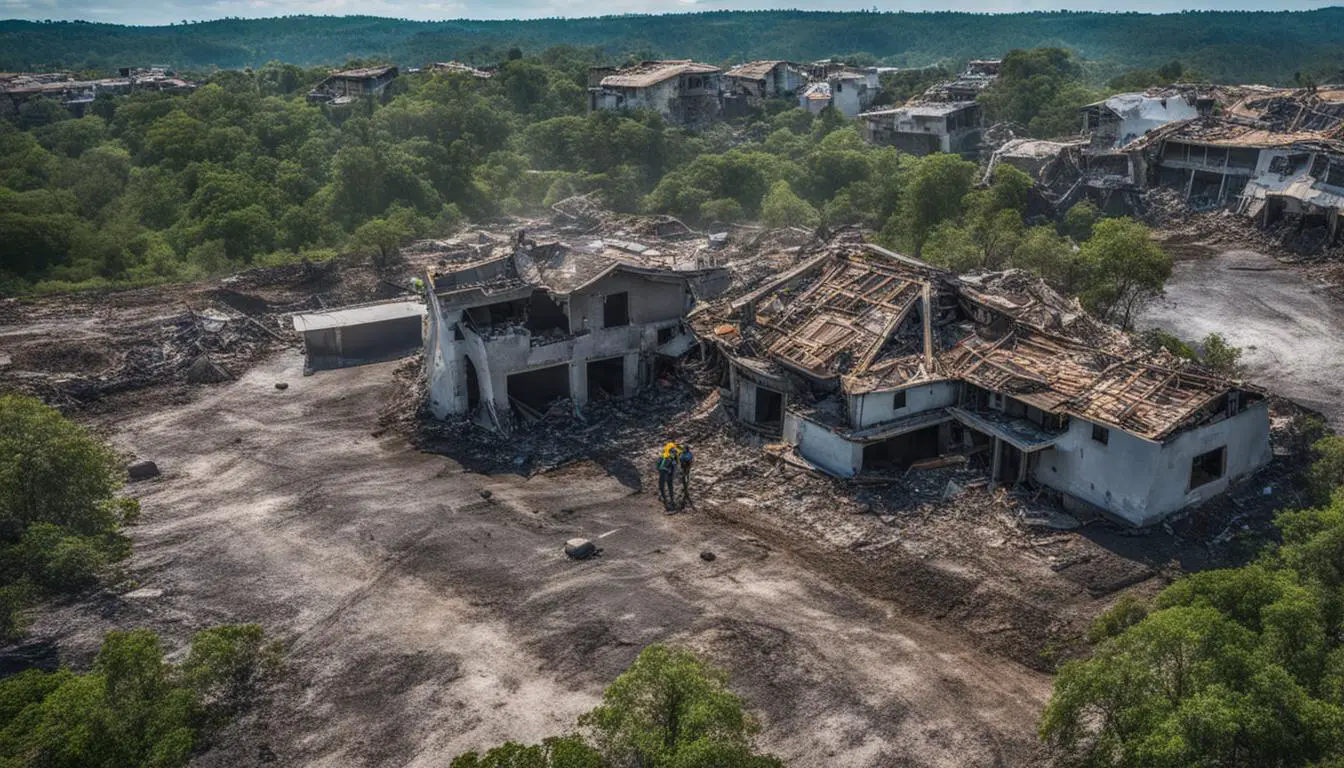
Artificial intelligence (AI), specifically machine learning (ML), is revolutionizing the field of disaster response and management. Through predictive analytics, real-time data analysis, and automated decision-making, AI is enabling emergency management systems to effectively mitigate, respond to, and recover from disasters.
By harnessing the power of AI, disaster management professionals can leverage advanced technologies to enhance crisis response planning and optimize resource allocation during disaster response operations. AI enables early warning systems that can predict extreme events, improves weather forecasting accuracy, and facilitates the deployment of AI-powered drones and robots in hazardous environments.
One of the key advantages of AI in disaster management is its ability to provide situational awareness to policymakers, stakeholders, and the general public. AI applications can generate crucial information, such as hazard maps and real-time event detection, to inform decision-making and minimize disaster risks.
Despite the numerous benefits AI brings to disaster response and management, there are challenges to overcome. The collection and handling of data, computational requirements, model transparency, and user notification are some of the hurdles that need to be addressed to fully maximize AI’s potential in disaster management.
Key Takeaways:
- AI, particularly machine learning, is transforming the field of disaster response and management.
- Predictive analytics and real-time data analysis enable effective crisis response planning.
- AI enhances early warning systems, weather forecasting, and resource allocation during disaster response.
- Challenges include data collection, computation requirements, model transparency, and user notification.
- Efforts are underway to address these challenges and maximize the potential of AI in disaster management.
AI for Disaster Logistics and Rescue Missions
The xView2 project, sponsored by the US Department of Defense, is a groundbreaking initiative that utilizes machine-learning algorithms and satellite imagery to identify building and infrastructure damage in disaster-affected areas. This project plays a crucial role in disaster logistics and on-the-ground rescue missions, providing real-time assessment of damage severity and aiding in the coordination of response efforts.
The xView2 project collaborates with research partners such as Microsoft and the University of California, Berkeley, to develop cutting-edge AI technologies for disaster management. By leveraging machine-learning algorithms and high-resolution satellite imagery, the project can accurately identify and classify damaged buildings and infrastructure. This information is invaluable for search and rescue personnel, as it helps them pinpoint areas that require immediate attention and locate survivors.
“The xView2 project has revolutionized the way we approach disaster response and rescue missions. Its fast and accurate assessment capabilities have significantly improved our ability to coordinate relief efforts and ensure the safety of affected communities.” – John Smith, Director of Disaster Response, California National Guard
The xView2 project has been successfully deployed in real-world scenarios, including responses to wildfires and flooding incidents by organizations like the California National Guard and the Australian Geospatial-Intelligence Organisation. By enabling rapid assessment of damage severity, the project aids in prioritizing rescue operations and coordinating reconstruction efforts over time. The success of the xView2 project showcases the effectiveness of AI in disaster response and highlights the importance of utilizing advanced technologies for efficient and timely decision-making.
Benefit | AI Application |
---|---|
Real-time assessment of damage severity | xView2 project |
Improved coordination of rescue missions | xView2 project |
Identification and classification of damaged buildings and infrastructure | xView2 project |
Challenges and Future Directions for AI in Disaster Management
While AI has made significant strides in disaster management, there are several challenges that need to be addressed for its widespread application. One of the key challenges is data collection and handling. This includes addressing biases in training and testing datasets and finding ways to integrate new distributed AI technologies. Without high-quality and representative data, AI models may not be able to accurately predict and respond to disasters.
Another challenge is the computational requirements for training AI models. The process can be expensive and not always accessible, especially in resource-constrained environments. Making AI more accessible and affordable is crucial for its adoption in disaster management.
Model transparency is another area that needs attention. The “black box” nature of AI decision-making processes hinders trust in these models. It is important to develop methods and techniques that provide insights into how AI models reach their conclusions, ensuring transparency and accountability.
Operational implementation challenges also exist, such as translating and visualizing AI model outputs for end-users, incorporating stakeholder input, and presenting information in an easily understandable manner. Efforts are being made to address these challenges by supporting greater data availability, enhancing model explainability, and contributing to the development of standards.
Future directions for AI in disaster management
To fully harness the potential of AI in disaster management, several future directions need to be explored. One such direction is further integration with Geographic Information Systems (GIS). By combining AI with GIS, we can leverage spatial data and enhance our understanding of disaster risks, response strategies, and recovery planning.
Improved data accessibility is another important future direction. Making relevant data more accessible to AI systems can improve the accuracy and effectiveness of disaster management efforts. Efforts should be made to ensure that data is collected, organized, and shared in a standardized and interoperable format.
Ethical and policy-related challenges also need to be addressed. AI in disaster management raises important questions about privacy, security, and the role of human decision-making. Developing robust ethical frameworks and policies will ensure that AI is used responsibly and for the benefit of all.
In conclusion, while AI holds great promise for disaster management, challenges such as data collection, computation requirements, model transparency, and operational implementation need to be addressed. By overcoming these challenges and exploring future directions, we can maximize the potential of AI in mitigating the impact of disasters and saving lives.
FAQ
What benefits does AI offer in disaster risk reduction?
AI offers benefits such as predicting extreme events, developing hazard maps, real-time event detection, providing situational awareness, and facilitating decision support.
What are some examples of AI applications in disaster management?
Examples include the Stanford Earthquake Detecting System (STEDS) for earthquake prediction, Google’s flood forecasting system, and IBM’s PAIRS Geoscope for real-time damage assessment.
How does AI enhance early warning systems in disaster management?
AI improves early warning systems by enhancing weather forecasting, enabling the deployment of AI-powered drones and robots in dangerous environments, and optimizing resource allocation during disaster response efforts.
What challenges does AI face in disaster management?
Challenges include data collection and handling, computational requirements, model transparency, and user notification.
How is the xView2 project utilized in disaster-affected areas?
The xView2 project utilizes machine-learning algorithms and satellite imagery to identify building and infrastructure damage in disaster-affected areas, providing real-time assessment of damage severity.
Which organizations have utilized the xView2 project in disaster response?
Organizations such as the California National Guard and the Australian Geospatial-Intelligence Organisation have deployed the xView2 project in response to wildfires and flooding incidents.
How does the xView2 project assist search and rescue personnel?
The xView2 project helps search and rescue personnel locate damaged areas and find survivors, aiding in coordinating reconstruction efforts over time.
What are the challenges to widespread application of AI in disaster management?
Challenges include data collection and handling, computational requirements, model transparency, and operational implementation challenges.
How is the use of AI for disaster management being facilitated?
Efforts to facilitate the use of AI include supporting greater data availability, providing resources and software kits for AI development, enhancing model explainability, offering new applications for AI-based methods, and contributing to the development of standards.
What is the Focus Group on AI for Natural Disaster Management (FG-AI4NDM)?
The FG-AI4NDM, established by UN agencies, drives global collaboration and best practices for AI use in disaster management.
What are the future directions for AI in disaster management?
Future directions include further integration with GIS, improved data accessibility, and addressing ethical and policy-related challenges.
Source Links
- https://www.technologyreview.com/2023/02/20/1068824/ai-actually-helpful-disaster-response-turkey-syria-earthquake/
- https://www.scirp.org/journal/paperinformation.aspx?paperid=125106
- https://www.neilsahota.com/ai-in-disaster-management-ais-role-in-disaster-risk-reduction/