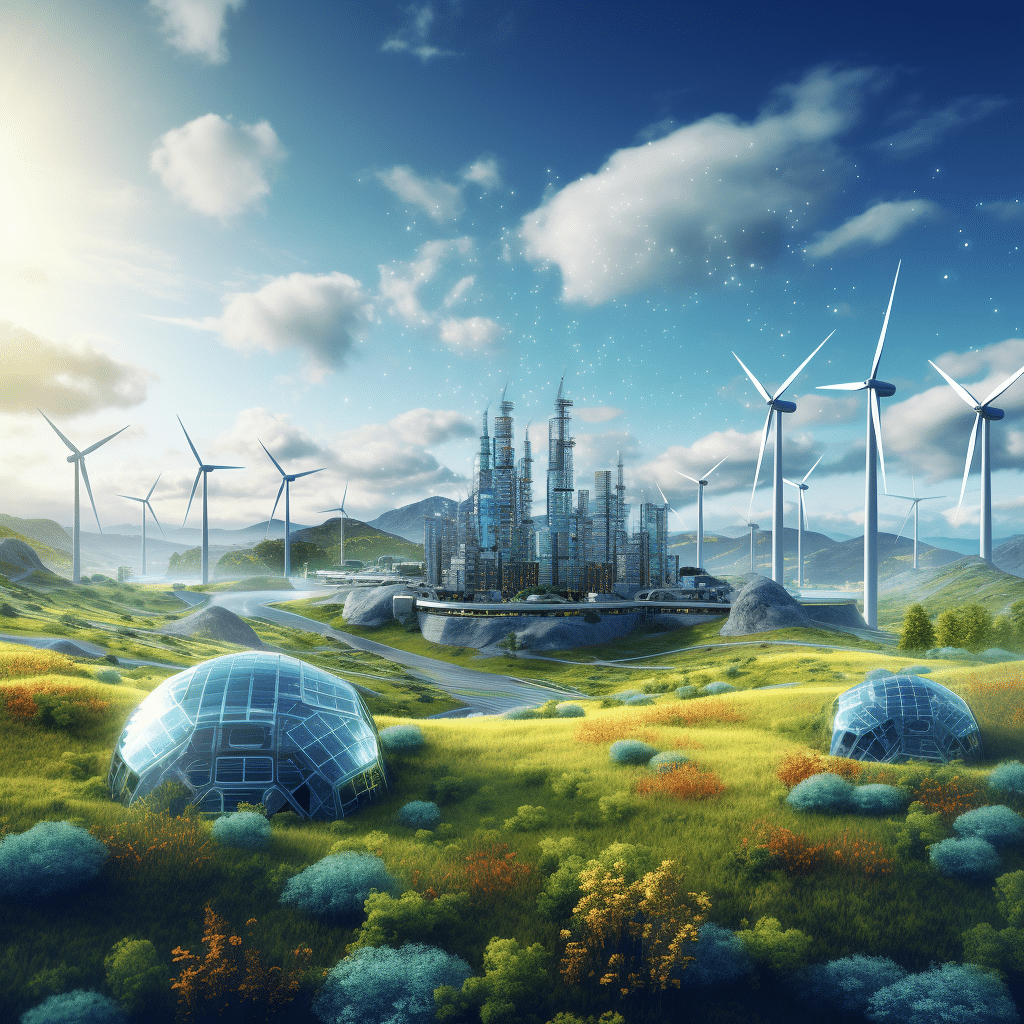
How AI is Shaping the Future of Renewable Energy
Renewable energy’s sources like solar, wind, and hydroelectric power have been gaining traction as the world shifts towards more sustainable alternatives. However, optimizing their efficiency and integration into existing power grids remains a challenge. This is where Artificial Intelligence (AI) steps in, revolutionizing the way we harness and utilize renewable energy resources.
AI algorithms play a crucial role in forecasting renewable energy’s generation based on weather patterns, historical data, and power demand. By analyzing vast amounts of information, AI can accurately predict the optimal times for energy generation and consumption, minimizing waste and reducing costs.
Future of Renewable Energy
Additionally, AI-driven systems can manage and streamline renewable energy’s grids, balancing supply and demand to ensure stability. Smart grids powered by AI are capable of autonomously adjusting energy distribution, reducing load imbalances, and even predicting failures or potential issues before they occur.
One exciting area where AI is making significant contributions is in solar panel technology. Deep learning algorithms can analyze real-time data from solar panels to identify any anomalies or malfunctions, enabling proactive maintenance and maximizing energy production.
“AI algorithms are driving efficiency and optimization in renewable energy production, paving the way for a sustainable future.”
Wind power is another beneficiary of AI advancements. Machine learning algorithms can determine optimal wind turbine placements based on wind speeds, topography, and other environmental factors. This enables more efficient energy production from wind farms, reducing costs and increasing output.
Furthermore, AI-powered energy management systems are empowering consumers to actively participate in the renewable energy’s transition. With intelligent systems monitoring consumption patterns, users can adjust their energy usage according to available renewable energy sources and take advantage of lower-priced electricity during periods of high production.
The future looks promising for the integration of AI and renewable energy’s. As AI algorithms continue to improve and evolve, the efficiency and efficacy of renewable energy generation will increase exponentially. With ongoing research and technological advancements in this field, we can expect a greener, more sustainable future.
In what ways is artificial intelligence being used to optimize the efficiency of renewable energy sources?
Artificial intelligence (AI) is being utilized in various ways to optimize the efficiency of renewable energy sources. Some of these ways include:
1. Predictive Analytics:
AI algorithms can analyze historical and real-time data from renewable energy sources such as solar panels or wind turbines to predict future energy production. This helps in balancing the supply and demand of electricity, optimizing storage and grid management, and reducing waste.
2. Energy Demand Forecasting:
AI models can analyze large datasets to forecast energy consumption patterns and predict peak demand periods. By anticipating demand fluctuations, renewable energy sources can be better managed, ensuring optimal utilization and reducing the need for fossil fuel-based backup power.
3. Smart Grid Optimization:
AI algorithms enable efficient management and control of electricity grids by integrating renewable energy sources with the existing infrastructure. Grid operators can dynamically balance supply and demand, enhance grid stability, and optimize energy distribution and storage using AI-driven decision-making systems.
4. Fault Detection and Maintenance:
AI can identify and detect faults or anomalies in renewable energy systems in real-time, enabling proactive maintenance and reducing downtime. AI-based monitoring systems can predict equipment failures, optimize performance, and schedule repairs, ensuring smooth operation of renewable energy infrastructure.
5. Energy Storage Optimization:
AI systems can analyze energy storage levels, demand patterns, and generation capacity to optimize the charging and discharging cycles of energy storage systems. This prevents overcharging or underutilization of batteries, improving their efficiency and prolonging their lifespan.
6. Renewable Resource Mapping:
AI technologies, such as machine learning and satellite imagery, can analyze geographical data to accurately map potential renewable energy resources, such as solar or wind energy. This helps in identifying suitable locations for installing renewable energy systems and maximizing their output.
7. Energy Trading and Pricing:
AI algorithms can help power markets find the best way to trade and price renewable energy. They can look at market data, consumption patterns, and weather forecasts to figure out the most cost-effective ways to set prices and make it easier for green energy producers and consumers to trade energy in a way that benefits both parties.
By using AI, renewable energy sources can be added to current energy systems in a more effective way. This leads to more efficiency, lower costs, and a smoother transition to a sustainable energy future.
What are some examples of successful AI applications in the integration of renewable energy’s into smart grids?
There are several examples of successful AI applications in the integration of renewable energy’s into smart grids. Some of them include:
1. Predictive Modeling and Forecasting: AI algorithms examine past weather, load, and renewable energy patterns to anticipate future energy generation and consumption. This data helps grid managers maximize renewable energy’s consumption and balance supply and demand.
2. AI-powered Energy Management Systems optimize energy distribution by analyzing real-time data from smart meters, weather sensors, and renewable energy sources. The systems can efficiently manage energy storage charging and discharging, allocating resources based on demand and availability.
3. Grid Stability and Flexibility: AI algorithms can aid grid stability and intermittent renewable energy’s management. AI monitors and analyzes real-time grid data to forecast and address disturbances, fluctuations, and imbalances, guaranteeing a stable electricity supply.
4. Demand Response and Load Management: AI can aid utilities by studying consumer behavior data and predicting demand patterns. Using this data, utilities can encourage consumers to consume electricity at peak renewable energy availability, reducing peak demand and improving grid efficiency.
5. Detection and Diagnosis: AI algorithms evaluate data from smart sensors in grid infrastructure to identify flaws, anomalies, and probable equipment breakdowns. Early detection of faults by AI improves grid resilience by reducing downtime and enabling proactive maintenance.
These applications demonstrate how AI can play a crucial role in integrating renewable energy into smart grids by optimizing energy management, ensuring grid stability, enabling demand response, and improving overall system efficiency.
How can AI algorithms assist in forecasting renewable energy production and demand?
AI algorithms can assist in forecasting renewable energy production and demand in several ways:
1. Data analysis: AI algorithms can analyze historical data on renewable energy production, weather patterns, and energy consumption to identify patterns and trends. This analysis can help in predicting future production and demand levels.
2. Weather prediction: AI algorithms can analyze weather data and models to forecast solar irradiation, wind speed, and other factors that impact renewable energy production. This information can be used to estimate the potential energy output from solar and wind farms.
3. Demand prediction: AI algorithms can analyze historical energy consumption data, as well as socio-economic and demographic factors, to predict future energy demand. This information can be useful for planning and optimizing renewable energy generation and distribution systems.
4. Optimization and scheduling: AI algorithms can optimize the scheduling and dispatch of renewable energy sources based on real-time data, market prices, and demand forecasts. This can ensure efficient utilization of renewable energy resources and minimize costs.
5. Grid management: AI algorithms can assist in managing the integration of renewable energy into the power grid. They can predict fluctuations in energy supply and demand and help balance the grid by coordinating renewable energy generation, storage, and consumption.
6. Maintenance and performance monitoring: AI algorithms can analyze sensor data from renewable energy systems to detect anomalies, predict equipment failure, and optimize maintenance schedules. This can improve the reliability and performance of renewable energy infrastructure.
Overall, AI algorithms can provide valuable insights and automate complex analysis tasks, ultimately improving the accuracy and efficiency of forecasting renewable energy production and demand.
How does the implementation of AI in renewable energy contribute to overcoming the challenges of scalability and intermittency
Artificial Intelligence (AI) has the potential to significantly address the challenges of scalability and intermittency in renewable energy’s systems. Here are a few ways in which the implementation of AI contributes to overcoming these challenges:
1. Predictive Analytics:
Using data from renewable energy’s sources, weather patterns, historical data, and grid demand, AI can estimate future energy generation and consumption. Using AI to estimate renewable energy’s output and demand can assist grid operators optimize distribution and balance supply and demand, reducing intermittency.
2. Demand Response:
AI can optimize energy consumption by predicting peak demand periods and adjusting energy consumption in response. This can be done by utilizing machine learning algorithms to analyze historical data and patterns in demand. By implementing demand response mechanisms, the grid can reduce peak demand, balance loads, and compensate for renewable energy’s intermittency.
3. Energy Storage Optimization:
AI can determine when to charge and discharge energy storage systems, such as batteries, based on renewable energy supply and grid demand. Predictive algorithms can enhance energy storage efficiency and store extra renewable energy’s during high-generation periods for later usage during low-generation periods.
4. Grid Management and Control:
AI algorithms examine real-time data from renewable energy’s sources, grid dynamics, and energy storage devices to improve grid management and control. AI enables real-time energy flow adjustments, generation capacity optimization, and demand balance, reducing renewable energy intermittency and improving system scalability.
5. Fault Detection and Maintenance:
By analysing sensor data, historical data, and patterns, AI can find renewable energy’s system defects and estimate maintenance needs. Using machine learning, AI can detect equipment breakdown early, improving system reliability and reducing downtime for renewable energy systems.
AI can optimize energy generation, estimate demand, improve storage efficiency, grid management, and proactive maintenance in renewable energy to handle scalability and intermittency. Renewable energies are becoming steady and viable alternatives to fossil fuels as technology advances.
Your article helped me a lot, is there any more related content? Thanks!
I don’t think the title of your article matches the content lol. Just kidding, mainly because I had some doubts after reading the article.
Your article helped me a lot, is there any more related content? Thanks!
Rattling excellent info can be found on web site.Raise blog range