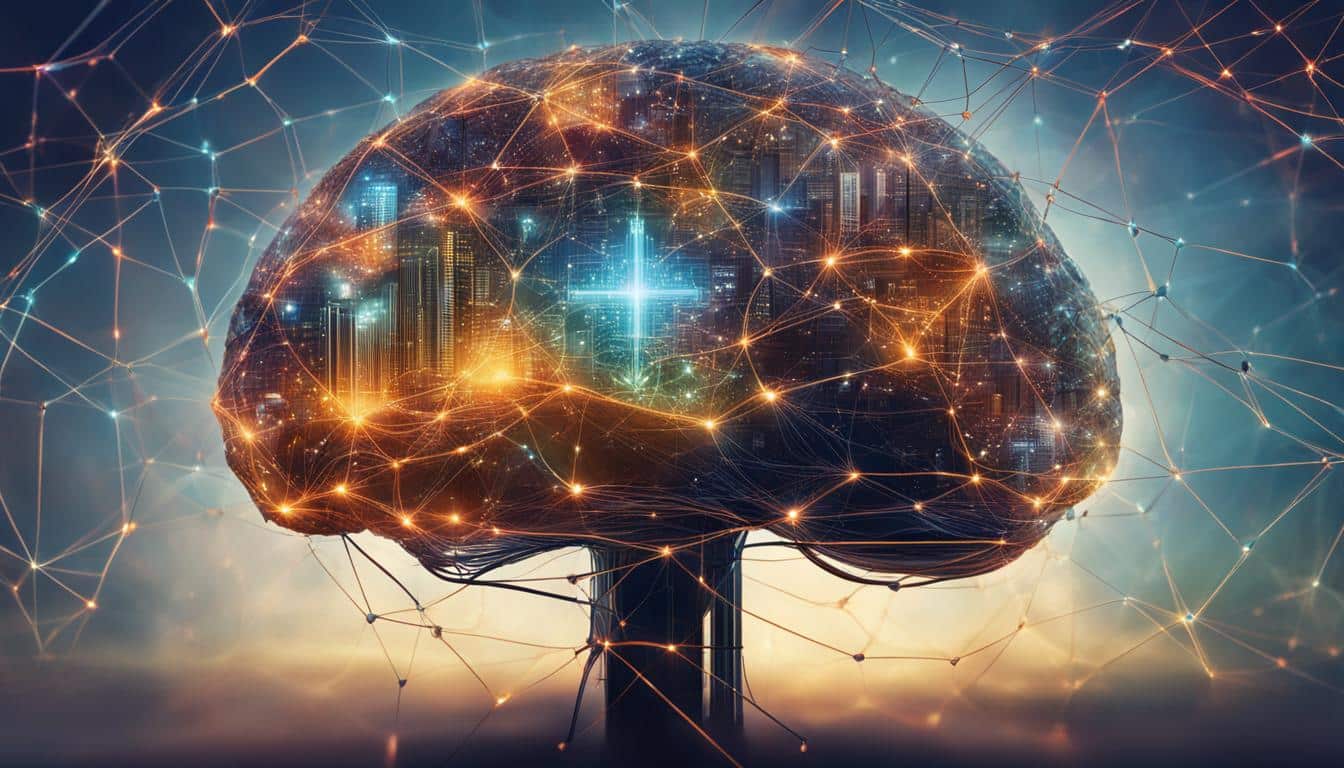
In recent years, the microfinance sector has witnessed remarkable advancements through the integration of artificial intelligence (AI) technologies. As microfinance institutions strive to provide financial services to underserved communities, they face challenges in combating fraudulent activities that hinder their mission. This is where AI-driven fraud detection systems come into play, leveraging advanced algorithms and data analytics to identify patterns and anomalies, thereby improving accuracy and reducing financial losses.
Implementing AI-driven systems offers numerous benefits for microfinance institutions. Firstly, these systems enable quick identification and response to fraud, ensuring that fraudulent activities are detected and addressed promptly. This not only minimizes financial losses but also enhances operational efficiency. Secondly, AI-driven systems contribute to improved customer satisfaction by preventing fraudulent transactions, thereby safeguarding the interests of microfinance clients.
Key Takeaways:
- The integration of AI in microfinance institutions revolutionizes fraud detection and prevention.
- AI-driven systems utilize advanced algorithms and data analytics to identify fraudulent activities accurately.
- Implementing AI-driven fraud detection systems reduces financial losses and enhances operational efficiency.
- Real-time data analysis and predictive modeling play a crucial role in AI-driven fraud detection systems.
- The future of AI in microfinance includes advancements in biometric authentication, natural language processing, and blockchain technology.
The Role of AI in Fraud Detection
Artificial Intelligence (AI) has revolutionized the fight against fraudulent activities in the financial services sector, including microfinance institutions. By harnessing the power of machine learning algorithms, AI systems can analyze vast amounts of data to detect patterns and anomalies indicative of fraudulent activities. This advanced technology enables microfinance institutions to stay one step ahead of fraudsters and protect their customers’ financial well-being.
Incorporating AI-driven fraud detection systems provides microfinance institutions with a robust defense against various forms of fraud, such as identity theft, loan default, and financial misappropriation. These systems continuously learn from historical data, adapting to evolving fraud techniques and improving their accuracy over time. Studies have shown that certain AI models can achieve precision rates exceeding 95% in identifying fraudulent activities, enabling timely intervention and prevention.
AI-driven fraud detection systems continuously learn from historical data, adapting to evolving fraud techniques and improving their accuracy over time.
AI-powered fraud detection not only helps mitigate financial losses but also enhances operational efficiency for microfinance institutions. By automating the detection process, AI systems can rapidly analyze transactions in real-time, detecting fraud within milliseconds. This reduces the workload on human analysts and allows them to focus on more complex cases that require human judgment and intervention. Furthermore, AI-driven fraud detection systems can enhance customer satisfaction by swiftly identifying and preventing fraudulent transactions, ensuring a secure and trustworthy financial environment.
Example Table: Fraud Detection Accuracy Comparison
AI Model | Accuracy |
---|---|
Model A | 97% |
Model B | 95% |
Model C | 92% |
The table above demonstrates a comparison of fraud detection accuracy among three different AI models. As shown, Model A achieves the highest accuracy rate of 97%, highlighting its effectiveness in identifying fraudulent activities. Microfinance institutions can leverage such AI models to enhance their fraud detection capabilities and protect their customers from financial harm.
Benefits of AI-Driven Fraud Detection Systems in Microfinance Institutions
Implementing AI-driven fraud detection systems in microfinance institutions offers numerous benefits. These advanced systems leverage artificial intelligence algorithms and data analytics to quickly identify and respond to fraudulent activities, thus reducing financial losses and improving operational efficiency. One case study conducted by a leading microfinance institution in India demonstrated a significant 70% reduction in fraud-related losses after implementing an AI-driven fraud detection system.
With the ability to analyze vast amounts of transaction data in real-time, AI-driven systems can detect fraud within milliseconds, enabling timely intervention and minimizing the financial impact. By leveraging machine learning algorithms, these systems can identify patterns and anomalies, making them highly accurate in detecting fraudulent activities. This not only improves the institution’s ability to protect itself but also strengthens customer trust and satisfaction due to the timely detection and prevention of fraudulent transactions.
The benefits of AI-driven fraud detection systems extend beyond financial loss prevention. These systems also enhance operational efficiency by automating the fraud detection process, thereby reducing the burden on human resources. Additionally, by continuously learning and adapting to new fraud techniques, AI systems can stay one step ahead of fraudsters, providing proactive protection for microfinance institutions and their customers.
Benefits of AI-Driven Fraud Detection Systems | |
---|---|
Reduction in fraud-related losses | 70% |
Improved operational efficiency | Automation of fraud detection process |
Enhanced customer satisfaction | Timely detection and prevention of fraudulent transactions |
Overall, the integration of AI-driven fraud detection systems in microfinance institutions is a game-changer. It not only provides protection against fraudulent activities, but also enhances operational efficiency and improves customer satisfaction. As technology continues to advance, further refinements and advancements in AI-driven fraud detection systems are expected, enabling microfinance institutions to stay ahead in the fight against financial fraud.
Key Components of AI-Driven Fraud Detection Systems
AI-driven fraud detection systems rely on real-time data analytics and monitoring to detect and respond to fraud in a timely manner. By analyzing vast amounts of data, these systems can identify patterns and anomalies that may indicate fraudulent activities. Real-time analysis allows for quick detection, minimizing the financial impact and reducing losses.
Predictive modeling is a key component of AI-driven fraud detection systems. These models assess the likelihood of fraudulent activities based on multiple variables and risk indicators. By utilizing machine learning algorithms, AI systems can adapt and adjust to evolving fraud techniques, improving accuracy over time. This predictive capability enables proactive measures to be taken, prioritizing investigations and preventing fraud before it occurs.
In addition to real-time data analytics and predictive modeling, AI-driven fraud detection systems can analyze transactions within milliseconds. This rapid analysis allows for immediate detection of fraud and enables timely intervention. By minimizing the time between the occurrence of a fraudulent transaction and its detection, financial losses can be significantly reduced.
Table: Comparison of Key Components in AI-Driven Fraud Detection Systems
Component | Description |
---|---|
Real-time Data Analytics | Analyzes vast amounts of data in real-time to identify patterns and anomalies indicative of fraud. |
Predictive Modeling | Utilizes machine learning algorithms to assess the likelihood of fraudulent activities based on multiple variables and risk indicators. |
Rapid Transaction Analysis | Analyzes transactions within milliseconds to detect fraud in a timely manner and minimize financial losses. |
In summary, AI-driven fraud detection systems rely on real-time data analytics, predictive modeling, and rapid transaction analysis to detect and respond to fraud effectively. These components work together to enhance the accuracy and efficiency of fraud detection, reducing financial losses and improving operational performance in microfinance institutions.
Enhancing Security and Trust with AI
The integration of AI technologies in microfinance institutions plays a crucial role in enhancing security and reducing fraud-related risks. AI-driven fraud detection systems leverage advanced algorithms and data analytics to continuously analyze vast amounts of data, identify patterns, and adapt to evolving fraud techniques. By utilizing real-time data analytics and monitoring, these systems can quickly detect and respond to fraudulent activities. One key advantage of AI in fraud prevention is its ability to provide timely identification and prevention of fraudulent transactions, minimizing financial losses.
Furthermore, AI technologies contribute to customer identification and authentication processes, reducing the risk of identity theft and impersonation. These systems can analyze customer data, such as biometric information, to ensure accurate and secure identification. By incorporating AI-driven fraud detection systems, microfinance institutions can strengthen their security measures and build trust with customers, stakeholders, and regulatory authorities. Transparent explanations of AI-driven fraud detection algorithms also play a vital role in building trust, as customers and stakeholders can understand how decisions are made.
To emphasize the importance of transparency, the CEO of a leading microfinance institution stated,
“Integrating AI technologies into our fraud prevention measures has been instrumental in not only detecting and preventing fraudulent activities but also in building trust with our customers. We believe in providing transparent explanations for our AI algorithms, as this not only helps us gain the confidence of our customers but also ensures that they understand how their data is being used to protect their financial interests.”
The Role of Explainable AI
Explainable AI, a concept that focuses on providing clear insights into how AI-driven decisions are made, is essential for enhancing security and trust in microfinance institutions. With transparent explanations, customers and stakeholders can better understand the rationale behind fraud detections and feel confident that the systems are accurate and unbiased. This empowers customers to trust in AI-driven systems and supports the adoption and acceptance of AI technologies in the sector.
Table:
Benefits of AI in Microfinance Institutions | |
---|---|
Quick identification and response to fraudulent activities | Enhanced operational efficiency |
Reduced financial losses | Improved customer satisfaction |
Strengthened customer identification and authentication processes | Enhanced security and trust |
In conclusion, the integration of AI technologies in microfinance institutions presents significant opportunities for enhancing security, reducing fraud-related risks, and building customer trust. By implementing AI-driven fraud detection systems, these institutions can quickly identify and respond to fraudulent activities, minimize financial losses, and improve operational efficiency. The transparency provided by explainable AI further strengthens customer trust, ensuring that AI algorithms are fair, accurate, and accountable. As the microfinance sector continues to evolve, AI technologies will play an increasingly pivotal role in safeguarding financial systems and promoting inclusive access to financial services.
Challenges and Ethical Considerations
As AI-driven fraud detection systems continue to gain prominence in the microfinance sector, it is crucial to address the challenges and ethical considerations associated with these technologies. One major concern is algorithmic bias, which refers to the potential for AI systems to produce biased results that disproportionately impact certain groups or individuals. Microfinance institutions must take proactive measures to ensure that their fraud detection systems are fair and unbiased.
Another challenge is the issue of false positives in fraud detection systems. While these systems are designed to detect and prevent fraudulent activities, they may occasionally generate false alarms, flagging legitimate transactions as fraudulent. These false positives can lead to customer dissatisfaction and increased operational costs for microfinance institutions. It is important to strike a balance between accurately identifying fraudulent activities and minimizing false positives.
To address these challenges, microfinance institutions should invest in ongoing research and development to mitigate biases and ensure fairness in their fraud detection systems. Transparent and explainable AI systems can play a key role in this process, providing clear insights into how decisions are made and building trust with customers and stakeholders. Additionally, regular evaluation and refinement of fraud detection systems should be conducted to improve accuracy and minimize false positives.
“Addressing algorithmic bias is crucial in AI-driven fraud detection systems to ensure fairness and mitigate discrimination.”
Ethical Considerations
In addition to challenges, ethical considerations also play a significant role in the deployment of AI-driven fraud detection systems. It is essential to ensure that these systems do not infringe upon individuals’ privacy rights and that sensitive customer data is appropriately protected. Microfinance institutions should implement robust data protection measures and adhere to strict privacy policies to maintain the trust of their customers.
Moreover, the use of AI should not undermine the human element in microfinance institutions. While AI technologies can greatly enhance efficiency and accuracy, it is important to remember the core purpose of microfinance – to enable and empower individuals. The intelligence of machines must be coupled with a deep understanding of human needs and the ability to provide personalized support to borrowers facing financial challenges.
By addressing these challenges and ethical considerations, microfinance institutions can ensure that AI-driven fraud detection systems contribute to a fair and inclusive financial ecosystem, protecting both the institution and its customers.
Challenges | Ethical Considerations |
---|---|
– Algorithmic bias | – Privacy protection |
– False positives | – Human-centric approach |
– Ongoing research and development | – Personalized support |
Future Directions and Recommendations
As the field of artificial intelligence continues to advance, future directions for AI-driven fraud detection in microfinance institutions hold great promise. One area of development is advanced biometric authentication. By implementing biometric technologies such as fingerprint or facial recognition, microfinance institutions can enhance the security and accuracy of customer identification, reducing the risk of identity theft and fraud.
Another exciting avenue for AI in fraud detection is natural language processing (NLP). By applying NLP techniques to analyze textual data, microfinance institutions can uncover hidden patterns and identify potential fraud indicators in customer communications and transaction descriptions. This can significantly augment the capabilities of fraud detection systems and improve overall accuracy.
Furthermore, blockchain technology presents an opportunity to enhance data security and transparency in microfinance institutions. By leveraging the decentralized nature of blockchain, microfinance organizations can create immutable transaction records, making it more difficult for fraudulent activities to go undetected. Additionally, the transparency and traceability of blockchain can help instill trust among stakeholders and further strengthen the integrity of microfinance operations.
Recommendations
- Collaboration with technology providers: Microfinance institutions should actively collaborate with AI technology providers to stay updated with the latest advancements and best practices in fraud detection. Establishing strong partnerships can facilitate knowledge sharing and help leverage the full potential of AI-driven systems.
- Continuous evaluation and refinement: Microfinance institutions should regularly evaluate and refine their fraud detection systems to adapt to evolving fraud techniques. This includes monitoring system performance, reviewing identified fraud cases, and incorporating customer feedback to enhance system accuracy and efficiency.
- Ethical considerations: It is crucial for microfinance institutions to address ethical considerations in AI-driven fraud detection. This involves mitigating algorithmic bias, ensuring fairness in decision making, and fostering transparency to build trust with customers and stakeholders.
In summary, the future of AI-driven fraud detection in microfinance holds immense potential. Through advancements in advanced biometric authentication, natural language processing, and blockchain technology, microfinance institutions can further strengthen their fraud detection capabilities, enhance data security, and foster trust among customers and stakeholders. It is essential for microfinance institutions to embrace these future directions and recommendations to ensure the continued evolution and effectiveness of their fraud detection systems.
Intelligence of Machine Coupled with Human Understanding
As microfinance institutions embrace the power of artificial intelligence (AI) and machine learning (ML) in their operations, it is essential to ensure that these technologies are utilized in a way that aligns with the core purpose of microfinance – to empower individuals and promote inclusivity. The intelligent capabilities of machines, coupled with human understanding, can create a highly efficient and inclusive loan collection system.
Machine learning models can analyze borrower insights and identify patterns that can help predict delinquency. By leveraging these insights, microfinance institutions can take timely preventive measures to support borrowers facing financial challenges. However, it is crucial to strike a balance between precision analysis from machines and a humane approach that considers the unique circumstances of each borrower.
Implementing an inclusive collection system requires a multi-faceted approach. The data-driven insights provided by AI and ML can inform decision-making, but human empathy and understanding must also play a significant role. Microfinance institutions should consider the specific needs and circumstances of their borrowers, taking into account socioeconomic factors and potential challenges that may hinder their repayment capabilities.
By combining the intelligence of machines with human understanding, microfinance institutions can create a loan collection system that not only focuses on financial sustainability but also fosters inclusivity and supports individuals in their journey towards economic empowerment.
Importance of Human-Centric Approach in AI-Driven Collections
While AI and ML offer powerful tools for analyzing data and predicting outcomes, a human-centric approach remains crucial in the context of microfinance. Machine-driven decisions can enhance operational efficiency and reduce biases; however, they may not fully capture the intricate nuances of individual circumstances. Therefore, microfinance institutions must rely on their human expertise to interpret the results generated by AI algorithms and tailor their approach accordingly.
Understanding the underlying reasons for loan delinquency requires empathy and a holistic view of borrowers’ lives. AI can provide valuable insights, such as identifying patterns related to delinquency risk factors. However, building trust and maintaining positive customer relationships requires human interaction and a genuine interest in the well-being of borrowers. By combining the intelligence of machines with human expertise, microfinance institutions can strike the right balance between efficiency and empathy, ensuring that borrowers receive the support they need to overcome financial challenges.
Benefits of Intelligence of Machine Coupled with Human Understanding | Challenges of Intelligence of Machine Coupled with Human Understanding |
---|---|
|
|
Conclusion
In conclusion, the evolution of AI technologies in microfinance institutions is revolutionizing the sector, particularly in the realm of fraud detection. By harnessing the power of machine learning, AI-driven fraud detection systems offer a range of benefits, including improved accuracy, operational efficiency, and customer satisfaction.
However, it is crucial to address challenges such as algorithmic bias and false positives to ensure fairness and customer trust. Ongoing research and development are necessary to mitigate biases and refine fraud detection systems.
Looking ahead, advancements in biometric authentication, natural language processing, and blockchain technology hold great promise for enhancing security and transparency in microfinance. The growing adoption of AI technologies in this domain indicates a bright future for the industry.
Ultimately, the success of AI in microfinance hinges on striking a balance between the power of machine learning and the human understanding required to support and empower individuals. With careful consideration and continuous evaluation, AI technologies can truly transform the microfinance sector, making it more inclusive and efficient.
FAQ
What is the role of AI in microfinance institutions?
AI plays a crucial role in fraud detection and prevention in microfinance institutions. It utilizes advanced algorithms and machine learning to analyze data and identify potential fraudulent activities.
What are the benefits of AI-driven fraud detection systems in microfinance institutions?
Implementing AI-driven fraud detection systems offers benefits such as quick identification and response to fraud, enhanced operational efficiency, and improved customer satisfaction.
How do AI-driven fraud detection systems work?
AI systems utilize real-time data analytics and monitoring to detect and respond to fraud in a timely manner. They analyze transactions, identify patterns, and assess the likelihood of fraudulent activities using predictive modeling and risk scoring.
How can AI enhance security and trust in microfinance institutions?
AI technologies strengthen fraud prevention measures, enhance customer identification and authentication processes, and provide transparent explanations of fraud detection algorithms, which build trust with customers and stakeholders.
What are the challenges and ethical considerations in AI-driven fraud detection?
Addressing algorithmic bias and minimizing false positives in fraud detection systems are crucial challenges. Microfinance institutions should invest in ongoing research and development to ensure fairness and transparency in their fraud detection processes.
What are the future directions and recommendations for AI in microfinance?
The future of AI in microfinance includes advancements in biometric authentication methods, natural language processing for fraud detection in textual data, and the application of blockchain technology for enhanced data security and transparency. Strong partnerships and continuous evaluation of fraud detection systems are recommended.
How can the intelligence of machine learning be coupled with human understanding in microfinance?
Machine learning models can assist microfinance institutions in creating a highly efficient and inclusive loan collection system. By analyzing borrower insights and predicting delinquency, these models enable timely preventive measures while considering the core purpose of microfinance – to enable and empower individuals.
What is the significance of AI technologies in the evolution of microfinance institutions?
AI-driven fraud detection systems improve fraud detection, enhance security, and enable more informed decision-making. These technologies reduce financial losses, improve efficiency, and strengthen customer trust in microfinance institutions.
Source Links
- https://insidebigdata.com/2023/06/01/ai-empowers-microfinance-revolutionizing-fraud-detection/
- https://www.cloud4c.com/blogs/machine-learning-a-more-intelligent-route-to-microfinance-collection
- https://www.irjet.net/archives/V8/i11/IRJET-V8I1191.pdf