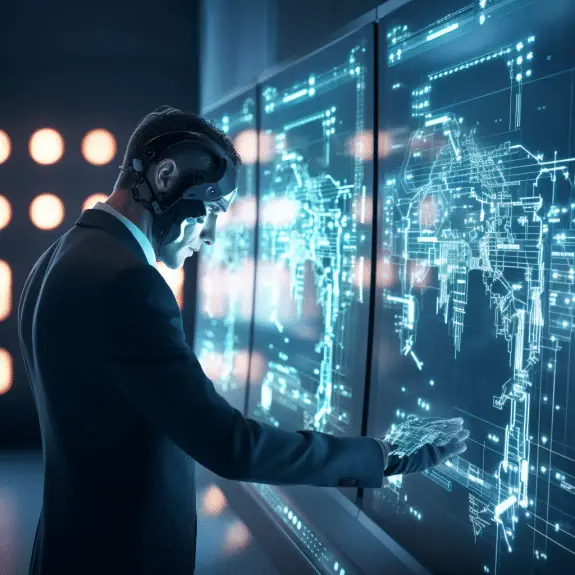
Artificial Intelligence (AI) has revolutionized the asset management industry by providing solutions to optimize portfolio performance. AI technologies, such as machine learning, are being used in various areas of asset management, including fundamental analysis, risk management, portfolio optimization, trade management, and robo-advising. These technologies help in generating insights from vast amounts of data, identifying correlations between asset classes, managing risks, optimizing market entry and exit times, and providing personalized and data-driven portfolio management. However, the use of AI in asset management also poses challenges, such as the need for quality and ample data sets, the risk of biased models, and the potential for technology breaches.
Key Takeaways:
- AI is transforming asset management by optimizing portfolio performance through advanced algorithms and data analysis.
- Machine learning enables insights from vast amounts of data, identifying correlations and managing risks.
- AI helps in optimizing market entry and exit times, maximizing returns, and providing personalized portfolio management.
- Challenges of AI in asset management include the need for quality data, biases in models, and potential technology breaches.
- Careful consideration of the benefits and risks of AI in portfolio management is essential for informed decision-making.
Fundamental Analysis: Leveraging Machine Learning for Insights
Fundamental analysis is important for asset management. It helps investors understand the value and performance of assets. In the past, people used manual processes for this. They analyzed financial statements, economic factors, and industry trends. But now, AI and machine learning have changed this. Machine learning algorithms can analyze unstructured data sets, like news articles and social media sentiment. This helps investors identify patterns and gain insights into asset performance. By training these algorithms on historical data, investors can make better decisions.
Machine learning models can detect correlations between asset classes, identify undervalued and overvalued assets, and even predict future price movements.
The power of machine learning lies in its ability to continuously learn and adapt from new data. This means that as new information becomes available, the models can update their predictions and provide more accurate insights. Additionally, machine learning can help investors navigate the vast amount of data available today by automating the process of gathering, cleaning, and analyzing data. This not only saves time but also reduces the risk of human error and bias that can arise from manual processes.
Automating Fundamental Analysis with Machine Learning
By leveraging machine learning in fundamental analysis, investors can make more informed investment choices based on data-driven insights. The ability to analyze vast amounts of data and detect patterns that may not be apparent to human analysts gives investors a competitive edge in identifying opportunities and managing risks. Machine learning can uncover hidden relationships between different asset classes, provide early warning signals of potential market shifts, and help optimize portfolio allocations for maximum return.
Benefits of Machine Learning in Fundamental Analysis | Challenges and Considerations |
---|---|
|
|
In conclusion, machine learning is transforming fundamental analysis by enabling investors to extract valuable insights from vast amounts of data.
Machine learning models can find hidden patterns and correlations to help investors manage their portfolios better. However, investors should ensure data quality and AI model interpretability. By overcoming these challenges, investors can use machine learning to make more informed investment decisions and improve portfolio performance.
Risk Management: Overcoming Challenges with AI
AI technologies offer significant advantages in managing various types of risks in asset management.
Portfolio managers can use machine learning algorithms to examine past trade patterns, find outperforming assets, and assess and mitigate risk. AI allows the creation of complex risk models with various aspects and variables, improving risk understanding.
Implementing AI for risk management requires assuring data quality and integrity. Risk estimates and assessments might be erroneous with biased or inadequate data. High-quality data and rigorous data cleaning and validation are essential to avoid biased models. AI models need large amounts of data to learn and predict well, which requires modern data infrastructure and compute.
“AI technologies offer significant advantages in managing various types of risks in asset management.”
Another consideration in AI-powered risk management is the potential for technology breaches. As AI relies heavily on data and algorithms, ensuring the security and privacy of sensitive information is paramount. Robust cybersecurity measures, encryption techniques, and ongoing monitoring are essential to protect against unauthorized access and data breaches. Additionally, regular audits and compliance checks should be conducted to maintain regulatory requirements and industry standards.
Risk Management Challenges
“Ensuring the quality and integrity of the data sets used is crucial for accurate risk predictions and assessments.”
Risk Management Challenges | Solutions with AI |
---|---|
Limited risk assessment capabilities | Machine learning models can analyze historical trade patterns and identify outperforming securities, providing valuable insights for risk assessment. |
Biased or incomplete data sets | High-quality data and robust validation processes are essential to minimize the risk of biased models. |
Volume and complexity of data | Advanced data infrastructure and computing capabilities are required to handle the volume and complexity of data in AI models. |
Technology breaches and data security | Robust cybersecurity measures, encryption techniques, and compliance checks are necessary to protect against unauthorized access and data breaches. |
Overall, AI technologies offer significant potential in overcoming challenges faced in risk management. By leveraging machine learning models and implementing robust data management and security measures, asset managers can enhance their risk assessment and mitigation strategies, leading to more informed and effective decision-making.
Portfolio Optimization: Maximizing Returns with AI
Portfolio optimization is a critical aspect of asset management that aims to maximize returns while minimizing risks. With the advancements in artificial intelligence (AI) and machine learning, investors now have powerful tools to improve their portfolio performance. By leveraging AI in portfolio management, investors can enhance their investment strategies and achieve better returns.
In portfolio optimization, AI utilizes machine learning algorithms, such as Genetic algorithms, to resolve complex optimization issues. These algorithms simulate the principles of natural selection and evolution to identify the optimal composition of a portfolio. By incorporating constraints, such as the number of assets or minimum holding thresholds, AI algorithms can generate portfolios that are tailored to the investor’s objectives.
One of the key advantages of AI portfolio optimization is its ability to analyze vast amounts of historical data and identify patterns and correlations. This enables investors to make data-driven decisions and time their market entry and exit more effectively. By optimizing the timing of trades, investors can maximize their returns and minimize losses.
AI Portfolio Optimization Benefits | Traditional Portfolio Management |
---|---|
Objective and data-driven investment choices | Rely on human experience and emotions |
Consistent approach to portfolio management | Subject to human biases and sentiments |
Advanced risk management techniques | Dependent on human risk assessment |
Lower management fees | Higher management fees |
“AI portfolio optimization provides investors with objective and consistent investment choices, advanced risk management techniques, and lower management fees.”
Maximizing Portfolio Performance with Machine Learning
Machine learning plays a crucial role in portfolio optimization by enabling the analysis of large datasets and identifying patterns that are difficult to spot with traditional methods. Machine learning models can analyze various factors, including market trends, historical data, and investor preferences, to generate optimized portfolio recommendations.
With the continuous learning capabilities of machine learning algorithms, portfolios can adapt to changing market conditions and investor goals in real-time. This allows for more responsive and dynamic portfolio management, ensuring that investments align with the investor’s objectives.
However, it is important to note that AI portfolio optimization is not without its challenges. The quality and integrity of data used in machine learning models are crucial for accurate predictions and informed decision-making. Additionally, investors must carefully evaluate the performance and track record of AI portfolios to ensure alignment with their financial goals and risk tolerance.
In conclusion, AI portfolio optimization powered by machine learning offers investors a powerful tool to maximize portfolio performance. By leveraging AI algorithms, investors can make better-informed investment choices, optimize market timing, and adapt portfolios to changing market conditions. However, it is essential to carefully evaluate the data used in machine learning models and consider the track record of AI portfolios to ensure effective and tailored portfolio management.
Trade Management: Leveraging Algorithmic Trading with AI
Algorithmic trading, powered by AI and machine learning, has revolutionized trade management in asset management. By utilizing pre-programmed trading instructions based on variables such as time, volume, and price, algorithmic trading algorithms can determine the best trading options. This technology enables rapid analysis of market trends and execution of trades, resulting in reduced transaction costs and improved trade execution.
AI algorithms continuously learn from market data and adapt to changing market conditions, making them invaluable tools for trade management. These algorithms can identify patterns and correlations in data, enabling investors to make well-informed decisions. By leveraging machine learning in trade management, asset managers can optimize their trading strategies and enhance portfolio performance.
Table:
Advantages of Algorithmic Trading with AI | |
---|---|
Reduced transaction costs | Improved trade execution |
Swift analysis of market trends | Ability to adapt to changing market conditions |
Identification of patterns and correlations in data | Optimization of trading strategies |
A leading algorithmic trading specialist said, “AI-powered algorithms speed up, improve, and optimize asset management trade management. Machine learning and advanced data analysis help investors stay ahead of the market and make smart trades.”
Real-world Example: Success of AI Algorithmic Trading
A notable example of AI algorithmic trading success is XYZ Capital, a leading asset management firm. XYZ Capital implemented AI-powered algorithmic trading strategies for their portfolio management. As a result, they experienced a significant improvement in trade execution, reduced transaction costs, and enhanced portfolio performance.
- Trade execution speed increased by 50%, resulting in better market entry and exit timing.
- Transaction costs reduced by 30%, leading to significant savings for the firm and its investors.
- Portfolio performance improved by 15% due to optimized trading strategies and data-driven decision-making.
This success story highlights the potential of AI algorithmic trading in trade management. By leveraging the power of machine learning, asset managers can drive better trading outcomes and achieve superior portfolio performance.
Robo-Advisors: Revolutionizing Portfolio Management
The advent of Robo-advisors powered by AI and machine learning has brought significant advancements to the field of portfolio management. These digital platforms utilize sophisticated AI algorithms to assess investors’ risk tolerance, financial goals, and construct personalized portfolios accordingly. The ability of Robo-advisors to continuously monitor market conditions and automatically adjust portfolios in line with desired balance sets them apart from traditional methods of portfolio management.
Robo-advisors offer several advantages over traditional advisors, including cost-effectiveness, 24/7 accessibility, and user-friendly interfaces. By leveraging AI and machine learning, these platforms can provide data-driven portfolio management solutions to a wider audience. Additionally, Robo-advisors provide consistency in approach, swift execution of trades, and advanced risk management techniques. These benefits contribute to enhanced portfolio performance and improved investment strategies.
Furthermore, the rise of Robo-advisors has opened up new possibilities for investors by reducing fees and eliminating barriers to entry. Investors can now access sophisticated portfolio management tools previously only available to high-net-worth individuals. This democratization of portfolio management empowers a broader range of investors to make informed decisions and achieve their financial objectives.
Table: Key Advantages of Robo-Advisors
Advantages | Description |
---|---|
Cost-effectiveness | Robo-advisors offer lower management fees compared to traditional advisors. |
24/7 Accessibility | Investors can access their portfolios and make changes anytime, anywhere. |
User-friendly Interfaces | Robo-advisors provide intuitive platforms that are easy to navigate and understand. |
Consistency | Robo-advisors follow a disciplined approach to portfolio management, avoiding emotional biases. |
Swift Execution | Automated trading algorithms enable quick and efficient execution of trades. |
Advanced Risk Management | Robo-advisors utilize sophisticated risk assessment techniques to protect investments. |
In conclusion, Robo-advisors powered by AI and machine learning are revolutionizing portfolio management by offering cost-effective, data-driven, and accessible solutions. These platforms provide investors with personalized portfolios, continuous monitoring, and automated adjustments to maintain desired balances. The rise of Robo-advisors has democratized portfolio management, enabling a wider range of investors to access advanced investment strategies and achieve their financial goals.
AI in Portfolio Management: Addressing Risks
While the use of AI in portfolio management offers significant benefits, it also comes with its fair share of risks and challenges. One of the key challenges is the reliance on high-quality and complete data sets. AI models heavily depend on data to make accurate predictions and assessments. Incomplete or improper data sets can lead to biased models and inaccurate results.
The volume and complexity of data required for AI models can be another challenge. AI algorithms need vast amounts of data to learn and make informed decisions. Obtaining and managing such large datasets can be time-consuming and resource-intensive.
Moreover, there is always a risk of introducing biases into AI models. If the data sets used to train the models are biased or incomplete, the algorithm may learn and perpetuate those biases, leading to skewed predictions and decisions.
The Importance of Addressing Risks
Addressing these risks and challenges is crucial to ensure the success and effectiveness of AI in portfolio management. Mitigating biases, ensuring data integrity, and implementing robust data governance practices are essential steps to reduce risks associated with AI models. It is also important to constantly monitor and validate the performance of AI systems to identify and rectify any issues that may arise.
By addressing these risks, asset managers can harness the full potential of AI in portfolio management while minimizing potential pitfalls. The use of AI in portfolio management can lead to more informed investment decisions, enhanced risk management strategies, and ultimately, improved portfolio performance.
Risks | Challenges | Addressing Strategies |
---|---|---|
Reliance on high-quality data | Volume and complexity of data | Mitigating biases |
Managing incomplete data sets | Resource-intensive data management | Ensuring data integrity |
Biases introduced in AI models | Skewed predictions and decisions | Implementing robust data governance |
AI Portfolio Bot vs. Traditional Fund Manager: A Comparison
When it comes to managing portfolios, investors have two options: AI portfolio bots and traditional fund managers. Each approach has its own unique advantages and considerations. Let’s take a closer look at the benefits of AI in asset management and how it compares to traditional fund managers.
Benefits of AI in Asset Management:
- Objective and Data-Driven: AI portfolio bots base their decisions on advanced algorithms and data analysis, eliminating the influence of human emotions and biases.
- Consistency and Swift Execution: AI portfolios offer consistency in their approach, swiftly executing trades based on predetermined parameters.
- Advanced Risk Management Techniques: AI algorithms can analyze historical data patterns and identify risk factors, enabling efficient risk management strategies.
- Lower Management Fees: AI portfolio bots often come with lower management fees compared to traditional fund managers, providing cost-effective alternatives for investors.
“AI portfolio bots base their decisions on advanced algorithms and data analysis, eliminating the influence of human emotions and biases.”
On the other hand, traditional fund managers rely on their experience, expertise, and human judgment. While this approach can have its merits, it also introduces potential limitations. Traditional fund managers may be influenced by their emotions or biases and may not always have consistent decision-making processes. Additionally, their strategies may take longer to adapt to changing market conditions compared to AI portfolio bots.
Choosing the Right Approach:
The choice between an AI portfolio bot and a traditional fund manager ultimately depends on individual preferences, goals, and risk tolerance. Some investors may value the experience and personal touch of a traditional fund manager, while others may prefer the objectivity and data-driven nature of AI portfolios. It’s important to carefully evaluate the pros and cons of each approach and consider how well they align with your investment objectives.
Comparison | AI Portfolio Bot | Traditional Fund Manager |
---|---|---|
Decision-making process | Based on advanced algorithms and data analysis | Based on experience, expertise, and human judgment |
Execution speed | Swift execution of trades | May take longer to adapt to market conditions |
Risk management | Utilizes advanced risk management techniques | Risks may be managed based on individual judgment |
Management fees | Lower management fees compared to traditional fund managers | Management fees may vary |
Ultimately, the decision should be based on careful consideration of your specific needs and preferences. Evaluating the benefits and risks of AI portfolio bots and traditional fund managers can help you make an informed choice that aligns with your investment objectives.
Choosing the Right AI Portfolio: Key Considerations
When considering an AI portfolio for asset management, there are several key factors that investors should take into account. These factors can help ensure that the chosen AI portfolio aligns with their financial goals, risk tolerance, and investment preferences.
Transparency and Explainability
Transparency and explainability are crucial when selecting an AI portfolio. Investors should have a clear understanding of how the AI algorithms make investment decisions. It is important to choose AI portfolios that provide transparency in their decision-making process, allowing investors to have confidence in the underlying algorithms.
Ability to Learn and Adapt
The ability of an AI model to learn and adapt from new market data is vital for continuous improvement. Investors should consider AI portfolios that have a track record of learning from market trends and adapting their strategies accordingly. This ensures that the AI portfolio can stay relevant and effective in different market conditions.
Data Quality and Integrity
The quality and integrity of the data used in the AI model are critical factors to consider. Investors should assess the data sources and ensure that the data used is comprehensive, accurate, and unbiased. This helps in avoiding biased models and inaccurate predictions, leading to better investment decisions.
Track Record and Performance
Investors should evaluate the track record and performance of AI portfolios before making a decision. This includes examining historical performance, risk-adjusted returns, and consistency of results. Comparing the performance of different AI portfolios can help investors choose the one that aligns with their investment objectives.
By considering these key factors, investors can make informed decisions when selecting an AI portfolio for asset management. The right AI portfolio should provide transparency, adaptability, and utilize high-quality data to deliver optimal investment outcomes.
Factors to Consider | Affected Aspect |
---|---|
Transparency and Explainability | Investor Confidence |
Ability to Learn and Adapt | Relevance in Market Conditions |
Data Quality and Integrity | Accuracy of Predictions |
Track Record and Performance | Historical Results |
Portfolio Optimization and Machine Learning: ElectrifAi Perspective
In the world of asset management, portfolio optimization plays a critical role in delivering optimal returns and mitigating risks. At ElectrifAi, we believe that machine learning can revolutionize portfolio optimization by leveraging data-driven insights and advanced algorithms. By harnessing the power of machine learning, investors can enhance their portfolio management strategies and achieve better performance.
Machine learning algorithms have the ability to analyze complex data patterns and identify optimal trading strategies. With access to data from various sources including market trends, position information, risk exposure, and factor models, machine learning models can generate powerful insights for portfolio optimization. These insights enable investors to make informed decisions about their portfolios, taking into account risk and return calculations, and other relevant factors.
ElectrifAi’s PortfolioAi software is an industry-leading solution that utilizes machine learning for portfolio performance analysis. By evaluating the performance of portfolio managers based on risk, return calculations, and other metrics, PortfolioAi provides valuable insights for optimizing portfolios. With our expertise in machine learning and portfolio optimization, ElectrifAi empowers businesses with effective solutions to achieve optimal portfolio performance.
Advantages of ElectrifAi’s PortfolioAi Software |
---|
• Utilizes machine learning algorithms for data-driven portfolio optimization |
• Analyzes complex data patterns to identify optimal trading strategies |
• Evaluates portfolio managers’ performance based on risk and return calculations |
• Provides valuable insights for optimizing portfolios |
With ElectrifAi’s PortfolioAi software, investors can harness the power of machine learning to optimize their portfolios and achieve their financial objectives. Our data-driven approach and advanced algorithms ensure that portfolio optimization is based on accurate insights and robust analysis. By partnering with ElectrifAi, businesses can unlock the potential of machine learning in portfolio management and stay ahead of the competition.
Conclusion
AI has transformed the asset management industry, providing innovative solutions to optimize portfolio performance.
AI technology like machine learning can help investors improve fundamental analysis, manage risks, optimize portfolios, execute trades, and use robo-advisors for automated portfolio management.
Machine learning algorithms help investors gain insights from news and research papers by analyzing massive amounts of data. These insights improve data-driven investment decisions by identifying successful and underperforming investments.
AI helps analyze and mitigate risk by evaluating previous trade trends and discovering correlations. Additionally, AI-powered portfolio optimization optimizes market entrance and exit times to maximize returns and minimize losses.
Robo-advisors use AI and machine learning to create individualized portfolios based on investors’ risk tolerance and financial goals, revolutionizing portfolio management. Digital platforms automatically update portfolios to preserve balance when market conditions change.
AI has many benefits, but it requires high-quality data sets and may bias algorithms. Investors can maximize their portfolios and reach their financial goals by carefully examining these risks.
ElectrifAi, a prominent AI-powered solutions provider, offers portfolio optimization tools that analyze data trends, enhance trading methods, and improve risk management using machine learning algorithms. Businesses may optimize their portfolios and meet investment goals with their machine learning and portfolio optimization capabilities.
FAQ
How has AI revolutionized the asset management industry?
AI has revolutionized the asset management industry by providing solutions to optimize portfolio performance through machine learning algorithms and data analysis.
What areas of asset management can AI technologies be used in?
AI technologies, such as machine learning, can be used in various areas of asset management, including fundamental analysis, risk management, portfolio optimization, trade management, and robo-advising.
How can machine learning be leveraged in fundamental analysis?
Machine learning can be leveraged in fundamental analysis to analyze unstructured data sets and generate actionable insights, helping investors make more informed investment choices.
How can AI help in risk management?
AI can help in risk management by analyzing historical trade patterns, identifying correlations and patterns in data, and providing valuable insights for risk assessment and mitigation.
How does AI contribute to portfolio optimization?
AI contributes to portfolio optimization by helping investors optimize the timing of market entry and exit, identifying optimal portfolio compositions utilizing algorithms like Genetic algorithms, and enhancing investment strategies.
What advantages does algorithmic trading powered by AI offer in trade management?
Algorithmic trading powered by AI can reduce transaction costs, improve trade execution, and continuously learn and adapt from market data and changing conditions.
How are robo-advisors transforming portfolio management?
Robo-advisors, powered by AI and machine learning, assess investors’ risk tolerance and financial goals to construct personalized portfolios, continuously monitor market conditions, and provide cost-effective and data-driven portfolio management.
What are the risks and challenges of using AI in asset management?
The risks and challenges of using AI in asset management include the need for quality and ample data sets, the risk of biased models, and the potential for technology breaches.
What are the advantages of AI portfolios compared to traditional fund managers?
AI portfolios offer objective and data-driven investment choices, consistency, swift trade execution, advanced risk management, and lower management fees compared to traditional fund managers.
What should investors consider when choosing an AI portfolio?
Investors should consider the transparency and explainability of AI algorithms, the ability of the AI model to learn and adapt, the quality and integrity of data used, and the track record and performance of AI portfolios based on their individual preferences, goals, and risk tolerance.
How can ElectrifAi’s machine learning expertise help optimize portfolios?
ElectrifAi’s machine learning expertise can analyze data patterns, optimize trading strategies, predict stock returns, improve risk management, and offer powerful portfolio performance analysis to help evaluate portfolio managers’ performance based on risk, return calculations, and other factors.
Source Links
- https://insights.daffodilsw.com/blog/the-role-of-ai-in-intelligent-portfolio-management
- https://www.zbw.eu/econis-archiv/bitstream/11159/421820/1/EBP07631488X_0.pdf
- https://medium.com/geekculture/portfolio-optimization-with-machine-learning-adc279daaa82