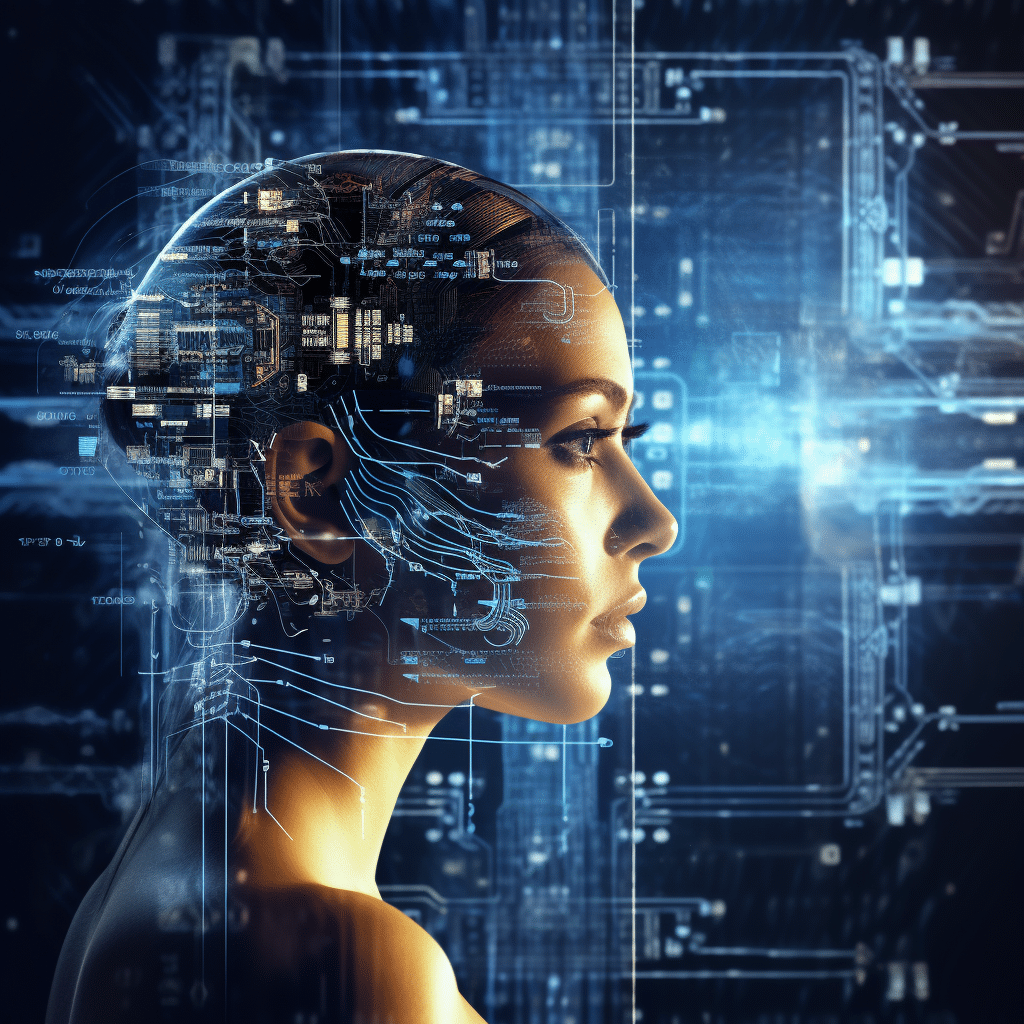
The Gigantic Data Challenge: Managing AI-Generated Insights
AI algorithms excel at extracting valuable insights from unstructured and structured data, enabling businesses to uncover patterns, trends, and correlations that were previously hidden. However, this abundance of AI-generated insights presents new hurdles that organizations must overcome.
The Scale of the Challenge
The massive scale of AI-generated data poses several difficulties. Firstly, storing such vast amounts of information requires significant computational resources and robust infrastructure capable of handling the volume, velocity, and variety of data. Organizations must invest in scalable storage solutions and cloud technologies to accommodate this surge in data.
Secondly, processing and analyzing petabytes of data in real-time demands sophisticated data pipelines, where AI models can rapidly preprocess, analyze, and generate insights. These pipelines need to be efficient, reliable, and scalable to handle the complexities of AI-generated data.
“The success of managing AI-generated insights lies in establishing a strong data governance framework.”
Lastly, managing the quality and accuracy of AI-generated insights is crucial. Although AI models excel at pattern recognition, biases or irrelevant data can skew the accuracy of the insights generated. Organizations need to regularly validate AI models, ensure proper training data, and detect and mitigate any inherent bias.
Data Governance and Ethical Consideration
With the colossal amounts of data being generated, organizations must prioritize data governance to ensure compliance, privacy, and ethical use of AI-generated insights. Implementing effective policies, procedures, and controls is essential to prevent misuse, unauthorized access, or breaches of sensitive information.
Additionally, ethical considerations are paramount. Transparency in AI decision-making processes is critical, ensuring that the insights generated align with organizational values and legal obligations. Human oversight and responsibility play a vital role in ensuring AI-generated insights are not used inappropriately or reinforce biases.
The Future of Managing AI-Generated Insights
Meeting the challenges posed by AI-generated insights requires a comprehensive approach involving technology, policies, and talent. Organizations need to invest in cutting-edge infrastructure and technologies that can handle the volume and complexities of data generated by AI algorithms.
-
- Developing robust data governance frameworks with clear policies and procedures for data management, security, and ethics.
-
- Establishing multidisciplinary teams consisting of data scientists, AI experts, and domain specialists to validate and interpret AI-generated insights.
-
- Implementing ongoing monitoring and auditing mechanisms to ensure compliance and detect any biases or inaccuracies.
-
- Promoting a culture of data literacy and responsible AI usage within the organization.
The potential for AI-generated insights to revolutionize industries and drive innovation is immense. However, managing this tremendous influx of data is a significant challenge. By addressing the scale, governance, and ethical considerations associated with AI-generated insights, organizations can unlock the full potential of their data-driven endeavors.
What strategies and tools are available to ensure the quality and accuracy of AI-generated insights for informed decision-making
When it comes to ensuring the quality and accuracy of AI-generated insights for informed decision-making, several strategies and tools can be employed:
1. Data Quality Checks:
Implement rigorous data quality checks to identify and rectify any errors, inconsistencies, or biases in the data being fed into the AI models. This may involve data cleaning, preprocessing, and normalization techniques.
2. Algorithm Selection and Validation:
Choose appropriate AI algorithms based on the specific use case and requirements. Validate these algorithms against known benchmarks or ground truth data to assess their accuracy and performance.
3. Model Training and Testing:
Train AI models on high-quality and diverse datasets to ensure they can handle a wide range of scenarios. Conduct thorough testing to validate the models’ accuracy and generalizability.
4. Data Governance and Ethics:
Establish robust data governance practices to ensure compliance with ethical guidelines and regulations. This includes maintaining data privacy, protecting against biases, and addressing potential ethical concerns.
5. Human-in-the-loop Approach:
Incorporate human feedback and supervision throughout the AI development process. This involves human experts validating and interpreting the AI-generated insights, providing corrections or additional context when necessary.
6. Explainable AI Techniques:
Employ techniques that enhance the transparency and interpretability of AI models. This helps users and stakeholders comprehend how the insights are generated, making it easier to identify any potential issues or limitations.
7. Continuous Monitoring and Improvement:
Continuously monitor the performance of AI models in real-world applications. Regularly update and refine the models based on feedback and new data to ensure ongoing accuracy and relevancy of insights.
8. External Auditing:
Engage third-party auditors or independent experts to evaluate the AI systems, methodologies, and outputs. External auditing can provide objective assessments and recommendations for improvement.
9. Benchmarking and Comparison:
Compare AI-generated insights with existing domain knowledge or other trusted sources to evaluate their accuracy and identify any discrepancies or biases.
10. Feedback Loop:
Encourage users and stakeholders to provide feedback on the AI-generated insights and incorporate their input into the development process. This iterative feedback loop helps improve the accuracy and relevance of the insights over time.
Overall, a combination of these strategies and tools can help ensure the quality and accuracy of AI-generated insights, enabling informed decision-making.
What are the key challenges in extracting actionable insights from AI-generated data, and how can they be addressed?
There are several key challenges in extracting actionable insights from AI-generated data:
1. Data quality and bias:
AI models heavily rely on the quality and diversity of training data. If the data used to train the models is incomplete, biased, or of poor quality, it can lead to inaccurate or unreliable insights. To address this, it is important to ensure the data collected is representative, clean, and verified for bias.
2. Interpretability and explainability:
AI models often operate as black boxes, making it difficult to understand the underlying reasons behind their predictions or decisions. This lack of interpretability can hinder the extraction of actionable insights. Techniques such as model explainability algorithms, visualizations, or the use of transparent models like decision trees can help address this challenge.
3. Scalability and complexity:
As the volume and complexity of AI-generated data increase, it becomes challenging to analyze and extract valuable insights in a timely manner. Implementing scalable infrastructure, leveraging cloud computing, and utilizing distributed computing frameworks can help address this challenge.
4. Contextual understanding:
AI-generated data might lack context, making it harder to derive actionable insights. It is crucial to combine AI-generated data with domain expertise and contextual knowledge to properly interpret and extract meaningful insights.
5. Data privacy and ethical considerations:
AI-generated data may contain sensitive or personal information. Addressing privacy concerns and ensuring ethical use of data is vital. Implementing strong data protection measures, complying with regulatory requirements, and adopting responsible AI practices can help mitigate these challenges.
6. Continuous learning and adaptation:
AI models need to evolve and adapt as new data becomes available. Building systems that can continuously learn, update models in real-time, and adapt to changing conditions can enhance the extraction of actionable insights from AI-generated data.
Overall, addressing these challenges requires a combination of technical solutions, domain expertise, and ethical considerations. It is crucial to invest in data collection, annotation, and curation processes, employ interpretable and transparent AI models, foster collaboration between domain experts and AI practitioners, and adhere to privacy and ethical guidelines.
How can organizations effectively manage and make sense of the massive volumes of data generated by AI algorithms?
Managing and making sense of the massive volumes of data generated by AI algorithms requires a strategic and systematic approach. Here are some steps organizations can take to effectively handle this data:
1. Define a Data Management Strategy:
Organizations need to establish a clear strategy that outlines how data will be collected, stored, and managed. This includes defining data governance policies, data quality standards, and data retention rules.
2. Implement Robust Data Infrastructure:
Organizations should invest in scalable and flexible data infrastructure that can handle large volumes of data. This can include cloud-based storage solutions, big data platforms, and distributed computing systems.
3. Data Pre-processing and Cleaning:
Prior to analysis, organizations should conduct data pre-processing to remove noise, handle missing values, and standardize formats. This ensures that the data is clean and ready for analysis.
4. Use Advanced Analytics Tools:
Leveraging advanced analytics tools like data visualization, data mining, and machine learning can help organizations make sense of the data. These tools enable the identification of patterns, correlations, and trends within the data.
5. Employ Data Governance Practices:
Establishing data governance practices ensures data quality, consistency, and security. It involves setting up data access controls, defining data ownership, and regularly auditing and monitoring data usage.
6. Enhance Data Security:
Given the sensitive nature of AI-generated data, organizations must prioritize data security. Implementing robust security measures, including encryption, access controls, and regular backups, can help protect against data breaches.
7. Foster Cross-functional Collaboration:
Effective data management requires collaboration between different teams within an organization. IT, data science, operations, and business teams should work together to share insights, identify data requirements, and align on data-driven objectives.
8. Regularly Monitor and Evaluate:
Organizations should establish processes to continually monitor and evaluate data management efforts. This includes tracking key performance indicators, analyzing the effectiveness of algorithms, and making necessary adjustments to improve data processing and decision-making.
By adopting these steps, organizations can successfully manage and derive value from the massive volumes of data generated by AI algorithms.
Thank you for your sharing. I am worried that I lack creative ideas. It is your article that makes me full of hope. Thank you. But, I have a question, can you help me?