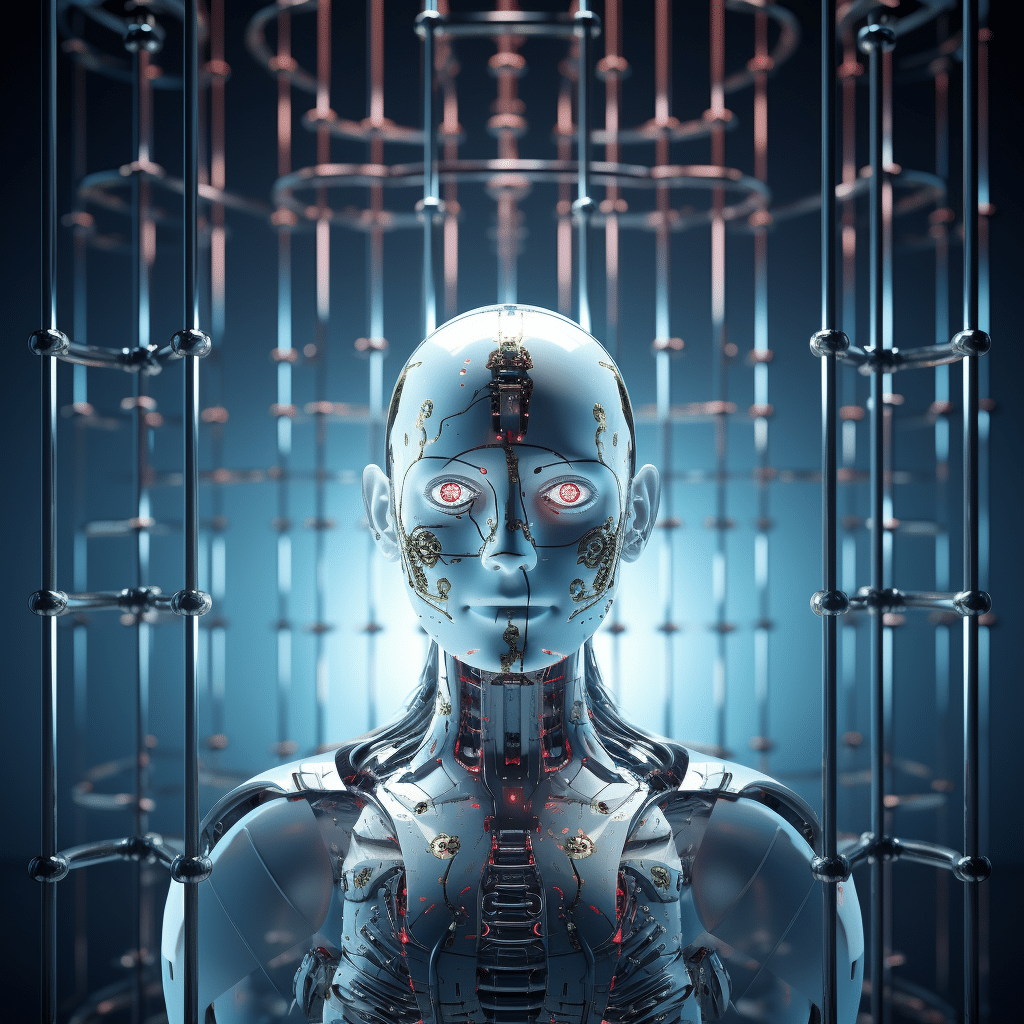
AI and Data Privacy: Striking a Balance for User Protection
Artificial Intelligence (AI) has become an integral part of our interconnected digital world,
revolutionizing industries and transforming the way we live and work. From personalized recommendations to voice assistants, AI algorithms are constantly evolving to provide smarter and more efficient services. However, with these advancements come concerns about data privacy. AI systems heavily rely on user data, extracting insights and patterns from vast amounts of information to enhance their capabilities. This raises questions about how companies can strike a balance between utilizing AI to improve user experiences while safeguarding their privacy and ensuring data protection.
The Importance of User Privacy
Protecting user privacy is essential in the age of AI. Users should have control over their personal data and be assured that it is handled securely. While AI can bring tremendous benefits, it also holds the potential for misuse or unauthorized access to sensitive information. Companies need to establish robust privacy policies and transparent data practices to build trust with their users. Implementing encryption protocols, anonymizing data, and obtaining explicit consent for data usage are key aspects to address when navigating the AI landscape.
Strategies for Balancing AI and Privacy
Striking a balance between AI advancements and data privacy requires a multi-faceted approach. Here are some strategies to ensure user protection while utilizing the power of AI:
- Privacy-By-Design: Incorporating privacy measures as a fundamental element from the inception of AI systems can minimize privacy risks. Building privacy considerations into the development process helps avoid potential pitfalls.
- Strong Data Governance: Establishing robust data governance frameworks assists in managing and securing user data. Ensuring compliance with privacy regulations, implementing strict access controls, and regular audits can help protect against unauthorized access and ensure privacy best practices.
- Enhanced Data Transparency: Providing clear and concise explanations of how user data is collected, used, and shared instills confidence and empowers users. Transparent privacy policies and intuitive data
management dashboards contribute to a sense of control over personal information. - User-Focused Permissions: Implementing granular permission models allows users to have fine-grained control over their data. Providing choice and flexibility in granting permissions strengthens user trust and ensures that AI systems operate within acceptable privacy boundaries.
A Collaborative Approach
Protecting data privacy while benefiting from AI requires collaboration between technology companies, policymakers, and users. Companies must prioritize user concerns and demonstrate their commitment to privacy. Policymakers should establish clear guidelines and regulations to ensure responsible AI use, and users need to understand the potential privacy implications and make informed choices.
By striking a balance between AI and data privacy, we can unlock the full potential of AI while maintaining trust and respect for user rights. It is crucial to ensure that AI continues to enhance our lives without compromising our privacy.
Author: John Doe
Published on: Month Day, Year
What are some ethical considerations that arise when balancing user protection and AI utilization for data analysis?
When balancing user protection and AI utilization for data analysis, there are several ethical considerations that arise:
1. Privacy:
The collection and analysis of user data can intrude upon privacy rights. It is important to inform users about the data being collected, the purpose of its use, and seek their consent. User data should be anonymized and stored securely to prevent unauthorized access or misuse.
2. Informed Consent:
Users should have a clear understanding of how their data is being used and for what purposes. They should have the ability to opt-in or opt-out of data collection and analysis, and their choices should be respected.
3. Transparency:
The algorithms and models used for data analysis should be transparent and explainable. Users should have clarity on how their data is being processed and used to make decisions that may affect them. This allows for accountability and helps prevent bias or discrimination.
4. Bias and Fairness:
AI can inherit biases from the data it is trained on or from the algorithms used. Ethical considerations demand that steps be taken to identify and mitigate biases to ensure fairness and prevent discrimination.
5. Security:
Safeguarding user data from unauthorized access, breaches, or misuse is crucial. AI systems must be built with robust security measures to protect user information.
6. Accountability and Oversight:
Clear responsibility and accountability should be established for the use of AI in data analysis. Mechanisms for auditing and regular assessments should be in place to ensure compliance with ethical guidelines and prevent misuse.
7. Data Quality and Accuracy:
The data used for analysis should be accurate, reliable, and representative. Ensuring data quality is important to prevent misleading or incorrect conclusions.
8. Intellectual Property:
Ownership and protection of user data must be clearly defined, and appropriate intellectual property rights must be respected.
9. Consent withdrawal and deletion:
Users should have the right to withdraw their consent and request the deletion of their data. Protocols must be in place to handle such requests promptly and effectively.
10. Benefit and Harm Assessment:
AI utilization for data analysis should be assessed for potential benefits and harms to individuals and society. The balance between user protection and AI utilization should aim to maximize benefits while minimizing harm.
It is important for organizations and developers to consider these ethical considerations and implement appropriate measures to ensure the responsible and ethical use of AI in data analysis.
How can organizations ensure the privacy of user data while leveraging AI technologies?
Organizations can ensure the privacy of user data while leveraging AI technologies by implementing the following practices:
1. Data anonymization
Remove or encrypt personally identifiable information (PII) from the data used for AI training and analysis, ensuring that individual identities cannot be linked to the data.
2. Consent and transparency
Obtain explicit consent from users for collecting and using their data for AI purposes. Clearly communicate to users how their data will be used, what insights or services AI will provide, and allow them to manage their privacy preferences.
3. Data minimization
Collect and retain only the necessary data required for AI applications, minimizing the amount of personal data that needs to be stored or processed.
4. Secure data storage and processing
Implement robust security measures to protect user data throughout its lifecycle. This includes encryption, access controls, and regular security audits to ensure data integrity and prevent unauthorized access.
5. Federated learning
Instead of transferring user data to a central server, employ federated learning techniques where the AI models are trained locally on user devices, preserving data privacy while still benefiting from aggregate insights.
6. Differential privacy
Apply techniques such as differential privacy to add noise or randomness to data, ensuring that individual user information cannot be inferred from the collected data.
7. Regular audits and compliance
Conduct regular audits to assess data handling practices, ensure compliance with privacy regulations (such as GDPR or CCPA), and rectify any potential vulnerabilities or privacy breaches.
8. Ethical AI practices
Establish guidelines and frameworks to ensure ethical AI practices, promoting fairness, accountability, and transparency in decision-making processes.
9. User-controlled data sharing
Give users control over their data by allowing them to easily access, modify, or delete their personal information. Provide options for users to customize data sharing settings based on their preferences.
10. Continuous monitoring and improvement
Continuously monitor AI systems and data handling practices to identify and address evolving privacy risks. Regularly update privacy policies and practices according to emerging standards and evolving regulations.
What are the risks and challenges associated with data privacy in the context of AI implementation?
Data privacy is a critical concern in the context of AI implementation due to the following risks and challenges:
1. Data Breaches
AI systems rely heavily on vast amounts of data, and any data breach or unauthorized access could potentially expose sensitive information to malicious actors. This poses a significant risk to individuals’ privacy, as personal data may be misused or sold for illicit purposes.
2. Data Misuse
Inaccurate or biased AI algorithms, driven by inadequate or inappropriate data, can lead to discriminatory outcomes and privacy violations. The misuse of personal data in AI algorithms may result in unfair targeting, profiling, or manipulation of individuals, infringing on their privacy rights.
3. Informed Consent
Obtaining informed consent for data collection and processing can be challenging in AI systems, as it is not always practical or feasible to explain the complex algorithms and potential implications to users. The lack of transparency and meaningful consent may undermine individuals’ privacy rights.
4. Inference Attacks
AI algorithms can infer sensitive information that was not explicitly shared. For example, by analyzing patterns in a person’s behavior, AI systems may deduce their political affiliations, sexual orientation, or health conditions, breaching their privacy without their consent.
5. Data Linkability
Merging different datasets can increase the risk of reidentification and linkability, allowing actors to combine supposedly anonymized data with other sources to de-anonymize individuals. This linkage jeopardizes privacy safeguards, potentially revealing sensitive information.
6. Lack of Regulations
The rapid advancement of AI technology often surpasses existing legislation and regulations. Insufficient legal frameworks to govern AI may result in a lack of accountability and proper protection for individuals’ privacy, leaving them vulnerable to potential abuses.
7. Data Access and Control
Data collected and used by AI systems may be stored and processed in multiple locations and shared among various entities. This lack of control over personal data raises concerns about who has access to the information and whether individuals have the ability to manage and delete their data effectively.
Addressing these risks and challenges requires the development of robust privacy frameworks, enhanced data protection practices, and clear guidelines for AI systems to ensure data privacy is prioritized throughout the AI implementation process.