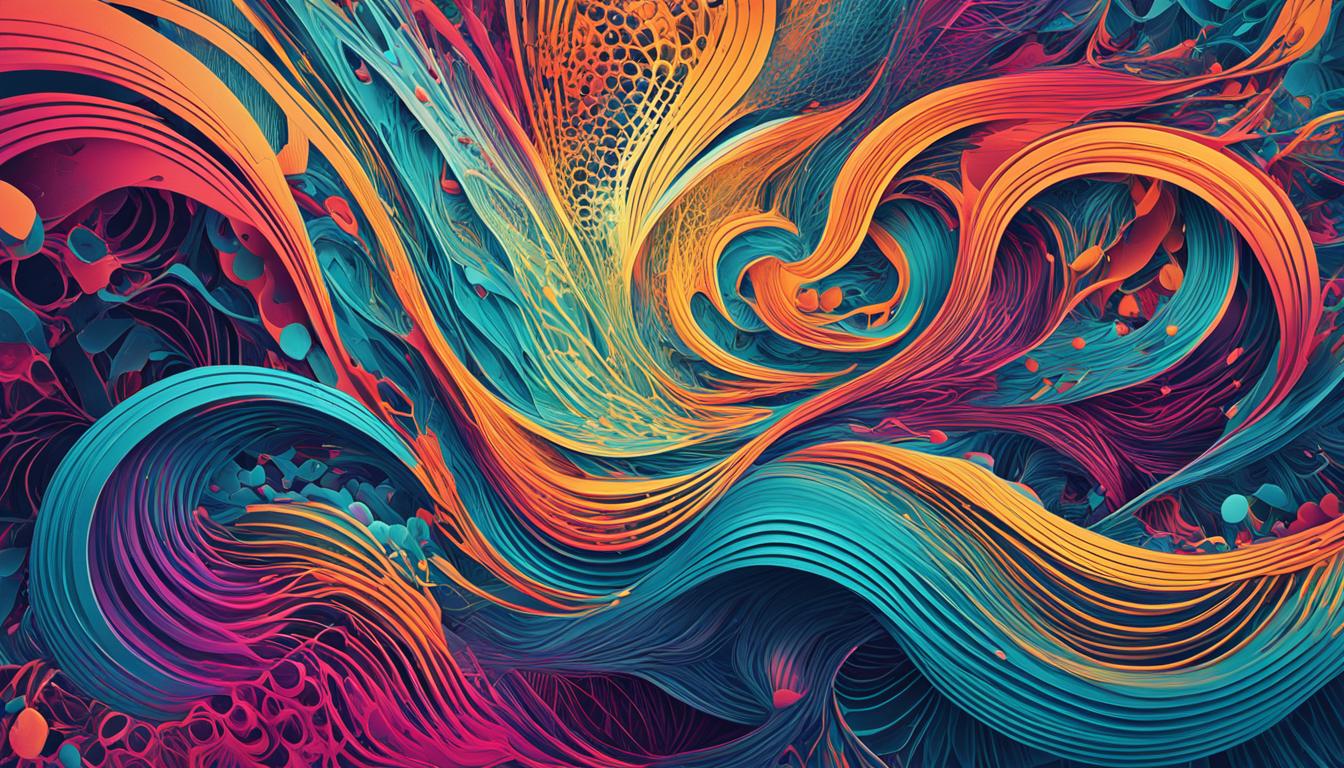
Generative AI has made significant advancements in creating media that is incredibly authentic and realistic, blurring the line between the virtual and the actual. This ability has been made possible by a revolutionary technology that has transformed the capabilities of AI algorithms. By leveraging this technology, generative AI has been able to generate jaw-dropping media that is almost indistinguishable from content created by humans.
Through the combination of sophisticated algorithms and powerful computational resources, AI systems can now produce convincingly authentic media, including images, videos, and even text. This represents a major breakthrough in the field of artificial intelligence and has opened up new opportunities in various industries, including entertainment, advertising, and design.
Key Takeaways:
- Generative AI has the ability to create media that is incredibly authentic and indistinguishable from human-created content.
- Advanced algorithms and powerful computational resources have enabled AI systems to generate convincing images, videos, and text.
- This breakthrough has opened up new opportunities in industries such as entertainment, advertising, and design.
- Deep learning, specifically neural networks, plays a crucial role in enhancing the realism and authenticity of generative AI-generated media.
- Generative adversarial networks (GANs) have significantly contributed to the advancement of AI in media creation by enabling a feedback loop that improves the quality and realism of the generated content.
The Role of Deep Learning in Generative AI’s Media Creation
Deep learning plays a critical role in the creation of authentic media by generative AI. At the heart of this process are neural networks, which are designed to replicate the structure and functioning of the human brain. These neural networks undergo training using vast amounts of data, including images, videos, and text, to learn the intricate patterns, features, and styles that are characteristic of human-created content.
By analyzing the relationships and patterns within the training data, generative AI systems can then generate new media that aligns with the desired style and aesthetics. The use of deep learning algorithms allows the AI system to understand and replicate the complex characteristics of different types of media, resulting in highly convincing and authentic output.
Deep learning has proven to be instrumental in enhancing the realism and authenticity of generative AI-generated media. By leveraging the power of neural networks and a robust training data set, AI systems can create media that mimics human-created content with remarkable precision.
In summary, deep learning is a crucial component in the creation of authentic media by generative AI. By training neural networks on a diverse range of data, including images, videos, and text, generative AI systems can produce convincing and realistic content that showcases the remarkable capabilities of this technology.
The Power of Deep Learning
Deep learning algorithms allow generative AI systems to understand and replicate the complex characteristics of different types of media, resulting in highly convincing and authentic output.
To further illustrate the importance of deep learning in generative AI’s media creation, consider the following example:
Media Type | Training Data | Generated Output |
---|---|---|
Image | An extensive dataset of various images | A new image that captures the style and characteristics of the training data |
Video | A diverse collection of videos | A realistic video that replicates the patterns and features of the training data |
Text | A vast amount of written content in different styles | Authentic text that aligns with the desired style and tone |
The table above demonstrates the versatility of deep learning in generating different types of media. By training on relevant and diverse data sets, deep learning algorithms enable generative AI systems to produce media that is surprisingly authentic and in line with the desired style.
The Advancement of GANs in Media Generation
Generative adversarial networks (GANs) have revolutionized the field of generative AI in media creation. GANs consist of two key components: a generator network and a discriminator network. The generator network is responsible for creating new media, while the discriminator network evaluates the authenticity of the generated media compared to real content.
The training process of GANs involves a feedback loop between the generator and discriminator networks. The generator network initially produces media that is evaluated by the discriminator network. Through this iterative process, the generator network learns from the feedback provided by the discriminator network and adjusts its output to improve its ability to generate authentic media.
With each iteration, the generator network becomes more proficient at creating convincing and realistic content. This continuous improvement through the feedback loop leads to the generation of highly authentic media that is virtually indistinguishable from human-created content.
The training process in GANs is a constant battle between the generator and discriminator networks. The generator network strives to produce media that fools the discriminator into classifying it as real, while the discriminator network becomes more skilled at differentiating between real and generated media.
“The advancement of GANs has propelled generative AI to new heights in media creation. The iterative training process enables AI systems to continually learn and improve their ability to generate authentic and realistic content.”
Through the use of GANs, generative AI has the capability to create media that surpasses expectations in terms of authenticity and realism. These advanced techniques have opened up endless possibilities for industries such as entertainment, design, and advertising, allowing for the creation of highly engaging and visually stunning content.
Exploring the Training Process of GANs
The training process of GANs involves several key steps:
- The generator network initially produces media based on random input or noise.
- The discriminator network then evaluates the authenticity of the generated media.
- The feedback from the discriminator network is used to update and improve the generator network.
- The updated generator network produces new media, and the process repeats.
This iterative training process allows the generator network to refine its output and generate media that closely resembles real content. The constant cycle of feedback and improvement ensures that the generative AI system continually enhances its ability to create authentic and compelling media.
“The training process in GANs is a dynamic interplay between the generator and discriminator networks, pushing the boundaries of generative AI and redefining what is possible in media creation.”
The powerful combination of GANs, with their sophisticated training process, and deep learning algorithms has revolutionized the field of generative AI. The advancement of GANs in media generation has brought us closer to a future where AI-created content can seamlessly coexist with human-created content, pushing the boundaries of what we thought was possible.
Advantages of GANs in Media Generation | Challenges of GANs in Media Generation |
---|---|
|
|
Conclusion
The rapid advancements in generative AI technology have revolutionized the creation of AI-generated media, offering a staggering level of authenticity. Deep learning and neural networks play a pivotal role, enabling AI systems to analyze and replicate patterns and styles from extensive training data. This results in highly realistic output that is virtually indistinguishable from human-created content. However, it is the integration of generative adversarial networks (GANs) that has truly propelled the authenticity of AI-generated media to unprecedented heights.
GANs introduce a feedback loop between a generator and discriminator network, continuously improving the quality and realism of the generated media. This revolutionary technology has paved the way for the creation of highly engaging and realistic media in numerous industries, such as entertainment, advertising, and design. The boundaries between virtual and actuality are now blurred, offering limitless possibilities for content creators and marketers alike.
As generative AI continues to evolve, we can anticipate even more groundbreaking advancements in the field of media creation. The combination of neural networks, deep learning, and GANs has unlocked the potential for AI to generate highly authentic content at an unprecedented level. The future holds remarkable prospects for AI-generated media, where authenticity knows no bounds and technology seamlessly blends virtual and actual worlds.
FAQ
What is generative AI?
Generative AI refers to the use of artificial intelligence algorithms to generate media, such as images, videos, and text, that is almost indistinguishable from content created by humans.
How does deep learning contribute to generative AI’s media creation?
Deep learning, specifically neural networks, plays a crucial role in generative AI by allowing the system to analyze patterns and styles in training data and replicate them in the generated media, resulting in more realistic output.
What are GANs and how do they advance generative AI?
GANs, or generative adversarial networks, consist of two neural networks—the generator and the discriminator. The generator creates new media, while the discriminator evaluates the authenticity of the generated content. Through iterative training, GANs improve the quality and realism of the AI-generated media.
What is the secret behind the authenticity of AI-generated media?
The authenticity of AI-generated media is a result of technologies such as deep learning and GANs that enable the system to analyze data patterns, replicate human characteristics and styles, and improve the quality and realism over time.