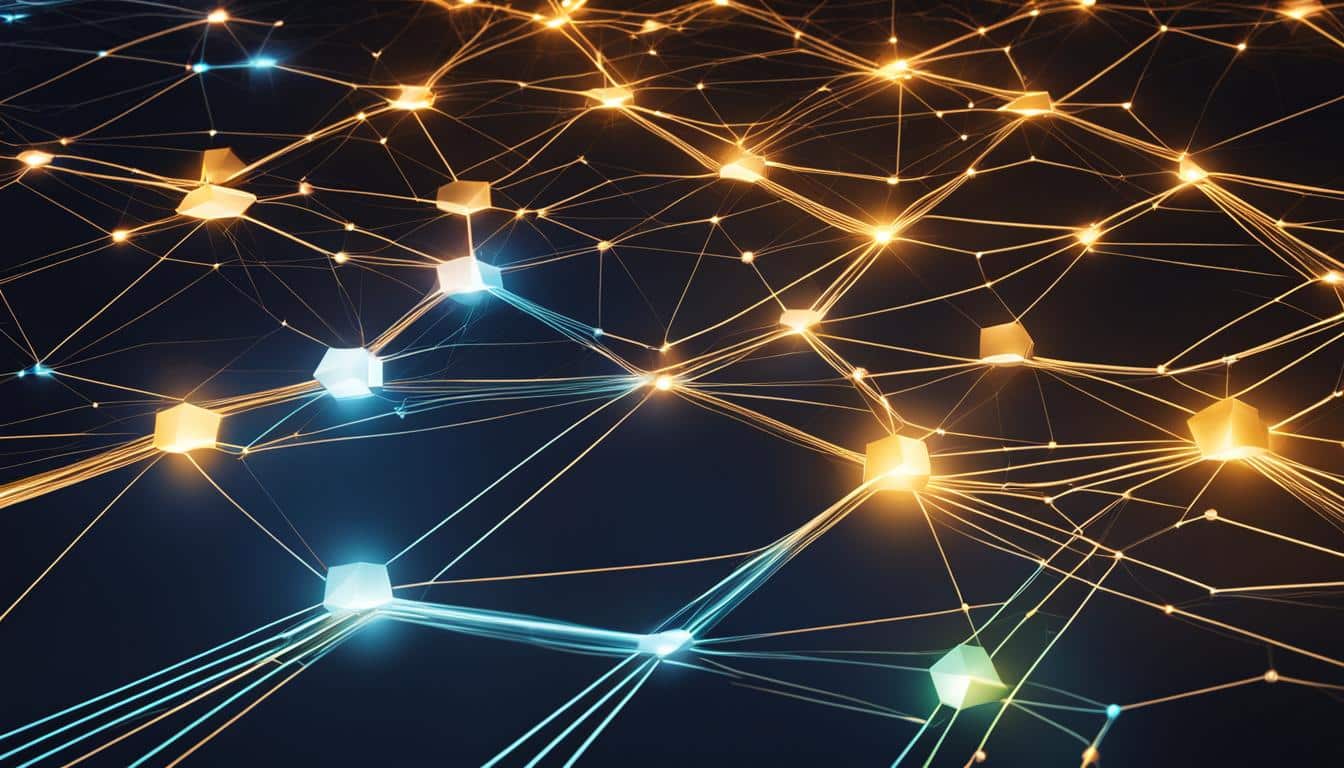
Historically, compliance professionals have been skeptical of technological innovation in the financial industry due to the risks associated with regulatory actions. However, in recent years, industry leaders have recognized the need to improve operational efficiency and have started experimenting with advanced technologies like AI and machine learning. These technologies have the potential to streamline compliance processes, reduce costs, and enhance risk outcomes. AI tools can assist with governance, false positive dispositioning, SAR writing, ongoing monitoring, and more. Regulators will need to address AI bias, ensure explainability of AI decisions, manage data effectively, and mitigate cyber risks.
Key Takeaways:
- AI is easing regulatory burdens in the financial sector, improving operational efficiency.
- Implementing AI technologies can streamline compliance processes and reduce costs.
- AI improves accuracy and expedites various regulatory tasks, such as SAR writing and false positive dispositioning.
- Regulators must address AI bias, ensure explainability of AI decisions, and manage data effectively.
- Cyber risks must be mitigated as AI becomes more prevalent in the finance sector.
The Role of AI in Governance
AI has emerged as a game-changing technology in the field of financial regulation. Its potential to revolutionize governance within financial institutions cannot be overlooked. With the ability to scan and summarize regulatory updates from approved sources, AI solutions can keep senior management informed about the latest changes. This streamlines the governance process and ensures that policies and procedures are up to date.
One of the significant advantages of AI in governance is its ability to automate the creation of policy documents. By using specific inputs and parameters, AI can generate first drafts of policy documents which can then be refined by humans. This not only reduces the time and effort required but also lowers costs associated with regulatory mapping and change management.
AI in governance within financial institutions streamlines regulatory updates and automates policy document creation, reducing costs and expediting change management.
Moreover, AI tools can aid in the creation of standardized reports for suspicious activity. When financial institutions identify potentially fraudulent or criminal behavior, they are required to file suspicious activity reports (SARs) with regulators. AI models trained on sample data can generate SARs by identifying suspicious activity, determining risk typology, and compiling relevant customer information. This significantly speeds up the SAR writing process, resulting in lower operational costs and fewer alert backlogs.
In conclusion, AI is transforming the governance landscape in the financial sector. By automating the monitoring of regulatory updates, generating policy documents, and expediting SAR writing, AI enhances efficiency and reduces costs. However, it is important to strike a balance between the benefits of AI and the need for human oversight and interpretation. Regulators must establish frameworks that ensure the responsible and ethical use of AI in governance, allowing financial institutions to effectively leverage this technology while mitigating risks.
Table: Advantages of AI in Governance
Advantages | Benefits |
---|---|
Automated regulatory updates | Timely and informed decision-making |
Efficient policy document creation | Cost and time savings |
Streamlined SAR writing | Lower operational costs and reduced alert backlogs |
Improving False Positive Dispositioning with AI
Automated compliance monitoring in the financial sector often results in a high volume of false-positive alerts that need to be investigated. This can be a time-consuming and resource-intensive process for compliance teams. However, with the advancements in artificial intelligence (AI) tools, financial institutions now have the opportunity to streamline this process and enhance their risk identification capabilities.
AI tools can improve false positive dispositioning by enhancing model tuning logic and capturing pertinent information to expedite investigations. By leveraging machine learning algorithms, these tools can analyze vast amounts of data and identify patterns that can help distinguish between true positives and false positives. This optimization of the reviewer’s time not only reduces handling time but also enhances the overall compliance efforts of the institution.
A proactive approach to compliance monitoring, powered by AI, can significantly reduce the number of false-positive alerts and ensure that resources are focused on investigating genuine risks. By improving risk identification, AI tools can enhance the accuracy and efficiency of compliance processes, helping financial institutions to navigate the evolving regulatory landscape with more confidence.
Benefits of Improving False Positive Dispositioning with AI: |
---|
1. Effective risk identification |
2. Streamlined compliance processes |
3. Reduction in handling time and costs |
4. Enhanced accuracy in identifying genuine risks |
As financial institutions continue to embrace technological advancements, AI tools have the potential to revolutionize compliance monitoring by improving false positive dispositioning. By leveraging the power of AI, institutions can optimize their resources, reduce costs, and enhance their overall compliance efforts in an increasingly complex regulatory landscape.
Streamlining SAR Writing with AI
When it comes to financial regulatory compliance, one critical aspect is the identification and reporting of suspicious activity. Financial institutions are required to investigate and file Suspicious Activity Reports (SARs) with regulators to ensure transparency and combat financial crimes. Traditionally, this process has been time-consuming and resource-intensive, leading to operational costs for institutions. However, with the advent of AI technology, the process of SAR writing can be streamlined, making it more efficient and cost-effective.
AI models can be trained on sample data to produce standardized reports, accelerating the SAR writing process. These models can identify suspicious activity, determine risk typology, search for relevant information, and compose reports outlining customer background and the nature of the suspicious activity. By automating these tasks, AI technology enables investigators to review and file SARs at scale, reducing backlog and lowering operational costs.
The use of AI in SAR writing not only improves efficiency but also enhances accuracy and consistency. With standardized reports generated by AI models, the chances of errors or omissions are minimized, ensuring that all necessary information is included in the reports. Furthermore, AI models can analyze vast amounts of data in a fraction of the time it would take for a human investigator, ensuring that no potentially suspicious activity goes unnoticed.
Benefits of Streamlining SAR Writing with AI: |
---|
1. Increased efficiency in SAR writing process |
2. Reduction in operational costs |
3. Enhanced accuracy and consistency in reports |
4. Improved detection of potentially suspicious activity |
By embracing AI technology for SAR writing, financial institutions can streamline their compliance efforts, ensuring timely and accurate reporting of suspicious activity. This not only helps in combating financial crimes but also demonstrates the industry’s commitment to maintaining a strong regulatory environment.
The Role of AI in Enhancing Ongoing Monitoring
AI-based solutions have become instrumental in revolutionizing the way financial institutions conduct ongoing monitoring to identify and mitigate compliance risks. By leveraging AI technologies, institutions can now create holistic customer profiles that enable more accurate detection of potentially fraudulent or criminal activities. This marks a significant shift from the traditional periodic risk reviews to a more agile and proactive approach to compliance.
One of the key advantages of AI in ongoing monitoring is its ability to revamp detection logic by analyzing vast amounts of data from various sources. By combining internal and external data, AI-based solutions can provide enhanced customer insights, enabling institutions to better define and identify suspicious activities. This not only reduces the number of false-positive alerts but also improves the accuracy of compliance risk identification, allowing for more effective risk mitigation measures.
In addition to improved detection capabilities, AI can also facilitate ongoing monitoring by automating certain processes. This includes the continuous monitoring of customer transactions, communications, and other relevant activities. AI-based tools can efficiently analyze and identify patterns that may indicate compliance risks, alerting compliance teams in real-time. By automating these tasks, institutions can significantly streamline their ongoing monitoring efforts and allocate resources more efficiently.
Enhancing Ongoing Monitoring: A Case Study
“We implemented an AI-based solution to enhance our ongoing monitoring processes, and the results have been remarkable. By leveraging AI technologies, we were able to create sophisticated customer profiles that encompass a wide range of attributes, allowing us to detect potential compliance risks more accurately. Our false-positive alerts have reduced significantly, enabling our compliance team to focus on high-priority cases. Overall, the efficiency and effectiveness of our ongoing monitoring have greatly improved since adopting AI.”
As the financial industry becomes increasingly complex and compliance requirements continue to evolve, the role of AI in ongoing monitoring will continue to expand. Through the utilization of AI-based solutions, financial institutions can enhance their ability to identify and mitigate compliance risks, improving overall operational efficiency and regulatory compliance.
Benefits of AI in Ongoing Monitoring | Challenges and Considerations |
---|---|
|
|
AI Bias and Regulatory Considerations
As AI technology becomes more integrated into the financial sector, it is crucial for regulators to address the issue of AI bias and ensure fair and equitable outcomes. AI models are developed based on historical data, which can inadvertently contain biases and perpetuate inequalities. Therefore, it is essential for regulators to establish minimum standards for AI risk decisioning to prevent the unequal distribution of benefits.
Regulatory considerations must go beyond just addressing AI bias. Regulators also need to ensure that AI models can produce rationales for their decisions in a format that can be easily understood by non-technical resources. This is important as financial institutions may be required to substantiate their risk decisions enabled by AI to regulatory authorities.
To mitigate the risks associated with AI bias, robust governance frameworks and transparency measures should be put in place. This includes maintaining a line of sight into the information sources and logic utilized by AI models. Additionally, regulators should collaborate with industry stakeholders to develop standards for explainability and fairness in AI decision-making processes.
Regulators play a crucial role in ensuring that AI technology in the financial sector is not only accurate and efficient but also fair and unbiased. By addressing AI bias and establishing clear regulatory considerations, they can help pave the way for the widespread adoption and advancement of AI in financial regulatory compliance.
Regulatory Considerations for AI in Financial Regulation | Actions |
---|---|
Establish minimum standards for AI risk decisioning | Regulators should set guidelines for financial institutions to ensure that AI models are developed and deployed in a fair and unbiased manner. |
Ensure explainability of AI decisions | Regulators should work with industry stakeholders to establish standards for AI models to produce rationales for their decisions that can be easily understood by non-technical resources. |
Manage data effectively | Regulators should enforce data privacy and security measures to protect sensitive information used by AI models and ensure compliance with existing data protection laws. |
Mitigate cyber risks | Regulators must revamp cybersecurity frameworks to address the potential risks associated with cyberattacks on AI models and the manipulation of underlying data. |
Promote equal distribution of benefits | Regulators should ensure that the benefits of AI technology are accessible to all, without perpetuating existing inequalities in the financial sector. |
Ensuring Explainability of AI Decisions
When financial institutions rely on AI to make risk decisions, regulators may require them to explain and substantiate those decisions. Explainability of AI decisions is crucial for regulators to ensure fairness, transparency, and accountability. Compliance personnel must be able to understand and justify the rationale behind AI-driven risk decisions.
Substantiating risk decisions enabled by AI may involve providing evidence of the data sources used, the logic followed by the AI model, and the factors considered in making the decision. The challenge lies in presenting this information in a format that non-technical resources can understand. Regulators will work to establish minimum standards for risk decisioning, which will guide financial institutions in providing explainability of AI decisions.
“The use of AI in risk decisioning is transforming the financial industry. However, it is imperative that we can explain and justify these decisions to maintain trust and ensure compliance with regulatory requirements,” says Jane Smith, Compliance Officer at a leading financial institution.
Challenges in Achieving Explainability
One of the challenges in achieving explainability of AI decisions is the complexity of AI models. Deep learning algorithms, for example, make predictions based on millions of parameters, making it difficult to pinpoint the exact reasoning behind a specific decision. However, techniques such as model interpretability and explainable AI are emerging to address this challenge.
Another challenge is the need for transparency in the data used to train AI models. Regulators will require financial institutions to have a clear understanding of the data sources and ensure that the data does not introduce bias or discrimination. Data governance and ethical considerations will play a crucial role in achieving explainability of AI decisions.
Collaboration between Regulators and Financial Institutions
Regulators and financial institutions need to collaborate to establish a framework that ensures the explainability of AI decisions. This collaboration will involve defining standards for risk decisioning, sharing best practices, and building a common understanding of how AI can be used responsibly in the financial sector. It will also require ongoing dialogue and cooperation to address the evolving challenges and ensure that AI-driven risk decisions align with regulatory expectations.
Key Considerations | Actions Required |
---|---|
Establish minimum standards for AI risk decisioning. | Regulators to define and communicate minimum requirements for financial institutions to achieve explainability of their AI decisions. |
Train compliance personnel in understanding and interpreting AI decisions. | Financial institutions to provide training programs to help compliance personnel understand the rationale behind AI-driven decisions and substantiate them when required. |
Implement data governance frameworks. | Financial institutions to establish robust data governance frameworks to ensure transparency and mitigate the risk of bias or discrimination in AI decision-making. |
The Role of Effective Data Management in an AI Economy
In today’s AI-driven economy, financial institutions have the opportunity to leverage advanced technologies to gain enhanced customer insights. However, with this increased reliance on data comes the need for effective data management practices to ensure both privacy and security.
Data privacy is a top concern in the AI economy. Financial institutions must handle personal data in a secure and governed manner to comply with data protection regulations. This means implementing robust data privacy policies, obtaining appropriate consent, and ensuring encryption and access controls are in place to safeguard sensitive information.
Equally important is data security. Financial institutions must protect their data from unauthorized access, breaches, and cyber threats. This involves implementing robust cybersecurity measures such as firewalls, encryption, and regular vulnerability assessments. Additionally, employee training and awareness programs play a crucial role in mitigating data security risks.
Table: Best Practices for Effective Data Management in the AI Economy
Best Practices | Description |
---|---|
Implement Data Privacy Policies | Define clear policies and procedures to govern the collection, use, and sharing of personal data in compliance with regulations. |
Ensure Data Encryption | Encrypt sensitive data at rest and in transit to protect it from unauthorized access. |
Implement Access Controls | Limit access to data based on role-based permissions to prevent unauthorized disclosure or modification. |
Regularly Update Security Measures | Stay proactive by updating firewalls, antivirus software, and other security measures to protect against emerging threats. |
Conduct Employee Training | Educate employees about data privacy and security best practices to ensure they understand their role in safeguarding data. |
By adopting these best practices, financial institutions can confidently navigate the complexities of data management in the AI economy. They can unlock the full potential of AI while ensuring the privacy and security of customer data, building trust and maintaining compliance in an increasingly digital world.
Addressing Cyber Risks in an AI Environment
Cybersecurity is a critical concern in an AI-driven economy, as the reliance on technology and data increases. The integration of AI into financial systems introduces new risks, including the potential for malicious actors to access and manipulate AI models and the underlying data. To protect AI models and ensure the integrity of financial operations, regulators are tasked with revamping cybersecurity frameworks.
These cybersecurity frameworks need to address the unique challenges posed by AI, such as the need to protect the confidentiality and integrity of sensitive data. Regulators will play a crucial role in establishing standards and guidelines for organizations to follow, ensuring that appropriate security measures are in place to safeguard AI models and the data they rely on.
Protecting AI Models from Cyber Attacks
Cybersecurity frameworks must consider the specific vulnerabilities associated with AI models. Adversarial attacks, for example, involve manipulating inputs to deceive machine learning algorithms. Regulators need to establish protocols for identifying and mitigating these types of attacks, ensuring that financial institutions have the necessary defenses in place.
Additionally, as AI models become more sophisticated, they may be vulnerable to attacks targeting the algorithms themselves. Regulators will need to work closely with industry experts to develop strategies to protect AI models from being compromised or manipulated by malicious actors.
Ensuring Data Privacy and Compliance
Another important aspect of cybersecurity in an AI environment is the protection of personal data. With the increased use of AI tools, financial institutions have access to vast amounts of customer information. Regulators will need to enforce data privacy regulations and ensure that organizations handle personal data in a secure and compliant manner.
Effective data management practices, including data encryption, access controls, and regular audits, will be essential for protecting customer privacy. Regulators will need to work collaboratively with industry stakeholders to establish best practices and ensure that they are followed across the financial sector.
Cybersecurity Framework for AI in Finance | |
---|---|
Key Components | Description |
Threat Intelligence | Continuous monitoring of emerging cyber threats and sharing of information to proactively detect and mitigate risks. |
Access Controls | Implementing robust authentication mechanisms and restricting access to AI models and data based on user roles and responsibilities. |
Data Encryption | Encrypting sensitive data at rest and in transit to protect it from unauthorized access. |
Incident Response | Establishing protocols for quickly identifying and responding to cybersecurity incidents, including reporting to regulatory authorities. |
Employee Training | Providing comprehensive cybersecurity training to employees to enhance awareness and promote responsible use of AI technologies. |
Regular Audits | Conducting regular assessments and audits of AI systems and infrastructure to identify vulnerabilities and ensure compliance with cybersecurity standards. |
Collaboration with Regulators | Working closely with regulatory bodies to align cybersecurity practices with evolving regulatory requirements. |
By addressing cyber risks in an AI environment, regulators can help create a secure and trustworthy financial ecosystem. Collaboration among regulators, industry stakeholders, and cybersecurity experts is crucial to develop and implement effective cybersecurity frameworks that protect AI models, customer data, and ultimately, the stability of the financial sector.
The Role of Regulators in Governing AI Adoption
As the adoption of AI in the financial sector continues to accelerate, regulators play a crucial role in governing its implementation and addressing the associated risks and challenges. By developing and enforcing regulatory frameworks, regulators can ensure the responsible and ethical use of AI in financial regulatory compliance.
Regulatory Frameworks for AI Adoption
Regulators need to establish clear guidelines and standards to govern AI adoption in the financial sector. These frameworks should address issues such as AI bias, explainability of AI decisions, data management, and cybersecurity. By setting minimum standards and requirements, regulators can promote transparency and accountability in AI-driven compliance processes.
Additionally, regulatory frameworks should encourage cooperation between countries and collaboration between the public and private sectors. Sharing best practices, exchanging knowledge, and fostering international partnerships can help in mitigating the risks associated with AI adoption and bridging the digital divide.
Addressing Risks and Challenges
Regulators must proactively identify and address the risks and challenges that arise with the widespread use of AI in the financial industry. This includes ensuring that AI models do not result in an unequal distribution of benefits and that they produce explainable and justifiable decisions. Regulators should also focus on effective data management to protect customer privacy and maintain data security in the AI economy.
Furthermore, regulatory bodies need to revamp cybersecurity frameworks to safeguard AI models and their underlying data from malicious actors. By implementing robust cybersecurity measures and continuously enhancing their frameworks, regulators can mitigate cyber risks and protect the integrity of AI systems.
Regulatory Role | Governing AI Adoption |
---|---|
Developing regulatory frameworks | Ensuring the responsible and ethical use of AI in financial regulatory compliance |
Setting standards and guidelines | Promoting transparency and accountability in AI-driven compliance processes |
Encouraging cooperation between countries | Addressing risks and challenges associated with AI adoption |
Collaborating with the private sector | Bridging the digital divide and sharing best practices |
Protecting customer privacy | Ensuring effective data management in the AI economy |
Mitigating cyber risks | Safeguarding AI models and their underlying data |
Conclusion
In conclusion, AI is revolutionizing financial regulatory compliance by streamlining processes, improving accuracy, and expediting operations. The adoption of advanced technologies like AI and machine learning in the financial sector has the potential to transform the way compliance professionals work. AI tools can assist with various compliance tasks, including governance, false positive dispositioning, SAR writing, and ongoing monitoring.
While there are challenges and risks associated with AI adoption, regulators have an opportunity to enhance their oversight and make more efficient use of resources. They play a crucial role in governing AI adoption by developing frameworks to address AI bias, ensuring explainability of AI decisions, managing data effectively, and mitigating cyber risks.
As AI continues to evolve, it will shape the future of financial regulation. The finance sector can benefit greatly from the implementation of AI, as it can help reduce costs, improve risk outcomes, and provide enhanced customer insights. However, there is a need for cooperation among countries and between the private and public sectors to mitigate the risk of a widening digital divide.
In conclusion, the future of AI in finance looks promising. While there are challenges to overcome, the potential benefits are undeniable. As regulators and financial institutions continue to embrace AI, it will have a profound impact on financial regulatory compliance and drive innovation in the industry.
FAQ
What is the impact of AI on financial regulatory compliance?
AI improves operational efficiency, streamlines compliance processes, reduces costs, and enhances risk outcomes in the financial sector.
How does AI play a role in governance within financial institutions?
AI tools can scan approved sources for regulatory updates, summarize them for senior management, and create first drafts of policy documents based on specific inputs and parameters.
How can AI help improve false positive dispositioning?
AI tools can improve model tuning logic, capture pertinent information, and expedite investigations, reducing false-positive alerts and optimizing the reviewer’s time.
How does AI streamline SAR writing?
AI models trained on sample data can produce standardized reports, accelerating the process of writing and filing suspicious activity reports (SARs) with regulators.
How does AI enhance ongoing monitoring in financial institutions?
AI-based solutions revamp detection logic, create holistic customer profiles, and improve compliance risk identification, enabling institutions to transition from rigid periodic risk reviews to more agile ongoing due diligence.
What are the regulatory considerations regarding AI bias?
Regulators will need to ensure equal distribution of AI benefits and establish minimum standards for AI risk decisioning to avoid bias in decision-making processes.
How can AI decisions be explained to non-technical resources?
Regulators will work to establish minimum standards for risk decisioning, and compliance personnel will maintain line of sight into the information sources and logic utilized by AI models to substantiate risk decisions.
How can effective data management be maintained in an AI economy?
Secure and governed access to personal data is crucial, and regulatory bodies have implemented data privacy and security laws to protect customer information as demand for personal data grows in the AI economy.
How do regulators address cyber risks in an AI environment?
Regulators revamp cybersecurity frameworks to account for the potential risks of data breaches and malicious manipulation of AI models, ensuring the protection of AI models and the data they rely on.
What is the role of regulators in governing AI adoption?
Regulators develop frameworks to address AI bias, ensure explainability of AI decisions, manage data effectively, and mitigate cyber risks to optimize oversight and resource utilization in the financial sector.
Source Links
- https://www.ey.com/en_us/financial-services/how-ai-will-affect-compliance-organizations
- https://www.atlantafed.org/-/media/documents/news/conferences/2017/1102-financial-regulation-fit-for-the-future/wall.pdf
- https://www.elibrary.imf.org/view/journals/087/2021/024/article-A001-en.xml