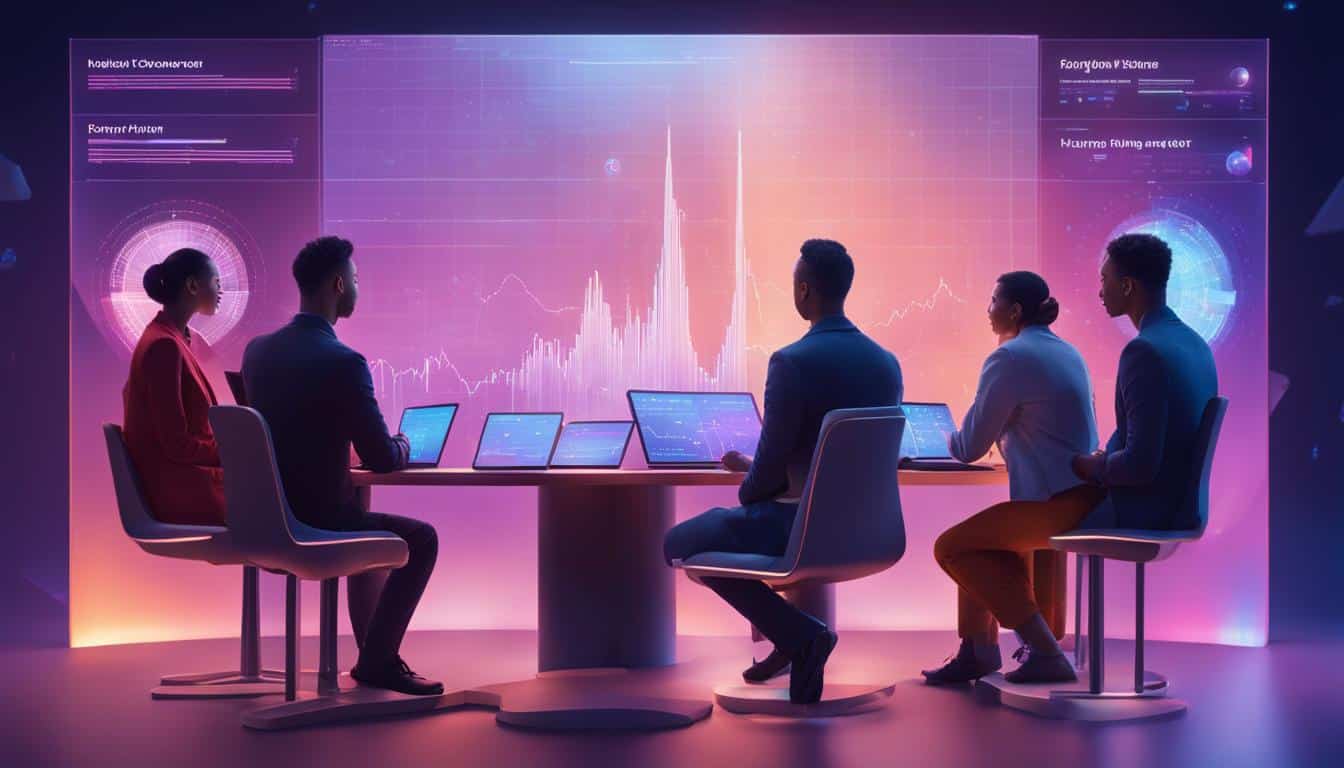
In the ever-evolving landscape of the US credit industry, a groundbreaking revolution is taking place. The advent of AI-driven credit scoring is transforming the way lenders assess creditworthiness and make lending decisions. This innovative approach optimizes risk management and decision-making, allowing lenders to extend financial services to a larger population.
Traditional credit scoring models have limitations when it comes to assessing the creditworthiness of individuals with limited credit history or unconventional financial behavior. However, AI-based credit scoring harnesses the power of machine learning algorithms to evaluate a borrower’s creditworthiness using a wide range of data points, including non-traditional sources. This enables a more accurate and individualized assessment, opening doors to financial services for more people.
By leveraging AI technology, lenders can now consider factors such as total income, credit history, transaction analysis, work experience, and user behavior analytics to determine an applicant’s creditworthiness. This personalized approach enables lenders to make more informed lending decisions, reducing the risk of defaults and improving overall risk management.
Key Takeaways:
- AI-driven credit scoring revolutionizes the lending industry.
- It optimizes risk management and decision-making.
- AI scoring allows more people to access financial services.
- Machine learning algorithms evaluate creditworthiness based on various data points.
- Individualized assessments lead to more informed lending decisions.
Understanding AI Credit Score and Its Meaning
Artificial Intelligence (AI) credit scoring is a cutting-edge approach to evaluating a borrower’s creditworthiness. By utilizing machine learning algorithms and analyzing a wide range of data points, AI credit scoring provides a more comprehensive and dynamic assessment of credit risk. This enables lenders to make more accurate decisions based on a borrower’s financial behavior.
An AI credit score takes into account various factors, including traditional credit history, total income, transaction analysis, work experience, and even user behavior analytics. By considering non-traditional data sources, AI credit scoring provides a more personalized and robust measure of creditworthiness. It goes beyond the limitations of traditional scoring models, offering lenders a deeper understanding of a borrower’s ability to repay loans.
This innovative approach to credit scoring has significant implications for both lenders and borrowers. For lenders, AI credit scoring allows for better risk management, as it can predict the likelihood of loan repayment more accurately. This helps lenders make smarter lending decisions and optimize their lending portfolios. For borrowers, AI credit scoring offers a fairer and more inclusive credit assessment, enabling access to financial services for those who may not have sufficient traditional credit history.
How AI Credit Score Predicts Loan Repayment
AI credit scoring models analyze a vast amount of historical data to identify patterns and factors that correlate with loan repayment behavior. By training on historical loan data, these models learn to recognize specific patterns associated with borrowers who have successfully repaid their loans in the past. The AI algorithms then apply this learning to predict the likelihood of loan repayment for future borrowers.
“AI credit scoring provides a more comprehensive and dynamic assessment of credit risk, enabling lenders to make more accurate decisions based on a borrower’s financial behavior.”
By taking into account a wide range of variables and analyzing their interdependencies, AI credit scoring models can identify nuanced patterns and trends that may not be evident to human analysts. This enables lenders to make more informed decisions and better allocate their resources. The predictive power of AI credit scoring helps lenders optimize their risk management strategies and offer more tailored and competitive loan products to borrowers.
Traditional Credit Scoring | AI Credit Scoring |
---|---|
Relies on limited traditional data sources | Considers a wide range of data, including non-traditional sources |
May have a narrow scope in assessing creditworthiness | Provides a more comprehensive and personalized credit assessment |
Less accurate in predicting loan repayment | Utilizes advanced algorithms to predict loan repayment with higher accuracy |
May exclude certain populations due to lack of credit history | Enables access to financial services for underserved populations |
The Role of Artificial Intelligence in Credit Scoring
Artificial Intelligence (AI) plays a transformative role in credit scoring by revolutionizing the way lenders assess borrowers’ creditworthiness. With its ability to process vast amounts of data, identify patterns, and make accurate predictions, AI has the power to provide a more personalized and fair assessment of creditworthiness.
Unlike traditional credit scoring methods that rely heavily on historical data and credit scores, AI-based credit scoring algorithms consider a wider range of factors. These factors may include non-traditional data sources such as transaction analysis, work experience, and user behavior analytics. By taking into account alternative data, AI scoring models can extend credit opportunities to underserved populations who may not have sufficient credit history.
One of the key benefits of AI credit scoring is its ability to provide a more individualized assessment. By analyzing a borrower’s financial behavior, AI algorithms can generate a credit score that reflects their unique creditworthiness. This personalized approach allows lenders to make more informed decisions and tailor their loan offerings to meet the specific needs of borrowers.
AI credit scoring brings a level of fairness to the lending industry by reducing bias and discrimination in the credit assessment process. With AI, lenders can make credit decisions based on objective data and algorithms, eliminating subjective judgments and human biases that may impact traditional credit scoring methods.
The table below illustrates how AI credit scoring provides a transformative role in credit assessment:
Traditional Credit Scoring | AI Credit Scoring |
---|---|
Relies heavily on credit scores and historical data | Considers a wider range of factors, including alternative data |
Limited to borrowers with established credit history | Extends credit opportunities to underserved populations |
Provides a generalized assessment of creditworthiness | Offers a more personalized and individualized credit assessment |
Susceptible to human biases and subjective judgments | Reduces bias and discrimination in the credit assessment process |
Overall, AI credit scoring has the potential to revolutionize the lending industry by providing lenders with a more accurate and fair assessment of borrower creditworthiness. By leveraging the power of artificial intelligence, lenders can make smarter lending decisions and extend credit opportunities to a broader range of individuals.
How AI-based Credit Scoring with Datrics is Different from Traditional Models
AI-based credit scoring models built with Datrics offer significant advantages over traditional scorecard approaches. By leveraging artificial intelligence and real-time indicators, these models provide more precise profit predictions and include creditworthy borrowers who may not have sufficient historical data. Let’s explore how AI-based credit scoring with Datrics sets itself apart from traditional models:
Real-time Indicators
Datrics utilizes real-time indicators, such as current income, employment opportunities, and potential earning ability, to assess a borrower’s creditworthiness. Traditional models often rely heavily on historical data, which can limit their ability to capture the current financial situation of borrowers. With real-time indicators, AI-based credit scoring models can provide more up-to-date and accurate assessments.
Precise Profit Predictions
The integration of AI algorithms in credit scoring models allows for more precise profit predictions. Datrics’ models consider a wide range of factors and analyze complex patterns to generate accurate predictions of loan repayment and profitability. This level of precision empowers lenders to make informed decisions and optimize their risk management strategies.
Inclusion of Creditworthy Borrowers
Traditional credit scoring models often have strict requirements and rely heavily on historical data, which can exclude creditworthy individuals who lack an extensive credit history. AI-based credit scoring with Datrics takes a more holistic approach by considering alternative data and real-time indicators. This enables the inclusion of individuals who may have limited credit history but demonstrate the potential to be creditworthy borrowers.
AI-based Credit Scoring with Datrics | Traditional Models |
---|---|
Utilizes real-time indicators | Relies heavily on historical data |
Provides precise profit predictions | Less accurate profit predictions |
Includes creditworthy borrowers with limited credit history | Excludes creditworthy individuals with limited credit history |
How Datrics Solves the Challenges of AI Credit Scoring
Datrics addresses several key challenges in AI credit scoring to ensure transparency, mitigate bias, and provide interpretable models. The following are the main challenges and how Datrics tackles them:
Challenge 1: Transparency
One of the major concerns with AI credit scoring is the lack of transparency in the decision-making process. Datrics addresses this challenge by providing tools that offer transparency and explainability. Their platform allows analysts to interpret credit default risk predictions and visualize the impact of different features on predictions. This not only helps lenders understand the factors influencing the credit decision but also improves trust in the AI scoring system.
Challenge 2: Bias
Bias in credit scoring can lead to unfair treatment of certain individuals or groups, resulting in potential discrimination. Datrics combats this challenge by offering a stratified approach to modeling and manual weighting. By considering different groups separately and assigning appropriate weights, they reduce the risk of biased decisions that unfairly favor or disadvantage specific cohorts. This approach promotes equal opportunity for all potential borrowers, ensuring a fair credit assessment process.
Challenge 3: Interpretation
Interpreting the predictions and recommendations of AI credit scoring models can be difficult for analysts. Datrics addresses this challenge by providing tools and visualizations that help interpret the credit scoring models. Analysts can gain insights into the factors contributing to credit assessments and make informed decisions based on the model’s predictions. This level of interpretation enables lenders to have a clear understanding of the creditworthiness of borrowers and reduces the reliance on black box models.
Challenge 4: Black Box Problem
The “black box” problem refers to the difficulty in understanding and explaining the inner workings of AI models. Datrics tackles this challenge by ensuring that the factors influencing credit decisions are interpretable. Analysts can explore and analyze the impact of different features on predictions, making the credit scoring process more transparent and understandable. This also helps in complying with regulatory requirements, where model interpretability is often mandated.
By addressing these challenges, Datrics enhances the reliability and effectiveness of AI credit scoring models, making them more trustworthy and suitable for the lending industry.
Integrating ML with Traditional Scoring for Comprehensive Analysis
When it comes to credit scoring, a hybrid approach that combines machine learning (ML) with traditional scoring methods offers a comprehensive and transparent analysis. This approach allows lenders to leverage the benefits of ML algorithms while maintaining the clarity and familiarity of traditional scorecards.
By integrating ML with traditional scoring, lenders can tap into the power of advanced algorithms to process large amounts of data and make more informed credit decisions. ML models can analyze a wide range of factors beyond traditional data points, such as current income, employment opportunities, and potential earning ability. This provides a more holistic view of a borrower’s creditworthiness.
Benefits of Integrating ML with Traditional Scoring
By embracing this hybrid approach, lenders can unlock several benefits:
- Improved Accuracy: ML algorithms can identify patterns and correlations in data that may go unnoticed by traditional scoring methods. This leads to more accurate credit assessments and reduces the risk of default.
- Enhanced Risk Management: ML models can assess credit risk in real-time, allowing lenders to adapt their lending strategies and mitigate potential losses.
- Increased Efficiency: By automating the credit analysis process, ML models can process large volumes of applications quickly and efficiently, allowing lenders to make faster lending decisions.
- Transparent Credit Analysis: While ML algorithms can be complex, the integration with traditional scoring methods provides transparency in the decision-making process. Lenders can understand the factors influencing credit decisions and ensure fairness in their lending practices.
In summary, the integration of ML with traditional scoring methods revolutionizes credit analysis by enabling lenders to make smarter lending decisions. It combines the power of advanced algorithms with the transparency and familiarity of traditional scorecards. This comprehensive approach enhances risk management, improves efficiency, and ensures fair and transparent credit assessments. By leveraging the benefits of ML in lending, lenders can optimize their lending processes and provide better financial outcomes for both lenders and borrowers.
Benefits of Integrating ML with Traditional Scoring |
---|
Improved Accuracy |
Enhanced Risk Management |
Increased Efficiency |
Transparent Credit Analysis |
Addressing Decision Bias
When it comes to credit scoring, decision bias can lead to unfair favoritism or disadvantage for certain groups or cohorts. At Datrics, we understand the importance of addressing this bias and ensuring a fair and unbiased credit assessment process.(Decision Bias, Unfair Favoritism, Disadvantage)
We offer a stratified approach to modeling and manual weighting to minimize the risk of biased decisions. By stratifying data and considering relevant factors, we aim to provide a more accurate and comprehensive credit assessment for all potential borrowers. This approach helps to reduce the chances of unfair treatment based on factors such as race, gender, or socioeconomic status.(Stratified Approach, Manual Weighting)
The Importance of Transparency
Transparency is another key aspect of addressing decision bias in credit scoring. At Datrics, we provide tools and insights that allow analysts to interpret credit default risk predictions and visualize the impact of various features on credit assessments. This transparency helps to highlight any potential biases and ensures that decisions can be thoroughly evaluated and understood.(Decision Bias, Transparency, Interpretation)
“By offering transparency and explainability in the decision-making process, we aim to tackle the black box problem often associated with AI credit scoring models.”
Striving for Fair and Unbiased Assessments
Our goal is to promote equal opportunity for all potential borrowers by providing fair and unbiased credit assessments. We believe that by addressing decision bias and promoting transparency, we can contribute to a more inclusive lending industry and help individuals access the financial services they need.(Decision Bias, Fair and Unbiased Assessments)
By leveraging the power of AI and combining it with a thoughtful and stratified approach, we can create credit scoring models that are more reliable, accurate, and fair. At Datrics, we are committed to continuously improving and refining our credit scoring solutions to ensure that they meet the highest standards of integrity and fairness.
Benefits | Features |
---|---|
Addressing Decision Bias | A stratified approach to modeling and manual weighting reduces the risk of biased decisions. |
Transparency and Explainability | Datrics provides tools for interpreting credit default risk predictions and visualizing the impact of features on credit assessments. |
Promoting Equal Opportunity | Fair and unbiased credit assessments help promote equal opportunity for all potential borrowers. |
Credit Scoring Process
In the credit scoring process with Datrics, we follow a comprehensive approach to assess the creditworthiness of borrowers. Our solution utilizes cutting-edge AI technology and machine learning algorithms to analyze various data points and provide accurate credit assessments. Here is an overview of the steps involved in our credit scoring process:
Data Collection and Analysis
First, we collect relevant data from borrowers, including financial information, credit history, employment details, and other factors. Our AI algorithms then analyze this data to identify patterns and trends that can help determine creditworthiness. By considering both traditional and alternative data sources, we provide a more holistic view of a borrower’s financial situation.
Credit Score Model Development
Once the data analysis is complete, we develop individual credit score models tailored to the specific needs of our clients. These models incorporate the insights gained from data analysis and are designed to accurately predict the likelihood of loan repayment. Our team of experts works closely with clients to ensure that the credit scoring models align with their risk management strategies and business objectives.
Deployment and Maintenance
After the credit scoring models are developed, we assist our clients in deploying them within their existing infrastructure. This ensures data privacy and security while allowing seamless integration with their lending processes. We also provide ongoing maintenance and support to ensure that the credit scoring models remain up-to-date and accurate over time.
Overall, our credit scoring process with Datrics enables lenders to make more informed and reliable lending decisions, leading to enhanced risk management and optimized loan portfolios. By leveraging the power of AI and machine learning, we aim to revolutionize the credit industry and extend access to financial services for a wider population.
Data Collection and Analysis | Credit Score Model Development | Deployment and Maintenance |
---|---|---|
Collect borrower data from various sources | Develop tailored credit score models | Assist in deploying models within client infrastructure |
Analyze data to identify creditworthiness indicators | Incorporate insights from data analysis | Provide ongoing maintenance and support |
Consider traditional and alternative data sources | Align models with client risk management strategies | Ensure data privacy and security |
Benefits of AI Credit Scoring with Datrics
AI credit scoring with Datrics offers numerous benefits that revolutionize the lending industry, resulting in faster lending decisions, smarter analysis of data for credit assessments, and enhanced risk management. By leveraging AI technology, lenders can streamline their processes and improve their overall efficiency, ultimately benefiting both lenders and borrowers.
One of the key advantages of AI credit scoring is the speed at which lending decisions can be made. With AI-powered algorithms, lenders can process large amounts of data quickly and accurately assess a borrower’s creditworthiness. This enables lenders to respond to loan applications in a timely manner, providing borrowers with faster access to the funds they need.
In addition to speed, AI credit scoring allows for smarter lending decisions. By analyzing a wide range of data points and considering non-traditional sources of information, AI models can provide lenders with a more comprehensive and dynamic assessment of credit risk. This enables lenders to make more informed decisions based on a borrower’s financial behavior and overall creditworthiness.
“AI credit scoring with Datrics enables lenders to process large amounts of data quickly and make more informed decisions, resulting in better financial outcomes for both lenders and borrowers.”
Moreover, AI credit scoring with Datrics enhances risk management for lenders. By uncovering patterns and trends in borrower data, AI models can help lenders identify potential risks and make proactive decisions to mitigate them. This reduces the likelihood of defaults and improves the overall portfolio performance for lenders.
Table: Benefits of AI Credit Scoring with Datrics
Benefits | Description |
---|---|
Speed | AI-powered algorithms enable faster lending decisions, reducing waiting time for borrowers. |
Smarter Lending Decisions | AI models consider a wide range of data points, resulting in more comprehensive credit risk assessments. |
Enhanced Risk Management | AI credit scoring helps lenders identify and mitigate potential risks, improving portfolio performance. |
Conclusion
AI credit scoring is revolutionizing the lending industry, bringing about a new era of automated credit assessment and machine learning in lending. This technology has the potential to optimize risk management and decision-making processes, leading to a significant revolution in the way loans are issued.
With AI credit scoring, lenders can make more accurate and personalized credit assessments, taking into account a borrower’s individual circumstances and financial behavior. By leveraging machine learning algorithms, this technology can process large amounts of data quickly, enabling lenders to make smarter lending decisions in a fraction of the time.
Furthermore, AI credit scoring offers enhanced risk management capabilities, allowing lenders to better assess creditworthiness and minimize the likelihood of default. By integrating machine learning with traditional scoring methods, this approach provides a transparent and comprehensive analysis of a borrower’s creditworthiness, ensuring a more efficient lending process.
In conclusion, the advent of AI credit scoring represents a remarkable revolution in lending. By automating and optimizing the credit assessment process, this technology has the potential to expand access to financial services, promote fair and unbiased lending practices, and drive better financial outcomes for both lenders and borrowers.
FAQ
How does AI credit scoring evaluate a borrower’s creditworthiness?
AI credit scoring evaluates a borrower’s creditworthiness based on factors such as total income, credit history, transaction analysis, work experience, and user behavior analytics.
What is the role of AI in credit scoring?
AI plays a transformative role in credit scoring by processing large amounts of data, identifying patterns, and making accurate predictions. It enables a more personalized and fair assessment of creditworthiness by considering alternative data and extending credit opportunities to underserved populations.
How is AI-based credit scoring with Datrics different from traditional models?
AI-based credit scoring models built with Datrics are more sensitive to real-time indicators of a borrower’s creditworthiness and consider factors such as current income, employment opportunities, and potential earning ability. This allows for more precise profit predictions and inclusion of creditworthy borrowers who may not have sufficient historical data.
How does Datrics tackle the challenges of AI credit scoring?
Datrics provides transparency and explainability in the decision-making process by offering tools for interpreting credit default risk predictions and visualizing the impact of features on predictions. They also address the black box problem by ensuring analysts can understand the factors influencing decisions.
How does Datrics integrate machine learning with traditional scoring methods?
Datrics integrates machine learning with traditional scoring methods to provide a comprehensive and transparent credit analysis. This hybrid approach leverages the benefits of machine learning while maintaining clarity and familiarity. Their model score distribution plot visualizes the distribution of output scores and the transformation of the credit scoring model to traditional scorecards.
How does Datrics address decision bias in credit scoring?
Datrics offers a stratified approach to modeling and manual weighting, reducing the risk of biased decisions that unfairly favor or disadvantage certain groups or cohorts. They promote equal opportunity for all potential borrowers by ensuring a fair and unbiased credit assessment.
What does the credit scoring process with Datrics involve?
The credit scoring process with Datrics involves developing individual credit score models and providing clients with business expertise. Their solution is usually run within the client’s infrastructure, ensuring data privacy and security. They also offer support for deployment and maintenance of the credit scoring models.
What are the benefits of AI credit scoring with Datrics?
AI credit scoring with Datrics offers benefits such as speed in making lending decisions, smarter analysis of data for credit assessments, and improved risk management. Their models allow lenders to process large amounts of data quickly and make more informed decisions, resulting in better financial outcomes for both lenders and borrowers.
How does AI credit scoring revolutionize the lending industry?
AI credit scoring revolutionizes the lending industry by optimizing risk management and decision-making. It enables lenders to make more accurate and personalized credit assessments, extending credit opportunities to underserved populations. With the integration of machine learning and traditional scoring methods, AI credit scoring with Datrics offers transparent and comprehensive analysis for a more efficient lending process.
Source Links
- https://www.datrics.ai/articles/the-essentials-of-ai-based-credit-scoring
- https://blog.aspiresys.com/data-and-analytics/how-ai-in-banking-is-revolutionizing-credit-scoring/
- https://attentioninsight.com/the-use-of-ai-and-machine-learning-in-lending/