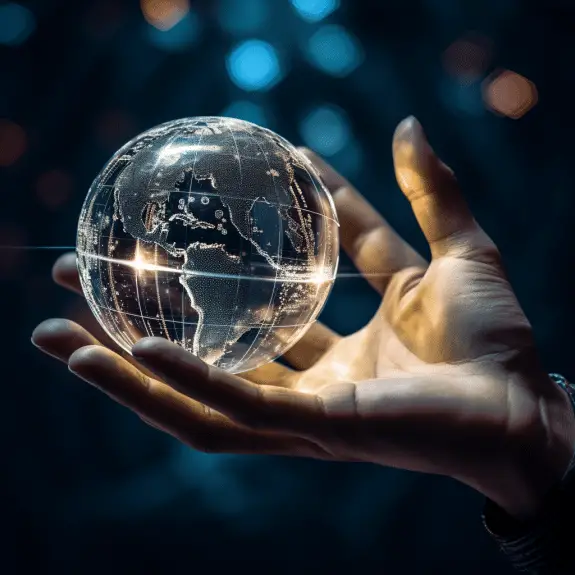
Transforming Finance This paper discusses the impact of the rapid adoption of artificial intelligence (AI) and machine learning (ML) in the financial sector, highlighting the benefits and concerns associated with these technologies. It emphasizes the need for prudential oversight and regulatory approaches to mitigate risks and ensure financial stability. The paper also addresses the potential for AI to deepen the digital divide between advanced and developing economies, highlighting the importance of cooperation and investment in developing economies. Finally, it discusses the unique risks and challenges that AI adoption in the financial sector presents, such as explainability, bias, and cybersecurity concerns.
Key Takeaways
- AI and ML systems have made significant advancements in the financial sector, improving client experiences and risk management.
- The adoption of AI/ML in the financial sector brings benefits but also concerns, such as explainability and bias.
- Developing economies can benefit from AI adoption but require investment in digital infrastructure and supportive business environments.
- Policy challenges in AI adoption include transparency, privacy, and cyber risks, requiring regulatory approaches to ensure financial stability.
- Decision-making on AI policy in the public sector is characterized by great uncertainty, requiring flexible and adaptive strategies.
The Evolution of AI and ML Systems
In recent years, there have been significant advancements in the development of artificial intelligence (AI) and machine learning (ML) systems.
AI systems can do tasks that require human intelligence. The financial sector likes AI and ML systems, using big data and cloud computing. These systems make client experiences better, processes faster, and risk management and compliance better. AI helps financial institutions give better services, like personalized investment advice and easier borrowing. AI also saves money for financial institutions. Central banks and regulators can also use AI to watch for risk and be better at oversight.
Advancements in AI and ML Systems in the Financial Sector | Benefits | Examples |
---|---|---|
Enhanced client experiences | Improved customer satisfaction and personalized services | Automated communication, personalized investment advice |
Operational efficiency | Cost savings, streamlined processes | Automated loan approvals, enhanced identity verification |
Risk management and regulatory compliance | Better risk assessment and regulatory reporting | AI-driven predictive analytics, automated regulatory compliance checks |
The Impact of AI in the Financial Sector
The adoption of AI/ML in the financial sector has brought about significant transformations. AI/ML systems are reshaping client experiences by providing automated communication, investment advice, borrowing processes, and identity verification. These systems also improve operational efficiency, cost savings, and risk management for financial institutions. Additionally, AI/ML systems offer new tools for central banks and regulatory authorities to enhance systemic risk surveillance and strengthen prudential oversight. The potential value of AI in the banking sector is estimated to reach $1 trillion.
In the financial sector, AI/ML systems have revolutionized the way financial institutions interact with clients. These systems are capable of automating communication processes, providing personalized investment advice, streamlining borrowing processes, and ensuring secure identity verification. Clients can now access financial services anytime and anywhere through AI-powered virtual assistants and chatbots, which offer prompt and accurate responses to their queries and concerns.
Financial institutions also benefit from the adoption of AI/ML systems. These technologies enable operational efficiency by automating repetitive and time-consuming tasks, such as data entry and analysis. AI-powered algorithms can quickly process vast amounts of financial data and generate insights, enabling financial institutions to make informed decisions and mitigate risks. Moreover, AI/ML systems improve cost savings by reducing manual labor and increasing productivity.
Benefits of AI/ML in the Financial Sector | Challenges of AI/ML in the Financial Sector |
---|---|
|
|
The Digital Divide and AI Adoption
The adoption of artificial intelligence (AI) and machine learning (ML) technologies has seen significant growth in advanced economies and a few emerging markets. However, this rapid adoption has the potential to widen the digital divide between advanced and developing economies. While advanced economies have embraced AI, developing economies are often left behind in terms of access and utilization of these technologies.
The digital divide poses challenges for developing economies, but it also presents opportunities. Through AI adoption, developing economies can enhance access to credit, reduce credit risk assessments, and improve financial inclusion. Bridging the digital divide requires a comprehensive approach, including the development of a digital-friendly policy framework, investment in digital infrastructure, supportive business environments, and capacity building in AI.
Cooperation among countries and between the private and public sectors is crucial in mitigating the risk of a widening digital divide and enabling knowledge transfer in developing economies. By working together, we can bridge the gap, foster inclusive growth, and ensure that the benefits of AI are shared by all.
The Potential Benefits of AI Adoption in Developing Economies
AI adoption in developing economies can bring numerous benefits, including:
- Enhanced access to credit for individuals and businesses
- Reduced credit risk assessments, enabling more efficient lending processes
- Improved financial inclusion and access to financial services for underserved populations
These benefits can contribute to economic growth, poverty reduction, and the overall development of these economies. However, to realize these benefits, developing economies must overcome the challenges posed by the digital divide and create an enabling environment for AI adoption.
Strategies for Bridging the Digital Divide
To bridge the digital divide and promote inclusive AI adoption, developing economies should consider implementing the following strategies:
- Develop a digital-friendly policy framework that encourages investment in AI and fosters innovation
- Invest in digital infrastructure, including affordable and reliable internet connectivity
- Create supportive business environments that encourage entrepreneurship and the development of AI technologies
- Build capacity and skills in AI through educational programs and vocational training
- Promote cooperation among countries and between the private and public sectors to share knowledge and resources
By implementing these strategies, developing economies can overcome the challenges of the digital divide and leverage AI technologies to drive economic growth and development.
Challenges | Solutions |
---|---|
Limited access to AI technologies | Invest in digital infrastructure and promote technology transfer |
Lack of AI skills and expertise | Develop educational programs and vocational training |
High implementation costs | Create supportive business environments and provide financial incentives |
Policy Challenges and Regulatory Approaches
The adoption of artificial intelligence (AI) in the financial sector poses unique policy challenges and requires robust regulatory approaches.
AI and ML algorithms are becoming more complex and autonomous. It’s important to have transparency and explainability to avoid biases and ensure accountability. Policymakers must address cybersecurity threats and consumer privacy risks associated with AI adoption. Regulatory frameworks need to keep up with advancements in AI to oversee its implementation in finance.
The lack of transparency and explainability in AI/ML-based decisions is a major policy challenge in finance. It’s crucial to understand the algorithms behind these decisions to ensure fairness and non-discrimination. Policymakers should work with industry experts to develop regulatory approaches that require clear explanations and address any biases that may arise.
Regulatory Approaches to Mitigate Risks
To effectively mitigate the risks associated with AI adoption in the financial sector, regulators should consider a combination of proactive and reactive approaches. Proactive approaches include the development of specific guidelines and standards for AI implementation, as well as the establishment of independent regulatory bodies with expertise in AI technology. These bodies can conduct audits, assess the fairness and transparency of AI systems, and enforce compliance with regulatory requirements.
Reactive approaches involve the establishment of mechanisms for monitoring and detecting potential risks and addressing any emerging issues. This can include ongoing assessments of AI systems’ performance, regular reporting requirements for financial institutions using AI, and the establishment of channels for reporting AI-related concerns. By combining proactive and reactive approaches, regulators can create a comprehensive regulatory framework that addresses the unique challenges posed by AI adoption in the financial sector.
Policy Challenges | Regulatory Approaches |
---|---|
Lack of transparency and explainability in AI/ML-based decisions | Establishing regulatory requirements for clear explanations of AI-driven decisions and addressing potential biases |
Cybersecurity threats and data breaches | Developing robust cybersecurity protocols and standards to protect consumer data |
Ethical implications of AI and unauthorized access to sensitive data | Addressing ethical considerations and establishing regulatory frameworks to ensure data privacy and prevent unauthorized access |
Uncertainty in AI Policy Decision-making
AI policy decisions are difficult due to uncertainty. AI is constantly changing and understanding its impact is challenging. Policymakers struggle to make effective decisions that consider all risks and benefits. A key challenge is the lack of understanding of the technology. More research is needed to comprehend AI’s capabilities and risks. This uncertainty can lead to overly cautious or permissive policies, which could hinder innovation or have unintended consequences.
In addition to the lack of knowledge, there are also inherent uncertainties in the development and evolution of AI itself. The technology is constantly changing and advancing, making it difficult to predict how it will develop in the future. This uncertainty adds another layer of complexity to policy decision-making, as policymakers must consider not only the current state of AI but also its potential future developments and the impact they may have.
policy decision-making
Challenges in AI Policy Decision-making | Strategies to Mitigate Uncertainty |
---|---|
Lack of thorough understanding of AI technology and its implications | Incorporate elements from the argumentative approach in decision theory |
Inherent uncertainties in the development and evolution of AI | Cooperation among stakeholders and investment in digital infrastructure |
Potential unintended consequences of policy decisions | Flexibility and adaptability in decision-making |
To address the uncertainties in AI policy decision-making, decision-makers can employ various strategies. One approach is to incorporate elements from the argumentative approach in decision theory. This involves analyzing normative issues and considering a pluralistic perspective to better understand and address the uncertainties involved in AI policy decisions. By engaging in robust discussions and considering multiple viewpoints, decision-makers can make more informed and well-rounded decisions.
AI policy decision-making
Cooperation among stakeholders is another crucial strategy in mitigating uncertainty. By collaborating with experts, industry leaders, and other relevant parties, decision-makers can access a wealth of knowledge and expertise that can help inform their decisions. Additionally, investment in digital infrastructure, such as data collection and analysis capabilities, can provide decision-makers with the tools they need to gather and analyze information, reducing uncertainty and improving the quality of their decisions.
Finally, decision-makers need to be flexible and adaptive in their approach to AI policy decision-making. They should be prepared to adjust their strategies and policies as new information becomes available and as the technology continues to evolve. This flexibility will allow decision-makers to respond effectively to emerging challenges and take advantage of new opportunities that AI presents.
Strategies to Mitigate Uncertainty in AI Policy
Uncertainty is inherent in AI policy decision-making, given the rapidly evolving nature of technology and the complexity of its implications. To navigate this uncertainty, decision-makers can employ various strategies that promote informed and effective decision-making.
1. Embracing the argumentative approach:
The argumentative approach in decision theory focuses on analyzing normative issues and incorporating diverse perspectives. Decision-makers can engage in robust discussions, seeking input from experts, stakeholders, and the public to better understand the uncertainties and complexities involved in AI policy decisions. This approach fosters dialogue, transparency, and democratic legitimacy in decision-making processes.
2. Temporal strategies:
AI policy decision-making should be flexible and adaptive, recognizing that the landscape of AI technologies and their impacts can change rapidly. Decision-makers should adopt temporal strategies that allow for ongoing monitoring, evaluation, and adjustment of policies as new information emerges. This approach ensures that policies remain relevant and effective in addressing the uncertainties associated with AI.
3. Investment in knowledge transfer and cooperation:
To mitigate uncertainty, decision-makers should prioritize investments in knowledge transfer and cooperation among stakeholders. Collaborative efforts between governments, academia, industry, and civil society can enhance understanding, promote best practices, and facilitate the exchange of information and expertise. By working together, decision-makers can navigate the uncertainties of AI policy more effectively.
In summary, mitigating uncertainty in AI policy decision-making requires a multi-faceted approach that incorporates the argumentative approach, temporal strategies, and collaborative efforts. By embracing these strategies, decision-makers can navigate the complexities of AI policy, ensure transparency and democratic legitimacy, and make informed decisions that maximize the benefits while mitigating the risks of AI technologies.
The Role of Democracy in AI Policy
When it comes to AI policy for public use, upholding democratic principles is of utmost importance.
AI should be used cautiously in public functions to guarantee transparency, privacy, safety, and fairness.
“As decision-makers, we must prioritize the principles of democracy when making decisions regarding AI for public use, considering the potential societal impacts and ensuring the highest level of democratic legitimacy in the decision-making process.”
When using AI in public, decision-makers should prioritize democratic principles, assess potential societal effects, and ensure the decision-making process is highly democratic.
Furthermore, the argumentative approach, which emphasizes democratic decision-making and legitimacy, is highly relevant in the context of AI policy. This approach encourages open dialogue, constructive debate, and the exploration of various ethical and social implications of AI adoption. By engaging in robust discussions and considering different viewpoints, decision-makers can make more informed and well-rounded decisions that align with democratic values.
Benefits of Democracy in AI Policy | Challenges in Implementing Democratic AI Policy |
---|---|
|
|
The Concept of Uncertainty in Decision-Making
Decision-making, across various fields like social science, economics, philosophy, and psychology, is marked by the presence of uncertainty. It is essential to acknowledge the limitations of available information and the intricacies of the decision context when making choices under uncertainty. Great uncertainty refers to decision problems where multiple uncertain circumstances prevail, making the decision-making process more challenging and complex.
“Decision-making is a complex process that involves analyzing risks, exploring potential outcomes, and accounting for uncertainties. It requires a thorough understanding of the context and an ability to adapt to changing circumstances. Acknowledging and addressing uncertainty is crucial for effective decision-making.”
Dimensions of Uncertainty | Definition |
---|---|
Ontological Uncertainty | The inherent unpredictability and complexity of the external environment |
Epistemic Uncertainty | The limitations in knowledge and the inability to fully comprehend the implications of a decision |
Uncertainty in AI Policy Decision-making
When it comes to making policy decisions regarding artificial intelligence (AI), decision-makers are faced with great uncertainty. The rapid evolution of AI technology, coupled with the lack of in-depth understanding of its impacts, creates a complex and unpredictable decision-making landscape. Traditional decision theory is often insufficient for addressing the broad and intractable uncertainties associated with AI policy.
Failure to correctly identify and address uncertainties in AI policy decision-making can lead to ineffective policies and missed opportunities. To navigate this uncertainty, decision-makers should incorporate strategies informed by the argumentative approach, which involves analyzing normative issues from multiple perspectives and considering the pluralistic nature of AI policy decisions. Temporal strategies and careful consideration of framing are also valuable tools in addressing uncertainty in AI policy.
Strategies for Mitigating Uncertainty in AI Policy
AI policy decision-makers can use different strategies to make informed decisions. One such strategy is the argumentative approach, which involves analyzing normative issues and considering various perspectives. This approach is useful in dealing with the complexities and uncertainties associated with AI policy.
By incorporating this approach, decision-makers can better understand the potential implications of AI policy and make well-informed decisions.
Temporal strategies also play a crucial role in mitigating uncertainty. Decision-makers need to be flexible and adaptive, particularly in an environment where technology is rapidly evolving. By developing strategies that account for future changes, decision-makers can navigate uncertainty with more confidence and agility.
Framing is another important consideration in addressing uncertainty in AI policy. Decision-makers must be aware of the potential biases and implications associated with different frames of reference. By carefully framing the issues at hand, decision-makers can ensure transparency, fairness, and effective communication of their policy decisions.
Conclusion
In today’s rapidly evolving world, navigating economic uncertainties requires a modern approach that harnesses the power of artificial intelligence (AI).
AI and machine learning have transformed the financial sector by improving client experiences, automating processes, and enhancing risk management. However, there are unique risks and challenges that need to be addressed.
Prudential oversight and regulatory approaches are essential to mitigate the risks associated with AI adoption in finance. Policymakers must prioritize transparency and robustness of AI algorithms to protect against potential biases and cybersecurity concerns. Cooperation and investment in developing countries are also crucial for inclusive AI adoption.
Decision-making on AI policy is challenging due to uncertainties surrounding the technology. Decision-makers must be flexible, adaptive, and well-informed. By incorporating elements from argumentative approach in decision theory and considering temporal strategies, policymakers can make more informed and effective decisions.
FAQ
What is the impact of AI and ML in the financial sector?
AI and ML systems have transformed client experiences, automated processes, and improved risk management and regulatory compliance in the financial sector.
How has the COVID-19 pandemic influenced the adoption of AI/ML in the financial sector?
The pandemic has further accelerated the adoption of AI/ML in the financial sector, with banks exploring ways to leverage these technologies for customer relationship management and risk management.
How can developing economies benefit from the adoption of AI?
Developing economies can benefit from AI adoption, including enhanced access to credit and reduced credit risk assessments. Bridging the digital divide requires developing a digital-friendly policy framework and investing in infrastructure, supportive business environments, skills, and risk management frameworks.
What are the unique risks and challenges associated with AI adoption in the financial sector?
AI/ML-based decisions made by financial institutions may lack transparency and could potentially be biased. Additionally, AI/ML adoption brings new cyber risks and privacy concerns.
How can policymakers mitigate risks and ensure the integrity and stability of the financial system?
Policymakers need to strengthen prudential oversight and regulatory approaches to address the robustness of AI/ML algorithms, potential structural shifts, and reliance on few AI/ML service providers.
How can decision-makers address uncertainty in AI policy decision-making?
Decision-makers can utilize strategies informed by the argumentative approach, incorporate elements from the argumentative approach in decision theory, and be flexible and adaptive to future changes.
What factors should decision-makers prioritize when implementing AI for public use?
Decision-makers should prioritize transparency, privacy, safety, and fairness when implementing AI for functions central to the public sphere.
What is uncertainty in decision-making, and how does it relate to AI policy?
Uncertainty in decision-making refers to multiple uncertain circumstances that make the decision-making process more challenging. AI policy decision-making involves great uncertainty due to the novelty of the technology and lack of thorough understanding of its impacts.
How can decision-makers mitigate uncertainty in AI policy decision-making?
Decision-makers can employ temporal strategies, incorporate elements from the argumentative approach in decision theory, and prioritize cooperation, knowledge transfer, and investment in digital infrastructure.
Source Links
- https://www.elibrary.imf.org/view/journals/087/2021/024/article-A001-en.xml
- https://aima.cs.berkeley.edu/contents.html
- https://link.springer.com/article/10.1007/s00146-021-01263-4