential attacks, safeguard sensitive data, and ensure the confidentiality and integrity of financial transactions.
Table: AI Applications in Finance Post-COVID-19
Application | Benefits |
---|---|
Customer Relationship Management (CRM) | Improved customer interactions, personalized support, enhanced satisfaction |
Risk Management | Real-time risk assessment, proactive risk mitigation, enhanced resilience |
Operational Efficiency | Streamlined processes, cost reduction, effective resource allocation |
Fraud Detection and Prevention | Quick identification of fraudulent activity, enhanced risk mitigation |
Ethical Considerations | Fairness, transparency, and accountability in AI decision-making |
Cybersecurity | Protection of AI systems, data privacy, and financial transaction security |
Regulatory Considerations for AI Adoption
As the adoption of AI in the financial sector continues to accelerate, regulatory considerations become paramount in ensuring the responsible and ethical use of these technologies. Several challenges need to be addressed to strike a balance between innovation and risk management. One of the key challenges is the explainability of AI models. Regulators must ensure that AI systems can provide transparent and interpretable explanations for their decisions, especially in critical areas like credit risk assessment.
Prudential oversight of AI systems is another crucial aspect of regulatory considerations. Regulators need to strengthen their capabilities in monitoring AI/ML-based systems to ensure their compliance with existing regulations and ethical standards. This includes establishing effective governance frameworks to manage risks associated with AI adoption, such as bias, privacy concerns, and cybersecurity.
AI ethics and governance are also essential components of regulatory considerations. Regulators must collaborate with industry stakeholders and academia to develop guidelines and standards for the responsible use of AI in finance. This includes addressing ethical risks, ensuring transparency and accountability, and promoting fairness and inclusivity in AI-driven decision-making processes.
“Regulatory considerations play a crucial role in shaping the adoption and deployment of AI in the financial sector. By establishing clear guidelines and frameworks, regulators can foster innovation while safeguarding against potential risks. It is imperative for regulators to strike a balance between promoting AI adoption to enhance financial services and mitigating the ethical and operational challenges that arise.” – Financial Regulatory Authority
Prudential Oversight of AI Systems
To oversee AI systems in finance, we need to monitor and supervise their use to maintain financial system stability and integrity. Regulators should have the skills and resources to assess AI’s reliability and soundness by evaluating its data, performance, and potential risks to financial institutions and consumers.
Prudential oversight should also focus on assessing AI’s resilience to external threats like cyberattacks and data breaches. Regulators should work with industry stakeholders to establish cybersecurity best practices and standards for AI adoption, including protecting sensitive data, ensuring secure infrastructure, and promoting continuous risk assessment.
Regulatory Considerations | Challenges | Solutions |
---|---|---|
Explainability of AI Models | Lack of transparency in AI decision-making | Regulations requiring AI models to provide interpretability and explainability |
Prudential Oversight of AI Systems | Limited expertise in assessing AI systems | Investment in regulatory capabilities and collaboration with industry stakeholders |
AI Ethics and Governance | Unaddressed ethical risks and lack of standards | Development of guidelines and collaboration with academia and industry |
Conclusion
Artificial intelligence (AI) has improved credit rating agencies and the financial sector. AI has increased efficiency and accuracy in credit assessments. AI development will continue to shape the future of credit rating agencies.
However, AI adoption in finance presents regulatory challenges. Ethical and responsible AI use requires consideration of factors such as explainability, oversight, and cyber risks. Collaboration between regulators, industry stakeholders, and academia is vital for effective regulations.
The future of AI in credit rating agencies is promising. Advanced AI-driven credit analysis will enhance accuracy and efficiency. Big data and improved credit scoring algorithms will further modernize credit rating agencies and credit assessments.
AI technology is developing rapidly. To maintain the stability of the financial system, it is important to balance innovation with responsible AI adoption.
FAQ
How is AI transforming credit rating agencies?
AI is transforming credit rating agencies by improving efficiency, accuracy, and risk assessment models through the use of machine learning techniques.
What are the benefits of AI and ML in the financial sector?
AI and ML bring benefits such as automation of processes, cost savings, predictive analytics, improved risk and fraud management, and more effective credit risk measurement and analysis.
How do ML techniques enhance credit risk analysis?
ML techniques, such as artificial neural networks, random forest, and boosting, enhance credit risk analysis by processing credit data, managing non-linear patterns between variables, and aggregating insights to improve accuracy and efficiency.
What opportunities and challenges does AI present in regulatory compliance?
AI presents opportunities to expand access to consumer credit and modernize compliance processes, but also poses challenges in terms of regulatory evaluation, due diligence, and balancing transparency and performance.
What is the future of AI in credit rating agencies?
The future of credit rating agencies lies in the continued integration of AI technology, which will enhance credit scoring accuracy and efficiency, and contribute to the modernization of credit assessment.
How has the COVID-19 pandemic accelerated the adoption of AI in the financial sector?
The pandemic has accelerated the adoption of AI in areas such as customer relationship management, risk management, underwriting processes, fraud detection, and post-pandemic intensive supervision activities.
What regulatory considerations are necessary for AI adoption in the financial sector?
Regulatory considerations include addressing the explainability of AI models, prudential oversight of AI systems, ethical concerns, and collaboration between regulators, industry stakeholders, and academia.
Source Links
- https://www.elibrary.imf.org/view/journals/087/2021/024/article-A001-en.xml
- https://www.birlasoft.com/articles/ai-machine-learning-and-future-credit-risk-management
- https://www.orrick.com/en/News/2023/07/Is-AI-the-Solution-to-Regulatory-Modernization